Analyzing Categorical Data Relationships Using Chi-Square Goodness of Fit and Independence Tests
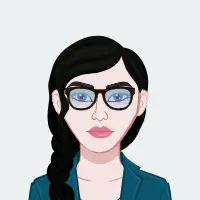
Statistics is a critical field of study that involves collecting, analyzing, and interpreting data to make informed decisions. One of the essential tools for analyzing categorical data is the Chi-Square test. This blog will delve into two significant types of Chi-Square tests: the Chi-Square Goodness of Fit Test and the Chi-Square Test for Independence. These tests help determine whether observed data match expected distributions or if there is an association between categorical variables. By understanding and applying these tests, you can effectively solve your statistics assignment and gain valuable insights from your data. Whether you're a student working on an assignment or a researcher analyzing survey data, mastering these techniques is crucial for accurate data analysis and interpretation. If you're seeking help with data analysis assignments, this guide will provide a step-by-step approach, practical tips, and real-world examples to help you confidently apply Chi-Square tests to your statistics assignments."
Understanding Chi-Square Tests
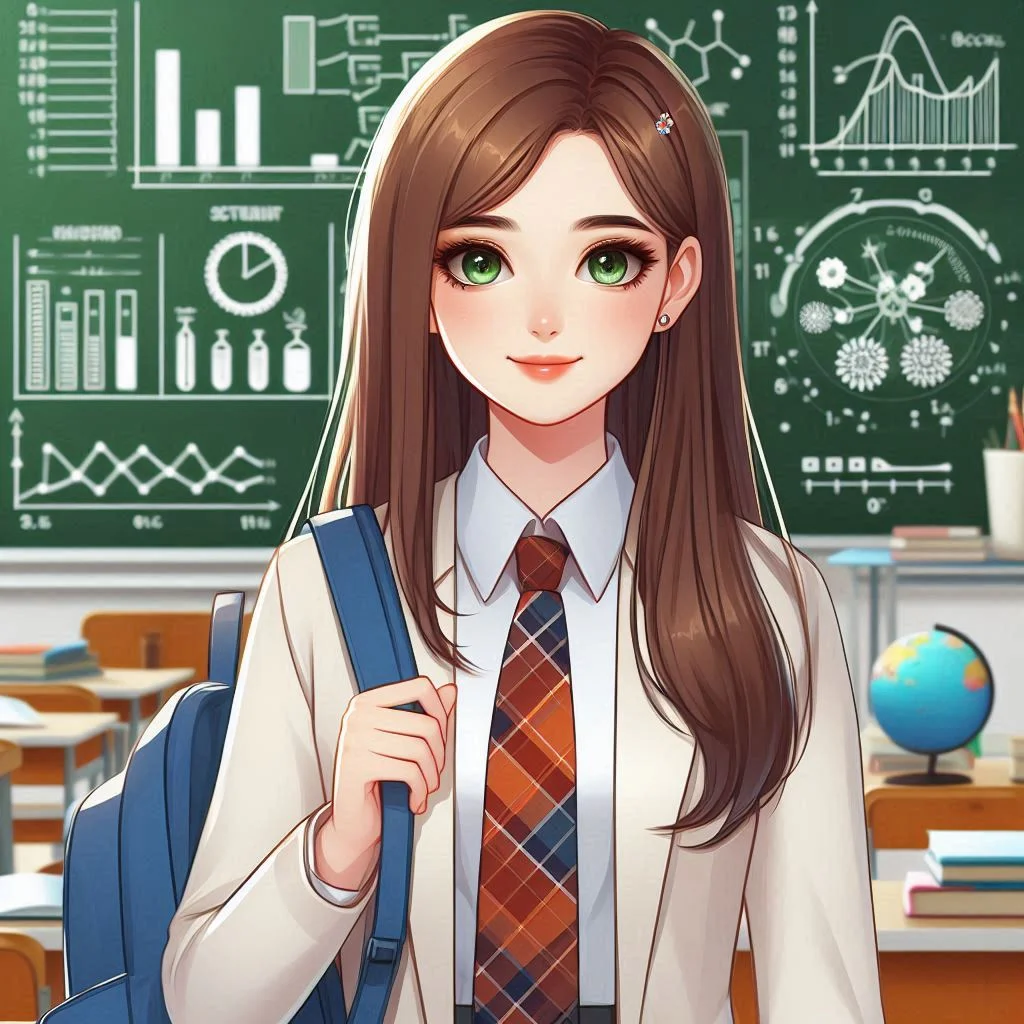
Chi-Square tests are vital for analyzing categorical data. They assess how well observed data fit expected distributions or reveal associations between variables. By comparing observed and expected frequencies, these tests determine if there are significant deviations or relationships. Mastery of Chi-Square tests enhances your ability to draw meaningful conclusions from categorical data.
