Comparing Means Between Two Populations: Statistical Methods and Practices
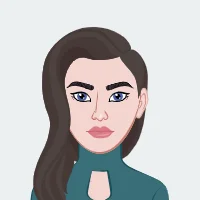
In the realm of statistical analysis, comparing means between two populations is a fundamental concept that underpins various research and data analysis tasks. Whether you're delving into coursework or tackling real-world data, understanding how to compare means accurately is crucial. This blog will guide you through the essential techniques for analyzing and comparing means from two distinct populations, providing you with practical strategies to solve your statistics analysis assignment effectively. From independent and paired samples t-tests to ANOVA, we'll cover the key methods and best practices you need to grasp. By mastering these concepts, you'll be well-equipped to handle assignments and research involving comparative data analysis, ensuring that you approach your statistical tasks with confidence and precision.
Understanding the Basics
Before diving into the specific techniques, it’s essential to grasp the foundational concepts of comparing means between two populations. The goal is often to determine if there is a significant difference between the means of two distinct groups. This can be useful in various scenarios, such as comparing test scores between two different classes, evaluating treatment effects, or assessing performance across different groups.
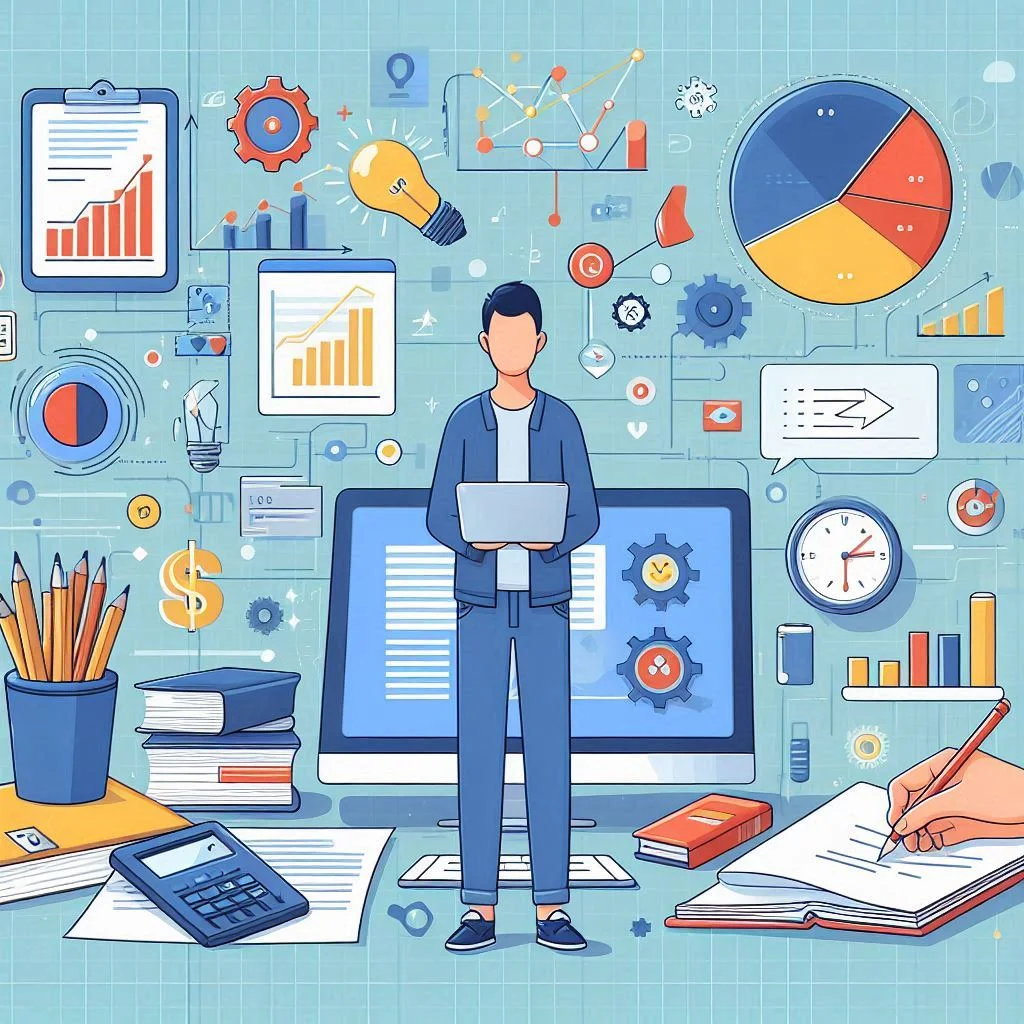
Key Concepts in Comparing Means
- Population and Sample: In statistics, a population is the entire group you're interested in studying, while a sample is a subset of this population. For comparing means, you typically work with samples from two populations.
- Mean: The mean, or average, is the sum of all values divided by the number of values. When comparing two means, you are essentially assessing whether the average value in one population is different from the average value in another.
