Analyzing Cognitive Biases and Factorial Design in Experimental Data
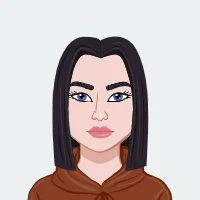
When it comes to completing your statistics assignment, especially those involving experimental design and data analysis, the process can seem daunting. These assignments require a deep understanding of experimental setups, data collection methods, and analytical techniques. Key topics such as cognitive biases and factorial designs are often at the heart of these tasks. This blog will provide a comprehensive approach to tackling such assignments, ensuring you understand how to define variables, design experiments, collect data accurately, and analyze results effectively. Whether you're studying psychological phenomena or other fields, following a structured method will help you excel in your statistics assignments. By breaking down the steps and highlighting common pitfalls, this guide aims to make the process more manageable and improve your confidence in handling complex data analysis tasks. Dive in to learn how to approach and solve your statistics assignment with clarity and precision.
Understanding Experimental Design
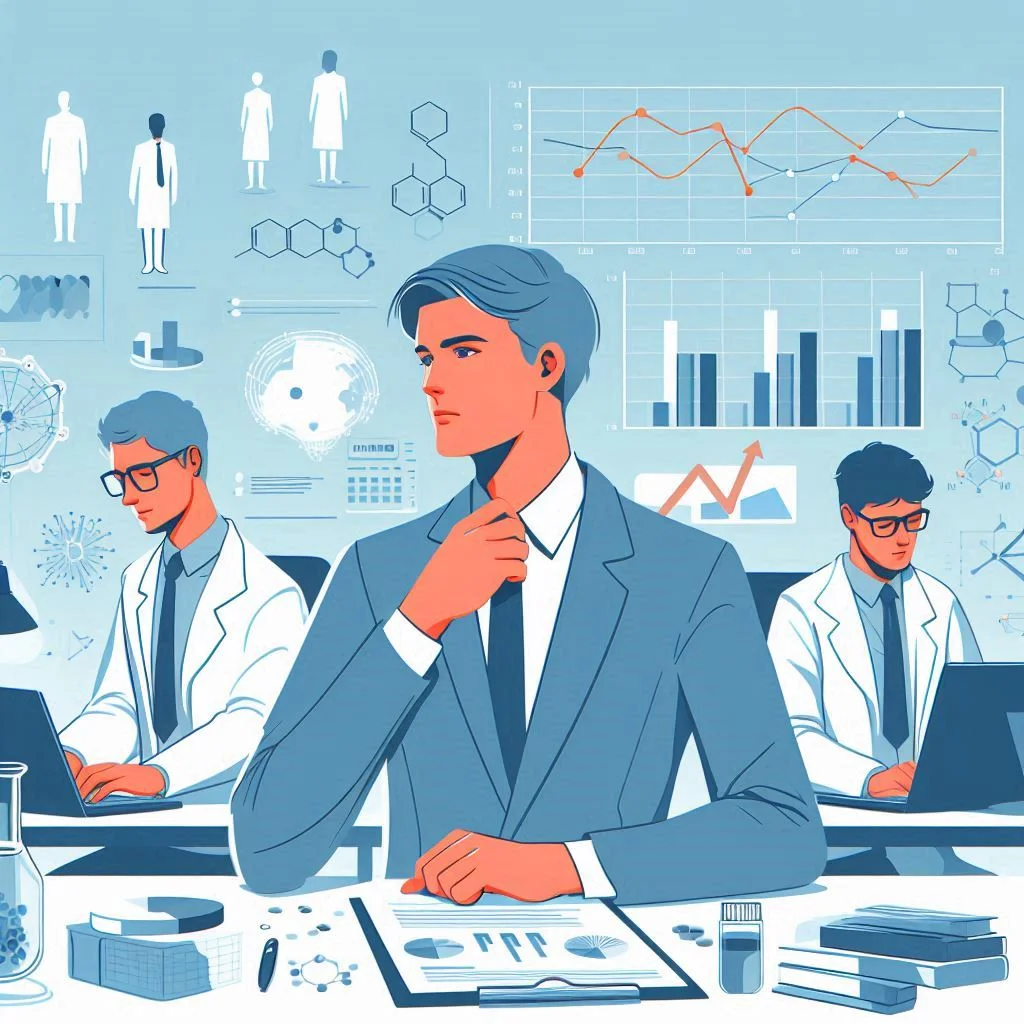
Experimental design is the foundation of any research study. It involves planning how to collect data to provide clear and interpretable results. One common design used in psychological experiments and other fields is the factorial design.
Factorial Design: A factorial design allows researchers to study the effects of two or more independent variables simultaneously. For instance, a 2x2 factorial design involves two independent variables, each with two levels. This design is advantageous because it enables the examination of both the main effects of each variable and their interaction effects.