A. Chi-Square Goodness of Fit Test
The Chi-Square Goodness of Fit Test is used to determine whether the observed frequency distribution of a categorical variable matches an expected distribution. This test is particularly useful when you want to see if your data fit a theoretical distribution or a distribution from previous research.
Steps to Conduct a Chi-Square Goodness of Fit Test:
1. State the Hypotheses:
- Null Hypothesis (H_0): The observed frequencies fit the expected distribution.
- Alternative Hypothesis (H_1): The observed frequencies do not fit the expected distribution.
2. Calculate the Expected Frequencies:
- Determine the expected frequency for each category based on the theoretical distribution.
3. Compute the Chi-Square Statistic:
- Use the formula: χ2=∑[(Oi−Ei)^2]/Ei
- Where O_i is the observed frequency and E_i is the expected frequency.
4. Determine the Degrees of Freedom:
- Degrees of freedom (df) is calculated as the number of categories minus one (df=k−1).
5. Compared to the Critical Value:
- Compare the computed Chi-Square statistic to the critical value from the Chi-Square distribution table based on the degrees of freedom and the significance level (α).
6. Make a Decision:
- If the Chi-Square statistic is greater than the critical value, reject the null hypothesis.
B. Chi-Square Test for Independence
The Chi-Square Test for Independence is particularly useful in contingency tables where you want to see if the variables are independent.
Steps to Conduct a Chi-Square Test for Independence:
1. State the Hypotheses:
- Null Hypothesis (H_0): The two variables are independent.
- Alternative Hypothesis (H_1): The two variables are not independent.
2. Create a Contingency Table:
- Construct a table summarizing the frequencies of the variables.
3. Calculate the Expected Frequencies:
- For each cell in the table, calculate the expected frequency using the formula: Eij=(Row Total × Column Total)Grand Total
4. Compute the Chi-Square Statistic:
- Use the formula: χ2=∑(Oij−Eij)^2/Eij
- Where O_{ij} is the observed frequency and E_{ij} is the expected frequency for each cell.
5. Determine the Degrees of Freedom:
- Degrees of freedom (df) is calculated as (number of rows−1)×(number of columns−1).
6. Compare to the Critical Value:
- Compare the computed Chi-Square statistic to the critical value from the Chi-Square distribution table based on the degrees of freedom and the significance level (α).
7. Make a Decision:
- If the Chi-Square statistic is greater than the critical value, reject the null hypothesis.
Common Challenges and How to Overcome Them
Analyzing categorical data using Chi-Square tests can present several challenges. Understanding these common issues and how to address them can significantly enhance your accuracy and confidence in performing these tests.
1. Data Preparation Issues:
One common challenge is ensuring that your data is properly categorized and formatted for the Chi-Square test. If your data isn't organized into the appropriate contingency tables or categories, the test results may be invalid. To overcome this, double-check your data entry and categorization. Ensure that your data is grouped correctly and meets the assumptions of the Chi-Square test.
2. Assumptions Violations:
Chi-Square tests assume that the data are independent and that expected frequencies in each category are sufficiently large (typically at least 5). If these assumptions are violated, the test results may be inaccurate. To address this, use statistical software to check and confirm that your data meets these criteria before performing the test. If necessary, consider combining categories to meet the minimum frequency requirement.