- Variance and Standard Deviation: Variance measures the spread of data points around the mean, while standard deviation is the square root of variance. These measures are crucial for understanding the dispersion of data in each population.
Methods for Comparing Means
Several statistical methods can be used to compare means between two populations. The choice of method depends on factors such as the nature of your data, the sample size, and the assumptions you can meet.
1. Independent Samples T-Test
The independent samples T-test , also known as the two-sample t-test, is used to compare the means of two independent groups. This test assumes that the samples are independent and that the data follows a normal distribution.
Assumptions:
- The data from each population should be normally distributed.
- The variances of the two populations should be approximately equal (homogeneity of variances).
- The samples are independent of each other.
Procedure:
- State the Hypotheses: Formulate the null hypothesis (H0) and alternative hypothesis (H1). For example, H0 might state that there is no difference in means, while H1 suggests a significant difference.
- Calculate the Test Statistic: Use statistical software or formulas to calculate the t-value, which measures the difference between the sample means relative to the variability within the samples.
- Determine the P-Value: Compare the t-value to the critical value from the t-distribution table to find the p-value, which indicates the probability of observing the data if the null hypothesis is true.
- Make a Decision: Based on the p-value, decide whether to reject or fail to reject the null hypothesis.
Interpreting Results: If the p-value is less than the significance level (usually 0.05), it suggests a significant difference between the means of the two populations.
2. Paired Samples T-Test
The paired samples t-test is used when the samples are related or matched in some way. This might include measurements taken before and after an intervention on the same subjects.
Assumptions:
- The differences between paired observations should be normally distributed.
- The pairs are independent of each other.
Procedure:
- Step 1: State the Hypotheses: Formulate the null and alternative hypotheses based on the difference in means of paired observations.
- Step 2: Calculate the Test Statistic: Compute the mean of the differences between pairs, the standard deviation of these differences, and the t-value.
- Step 3: Determine the P-Value: Use the t-distribution to find the p-value associated with the calculated t-value.
- Step 4: Make a Decision: Compare the p-value to your significance level to determine if there is a significant difference.
Interpreting Results: A significant result indicates that the intervention or change had a measurable effect.
3. Analysis of Variance (ANOVA)
While ANOVA is typically used for comparing means among three or more groups, it can also be applied to two groups when more complex analysis is required.
Assumptions:
- The data should be normally distributed.
- The variances across groups should be equal.
- Observations should be independent.
Procedure:
- Step 1: State the Hypotheses: The null hypothesis (H0) usually states that all group means are equal, while the alternative hypothesis (H1) indicates that at least one group mean is different.
- Step 2: Calculate the Test Statistic: Use ANOVA to compute the F-value, which compares the variance between groups to the variance within groups.
- Step 3: Determine the P-Value: The p-value is found by comparing the F-value to the critical value from the F-distribution.
- Step 4: Make a Decision: If the p-value is less than the significance level, reject the null hypothesis.
Interpreting Results: A significant result suggests that there is a difference in means among the groups. Post-hoc tests can further identify which groups differ.
Practical Tips for Analyzing Data
To effectively analyze data, ensure your samples meet the necessary assumptions, such as normality and equal variances. Utilize statistical software for precise calculations and visualizations. Regularly check for biases and ensure your sample size is adequate. Accurate data handling and thorough analysis will enhance the reliability and clarity of your results.
- Check Assumptions: Before performing any statistical test, it's crucial to verify that your data meets the underlying assumptions. For example, many tests assume normality, where data should follow a bell-shaped curve, and homogeneity of variances, meaning the variability should be similar across groups. Checking these assumptions helps ensure the validity of your results. Use visual tools like histograms or Q-Q plots to assess normality and statistical tests like Levene’s test for equal variances. If assumptions are not met, consider alternative methods or data transformations. Accurate assumption checking is essential for reliable and meaningful statistical analysis.
- Use Statistical Software: Leveraging statistical software is crucial for efficient and accurate data analysis. Tools like R, SPSS, and Python offer robust features for conducting various statistical tests, including t-tests and ANOVA. These programs handle complex calculations, manage large datasets, and generate detailed reports and visualizations, which simplify the analysis process. By utilizing statistical software, you can minimize human error, quickly interpret results, and focus on drawing meaningful conclusions. Familiarity with these tools not only streamlines your workflow but also ensures that your statistical analyses are precise and reliable. Embracing technology in your assignments will enhance both efficiency and accuracy.
- Visualize Your Data: Visualizing your data is crucial for understanding and interpreting the results of your analysis. Use graphs and charts, such as histograms, box plots, or scatter plots, to illustrate the distribution and differences between groups. Visualization helps identify patterns, trends, and outliers that may not be evident from statistical summaries alone. It also provides a clear and intuitive way to present your findings to others. Effective visualizations can highlight key insights and support your conclusions, making your analysis more accessible and comprehensible. Ensure your visuals are well-labeled and easy to interpret to enhance the overall quality of your presentation.