Steps to Setting Up Your Experiment
1. Define Your Variables:
- Independent Variables: These are the variables you manipulate. In a psychological experiment, these could be the type of participant (e.g., depressed vs. non-depressed) and the valence of words (e.g., positive vs. negative).
- Dependent Variable: This is the outcome you measure. In our example, it could be the proportion of words recalled by the participants.
2. Create a Hypothetical Dataset:
- Construct a set of hypothetical means to illustrate potential outcomes. This helps in visualizing the results and understanding the relationships between variables.
3. Design the Experiment:
- Detail the procedures for your experiment, including participant selection, assignment to conditions, and the tasks they will perform.
Data Collection and Analysis
Data collection and analysis are crucial steps in any experimental study. Proper data collection ensures the reliability and validity of your results, while data analysis helps in interpreting the findings.
Data Collection
1. Participant Recruitment:
- Recruit participants who fit the criteria for your study. Ensure you have a sufficient sample size to achieve statistical power.
2. Random Assignment:
- Randomly assign participants to different conditions to control for confounding variables. This helps in ensuring that any observed effects are due to the independent variables.
3. Task Design:
- Design tasks that participants will perform during the experiment. Ensure that these tasks are consistent and unbiased.
4. Data Recording:
- Record data accurately and systematically. Use software tools if necessary to minimize human error.
Data Analysis
1. Descriptive Statistics:
- Start with descriptive statistics to summarize your data.
2. Inferential Statistics:
- Use inferential statistics to test your hypotheses. Common tests include ANOVA (Analysis of Variance) for factorial designs, which helps in examining main effects and interactions.
3. Interaction Effects:
- Analyze interaction effects to understand how the independent variables influence each other. In our example, you would look at how the interaction between participant type and word valence affects the recall of words.
Hypothesis Testing
Hypothesis testing involves making predictions about the relationships between variables and testing these predictions using statistical methods.
1. Formulate Hypotheses:
- Null Hypothesis (H0): This is the default assumption that there is no effect or no difference. For instance, there might be no difference in word recall between depressed and non-depressed participants.
- Alternative Hypothesis (H1): This is the opposite of the null hypothesis, suggesting that there is an effect or a difference. For example, there could be a significant difference in word recall based on participant type and word valence.
2. Select Significance Level:
- Choose a significance level (alpha), typically 0.05, which indicates a 5% risk of concluding that an effect exists when there is none.
3. Conduct Statistical Tests:
- Perform appropriate statistical tests to evaluate your hypotheses. For factorial designs, ANOVA is commonly used to test main effects and interactions.
4. Interpret Results:
- Analyze the results of your tests. If the p-value is less than the significance level, you reject the null hypothesis and accept the alternative hypothesis.
Presenting Your Results
Presenting your results effectively is crucial for conveying your findings. Use tables, graphs, and descriptive summaries to make your results clear and understandable.
1. Tables:
- Create tables to present your data systematically. Include means, standard deviations, and sample sizes for each condition.
2. Graphs:
- Use graphs to visually represent your data. Bar charts and line graphs are useful for showing differences between groups and interactions.
3. Descriptive Summaries:
- Provide descriptive summaries of your findings. Discuss the main effects, interaction effects, and their implications.
Practical Tips for Solving Statistics Assignments on Factorial Design in Experimental Data
Approaching statistics assignments involving factorial design can be challenging. Focus on clearly defining your variables and hypotheses. Use statistical software to handle complex data analysis, and always check for interactions between variables. Regularly practicing these steps and seeking help when needed will significantly enhance your skills and confidence in completing your statistics assignments.
1. Understand the Problem:
Before diving into your statistics assignment, it’s crucial to fully grasp the problem statement and research objectives. Carefully read through the assignment to identify key variables, such as independent and dependent variables, and understand their roles in the experiment. Determine the experimental design used, whether it’s a factorial design or another model, and recognize how it impacts data collection and analysis. Clarify the hypotheses being tested, including null and alternative hypotheses. By breaking down the problem into manageable components and identifying the underlying research questions, you set a solid foundation for your analysis. This thorough understanding will guide you through designing experiments, collecting data, and applying the appropriate statistical methods, ultimately leading to more accurate and meaningful results in your assignment.
2. Plan Your Approach:
Planning your approach is crucial for tackling any statistics assignment involving factorial design and experimental data. Begin by carefully reading the assignment prompt to understand the key variables and their relationships. Outline the steps needed to complete the task, including defining your independent and dependent variables, designing the experiment, and collecting data. Create a detailed plan for data analysis, specifying which statistical tests to use and how to interpret the results. Establish a timeline to manage your workload efficiently, ensuring you have ample time for each stage of the assignment. By organizing your approach methodically, you can address each component of the assignment systematically, minimize errors, and ensure that your final analysis is thorough and accurate. This structured planning will greatly enhance the quality and clarity of your work.