3. Interpreting Results:
Interpreting the results of Chi-Square tests can be challenging, especially when dealing with p-values and determining statistical significance. Make sure to understand the meaning of the Chi-Square statistic, degrees of freedom, and p-values. Practice interpreting results with sample problems to build your confidence. Consulting textbooks or online resources can also provide clarification.
4. Managing Multiple Comparisons:
When performing multiple Chi-Square tests, the risk of Type I error increases. This means that you might incorrectly reject a null hypothesis when it is actually true. To manage this, use correction methods such as the Bonferroni correction to adjust your significance level and reduce the likelihood of false positives.
5. Software Usage:
Learning to use statistical software for Chi-Square tests can be challenging if you’re unfamiliar with the tools. Spend time familiarizing yourself with the software’s functions and capabilities. Many programs offer tutorials and user guides that can help you navigate through the analysis process.
Applying Chi-Square Tests to Real-World Data
Applying Chi-Square tests to real-world data allows you to validate theoretical findings and make practical decisions based on empirical evidence. By testing hypotheses about categorical variables, you can uncover relationships, trends, and patterns in actual datasets. This practical application enhances your analytical skills and provides actionable insights for diverse fields and research.
Example: Chi-Square Goodness of Fit Test
Imagine you are a market researcher interested in whether the distribution of preferences for a new product matches the expected distribution. You surveyed 200 people and found the following preferences:
- Strongly Like: 50
- Like: 70
- Neutral: 30
- Dislike: 30
- Strongly Dislike: 20
Your expected distribution is based on previous research: 20% strongly like, 30% like, 20% neutral, 20% dislike, and 10% strongly dislike.
1. State the Hypotheses:
- H_0: The observed distribution matches the expected distribution.
- H_1: The observed distribution does not match the expected distribution.
2. Calculate the Expected Frequencies:
- Expected frequencies: 40, 60, 40, 40, 20
3. Compute the Chi-Square Statistic:
- χ2=[(50−40)^2]/40 + [(70−60)^2}/60 + [(30−40)^2]/40 + [(30−40)^2]/40+ [(20−20)^2]/20
- χ2=2.5 + 1.67 + 2.5 + 2.5 + 0= 9.17
4. Determine the Degrees of Freedom:
- df=5−1=4
5. Compare to the Critical Value:
- For α=0.05 and df=4, the critical value is 9.488.
6. Make a Decision:
- Since 9.17 < 9.488, do not reject H_0. The observed distribution matches the expected distribution.
Advanced Considerations
When using Chi-Square tests, it's essential to consider factors such as sample size and expected frequency to ensure valid results. For multiple comparisons, apply corrections like the Bonferroni adjustment to control Type I errors. Additionally, use software tools for accurate calculations and detailed results, and report findings comprehensively for robust analysis.
Dealing with Multiple Comparisons
When performing multiple Chi-Square tests, the risk of Type I error increases. To address this, you can use methods such as the Bonferroni correction, which adjusts the significance level to account for multiple comparisons. For instance, if you are performing five tests and want to maintain an overall α=0.05, you can use an adjusted significance level of α=0.05/5=0.01 for each individual test.
Reporting and Interpreting Results
When reporting Chi-Square test results, include the following:
- The Chi-Square statistic (χ2)
- Degrees of freedom (df)
- P-value
- Effect size (if applicable)
Provide a clear interpretation of the results, explaining the implications for your research question or hypothesis. For example, in a Chi-Square Test for Independence, discuss the nature of the association between the variables if the test is significant.
Using Software Tools
While manual calculations are useful for understanding the concepts, using software tools can streamline the process and reduce errors. Popular statistical software for conducting Chi-Square tests includes:
- R: A powerful statistical programming language with built-in functions for Chi-Square tests.
- Python: Libraries such as SciPy and pandas make it easy to perform Chi-Square tests.
- SPSS: A user-friendly software package for statistical analysis with straightforward procedures for Chi-Square tests.
- Excel: Excel has built-in functions and tools for performing Chi-Square tests, making it accessible for those familiar with spreadsheets.