- Consider Sample Size: Sample size plays a crucial role in statistical analysis, influencing the accuracy and reliability of your results. A larger sample size generally provides more accurate estimates and increases the power of your statistical tests, reducing the likelihood of Type I and Type II errors. Conversely, a small sample size may lead to less reliable results and can affect the robustness of your conclusions. When planning your analysis, consider whether your sample size is sufficient to detect meaningful differences between populations. If possible, use power analysis to determine the optimal sample size for your study to ensure that your findings are both valid and generalizable.
- Be Mindful of Biases: Biases in data analysis can significantly skew your results and lead to misleading conclusions. It's crucial to identify and address potential sources of bias, such as selection bias, measurement bias, or confirmation bias. Ensure that your sample is representative of the population you're studying and that your data collection methods are consistent and objective. Regularly review your analysis for any signs of bias and consider alternative explanations for your findings. By maintaining a critical perspective and implementing rigorous procedures, you can minimize bias and enhance the accuracy and reliability of your statistical results.
- Consult Resources: When tackling complex data analysis tasks and statistical inference assignments, consulting additional resources can be immensely helpful. Utilize textbooks, online tutorials, and academic journals to deepen your understanding of statistical methods and their applications. Online forums and communities can offer practical insights and solutions to specific problems you might encounter. If you're still unsure, consider seeking help from statistics assignment experts who can provide personalized guidance and clarify difficult concepts. By leveraging statistical interference assignment help services, you can enhance your analytical skills, ensure accurate results, and gain confidence in solving your statistics assignments effectively. Remember, seeking assistance is a step towards mastering complex statistical analyses.
Common Challenges and Solutions
Common challenges in comparing means include non-normal data, unequal variances, and small sample sizes. To address these, consider non-parametric tests for non-normal data, use Welch’s t-test for unequal variances, and apply bootstrapping methods for small samples. Adapting your approach to these issues ensures more accurate and reliable analysis.
- Non-Normal Data: When your data does not meet the normality assumption, traditional parametric tests like the t-test may not be appropriate. Instead, consider using non-parametric tests such as the Mann-Whitney U test for independent samples or the Wilcoxon signed-rank test for paired samples. These tests do not assume a normal distribution and are robust to deviations from normality. Additionally, data transformations or bootstrapping methods can sometimes help to normalize data or estimate confidence intervals. Addressing non-normal data properly ensures that your analysis remains valid and that your conclusions are reliable despite deviations from standard assumptions.
- Unequal Variances: When comparing means between two populations, unequal variances can complicate the analysis, as many tests assume homogeneity of variances. To address this issue, use Welch’s t-test, which adjusts for differences in variances between the two groups and provides a more accurate p-value in such cases. Additionally, consider transforming your data to stabilize variances or apply non-parametric tests like the Mann-Whitney U test if variances remain problematic. Ensuring that your statistical method accounts for unequal variances will lead to more reliable results and help prevent misleading conclusions from your data analysis.
- Small Sample Sizes: Small sample sizes can limit the reliability of statistical analyses by reducing the power of tests and increasing the potential for Type I and Type II errors. To address this, consider using bootstrapping techniques to estimate the sampling distribution and improve the robustness of your results. Alternatively, you can apply corrections for small sample sizes, such as the t-distribution adjustments in hypothesis testing. If possible, increasing your sample size can also enhance the accuracy and reliability of your results. Small sample sizes require careful handling to ensure that your conclusions are valid and meaningful in your statistical analysis.
- Multiple Comparisons: When performing multiple statistical tests, the risk of Type I errors—finding false positives—increases. To address this, apply corrections such as the Bonferroni or Holm-Bonferroni methods, which adjust the significance threshold to account for the number of comparisons. Another approach is the False Discovery Rate (FDR) procedure, which controls the expected proportion of false positives. By incorporating these adjustments, you can mitigate the risk of incorrect conclusions and enhance the reliability of your findings. Ensuring robust methods for handling multiple comparisons is crucial for maintaining the integrity of your statistical analysis and ensuring accurate results in your assignments.
Reporting and Interpretation
When presenting your results, clearly communicate the findings and their implications. Include tables, graphs, and a narrative explanation of the statistical tests used and the results obtained. Discuss the practical significance of your findings and any limitations of your analysis.
Conclusion
Successfully comparing means between two populations involves more than just applying statistical tests; it requires a clear understanding of the methods and their appropriate usage. As you work through your statistics assignments, remember to verify your data assumptions, select the right statistical tests, and interpret your results accurately. With the techniques and practices outlined in this blog, you'll be better prepared to tackle these analyses and solve your statistics assignment with proficiency. Whether you’re handling simple comparisons or more complex data, these principles will help ensure that your analysis is robust and reliable. Keep these guidelines in mind as you approach your Statistics assignments and research, and seek further assistance if needed to achieve the best results.