3. Use Software Tools:
Leveraging statistical software tools is essential for efficiently handling complex data analysis in your statistics assignments. Programs like SPSS, R, or Python provide powerful capabilities for managing large datasets, performing intricate calculations, and running statistical tests. These tools streamline the process of analyzing factorial designs, allowing you to conduct ANOVA, visualize interactions, and test hypotheses with ease. They offer built-in functions for generating descriptive statistics, creating graphs, and conducting post-hoc analyses, which can save time and reduce errors. By becoming proficient in these tools, you can enhance the accuracy and efficiency of your analysis. Additionally, many software platforms offer tutorials and support, making it easier to learn and apply their features effectively. Embracing these tools will significantly improve your ability to complete your statistics assignment with precision.
4. Seek Help When Needed:
Statistics assignments involving factorial design and data analysis can be complex and demanding. If you find yourself struggling with these tasks, don’t hesitate to seek help. Data analysis assignment help services are a valuable resource for students facing difficulties. These services offer expert guidance on experimental design, data analysis, and interpretation of results. Professional tutors and statisticians can provide personalized support, ensuring you understand the concepts and complete your assignments accurately. Whether you need help with setting up your experiment, analyzing data, or interpreting statistical outputs, data analysis assignment help services can offer the expertise and assistance you need. Utilizing these resources can alleviate stress and improve your performance, allowing you to tackle your statistics assignments with greater confidence and efficiency.
5. Practice Regularly:
Regular practice is essential for mastering factorial design and data analysis in statistics assignments. By frequently working on problems involving these concepts, you can deepen your understanding and improve your analytical skills. Start with simpler examples and gradually progress to more complex scenarios. This will help you become familiar with various types of factorial designs, data collection methods, and statistical techniques. Repeated exposure to these concepts not only enhances your problem-solving abilities but also helps you recognize patterns and common pitfalls in experimental data. Make use of practice exercises, past assignments, and online resources to continually challenge yourself. As you practice, you’ll build confidence and proficiency, making it easier to tackle complex assignments and achieve better results in your statistics coursework.
Common Pitfalls and How to Avoid Them
When working with factorial designs, avoid common pitfalls such as misinterpreting interaction effects and overlooking statistical assumptions. Ensure you correctly identify and address outliers, and always report effect sizes along with p-values. By carefully checking your data and analysis, you can avoid these errors and ensure accurate, reliable results.
1. Misinterpreting Interaction Effects:
Misinterpreting interaction effects can lead to incorrect conclusions about how variables influence each other. To avoid this, carefully analyze how the effect of one independent variable changes at different levels of another variable. Use graphical representations, such as interaction plots, to visualize these effects clearly. Understanding how interactions impact your results helps ensure that you accurately interpret the combined influence of multiple variables on the dependent measure.
2. Ignoring Assumptions:
Ignoring statistical assumptions can lead to inaccurate results and misleading conclusions. Ensure that your data meet the assumptions of normality, homogeneity of variance, and independence before conducting analyses. For factorial designs, verify these assumptions to avoid errors in interpreting main effects and interactions. If assumptions are violated, consider using alternative statistical methods or transforming your data. By adhering to these requirements, you can enhance the validity and reliability of your statistical findings.
3. Overlooking Outliers:
Outliers can significantly skew your results and lead to inaccurate conclusions. Failing to identify and address outliers may distort statistical analyses, affecting the validity of your findings. To avoid this, use diagnostic tools and visualizations, like box plots and scatter plots, to detect unusual data points. Decide whether to exclude outliers or apply robust statistical methods to account for them. Properly managing outliers ensures that your analysis reflects the true nature of your data and maintains its accuracy.
4. Failing to Report Effect Sizes:
Neglecting to report effect sizes can significantly impact the interpretation of your statistical results. Effect sizes provide insight into the magnitude of the relationships between variables, beyond mere statistical significance. Including effect sizes alongside p-values offers a more comprehensive understanding of your findings and their practical significance. Always report effect sizes to convey the real-world relevance of your results and ensure a more complete and accurate analysis of your experimental data.
Conclusion
Completing your statistics assignment involving experimental design and data analysis can be challenging, but with a systematic approach, it becomes manageable. By understanding key concepts such as cognitive biases and factorial designs, you can effectively design experiments, collect data, and analyze results. Remember to define your variables clearly, use appropriate statistical tools, and interpret your findings accurately. Avoid common pitfalls by checking assumptions, addressing outliers, and reporting effect sizes alongside p-values. Regular practice and seeking help when needed will further enhance your skills. By following the steps outlined in this blog, you'll be well-equipped to handle any statistics assignment with confidence. Ultimately, mastering these techniques will not only help you do your statistics assignment but also build a strong foundation for future research and data analysis tasks. Embrace these strategies and watch your proficiency in statistics grow.