Additional Tips for Success in Statistics Assignments on Chi-Square Tests
Mastering Chi-Square tests requires a solid understanding of concepts, careful data preparation, and practical application. Use statistical software for accurate results and seek expert help if needed. Clear interpretation and reporting of your findings are essential. Following these tips will enhance your skills and ensure success in your statistics assignments.
1. Understand the Basics
A solid grasp of the fundamental concepts behind Chi-Square tests is essential for accurate analysis. Familiarize yourself with the key principles of the Chi-Square Goodness of Fit Test, which assesses whether observed data fits a specific distribution, and the Chi-Square Test for Independence, which examines relationships between categorical variables. Knowing the assumptions, calculation methods, and interpretations of these tests will help you apply them correctly in your assignments. A strong foundation in these basics ensures reliable results and a better understanding of statistical analysis in your studies.
2. Prepare Your Data Carefully
Accurate data preparation is critical for valid Chi-Square test results. Start by ensuring your data is appropriately categorized into distinct groups, and verify that all expected frequencies are sufficiently large, typically at least five, to meet the test's assumptions. Clean and organize your data to avoid errors and ensure that it aligns with the test requirements. Properly formatted and well-prepared data not only facilitates accurate calculations but also enhances the reliability of your analysis and conclusions, leading to more robust results in your statistics assignment.
3. Practice with Real Data
Applying Chi-Square tests to real-world datasets is crucial for gaining hands-on experience and deepening your understanding. Working with actual data helps you encounter and solve practical issues such as managing different data distributions and interpreting results. This practical approach enhances your problem-solving skills and prepares you for various scenarios you might face in assignments. Additionally, it allows you to see how theoretical concepts translate into real-world applications, improving both your confidence and accuracy in using Chi-Square tests effectively.
4. Use Statistical Software
Leveraging statistical software is crucial for accurate and efficient Chi-Square test analysis. Tools such as R, Python, SPSS, and Excel streamline calculations, handle complex data sets, and minimize errors. These programs offer built-in functions for Chi-Square tests, enabling you to perform analyses quickly and interpret results with ease. By using statistical software, you can focus on understanding and applying the results, rather than getting bogged down in manual calculations. Embrace these tools to enhance your analysis and achieve reliable outcomes in your statistics assignments.
5. Seek Help When Needed
If you encounter challenges while working on Chi-Square tests, don’t hesitate to seek help. Professional data analysis assignment help services, such as those provided by experts in statistics, can offer valuable guidance and support. These services can clarify complex concepts, assist with calculations, and provide detailed explanations to ensure you understand how to apply Chi-Square tests effectively. Utilizing expert help not only improves your assignment quality but also enhances your overall grasp of statistical analysis, leading to better academic performance and confidence in your skills.
6. Interpret Results Clearly
Accurately interpreting and reporting your Chi-Square test results is crucial for drawing meaningful conclusions. Start by clearly stating the Chi-Square statistic, degrees of freedom, and p-value. Explain whether the results support or reject your null hypothesis, and discuss their implications in the context of your research question. Include the expected versus observed frequencies and any significant patterns or associations found. A thorough and transparent interpretation ensures that your analysis is credible and provides valuable insights into the relationships within your data.
By following these tips, you can enhance your understanding and application of Chi-Square tests, leading to successful statistics assignments and a deeper appreciation of categorical data analysis.
Conclusion
The Chi-Square Goodness of Fit Test and the Chi-Square Test for Independence are indispensable tools for analyzing categorical data. By understanding the principles behind these tests and following the outlined steps, you can effectively solve your statistics assignment and interpret the results. Whether you're examining the distribution of preferences for a new product or investigating the association between variables in a contingency table, these tests provide a robust framework for statistical analysis. Remember to prepare your data meticulously, use appropriate software tools, and interpret your results within the context of your study. By doing so, you'll enhance your analytical skills and contribute meaningful insights to your research. Practice and familiarity with Chi-Square tests will build your confidence and proficiency, enabling you to tackle any statistics assignment with ease and accuracy. Embrace these powerful tools to unlock the full potential of your categorical data analysis.