Confidence Intervals: Calculating and Interpreting Statistical Data for Accurate Analysis
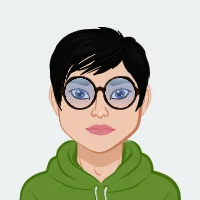
Confidence intervals are a fundamental concept in inferential statistics, offering a method to estimate the range within which a population parameter lies based on sample data. Understanding and calculating confidence intervals is essential for anyone looking to solve your statistics assignment effectively. These intervals provide critical insights into data variability and reliability, making them invaluable in fields ranging from research to business analytics. This blog will guide you through the key steps and techniques necessary to solve your statistics assignment involving confidence intervals. We will explore the principles behind confidence intervals, walk through the calculation process, and highlight practical tips for using tools like Excel to streamline your work. By the end of this guide, you will have a solid grasp of how to approach confidence interval problems, ensuring accuracy and confidence in your statistical analyses.
Understanding Confidence Intervals
A confidence interval (CI) is a range of values derived from sample data that is likely to contain the true value of an unknown population parameter.
For example, a 95% confidence interval for a population mean suggests that if we were to take 100 different samples and compute a confidence interval for each sample, approximately 95 of the 100 confidence intervals will contain the true population mean.
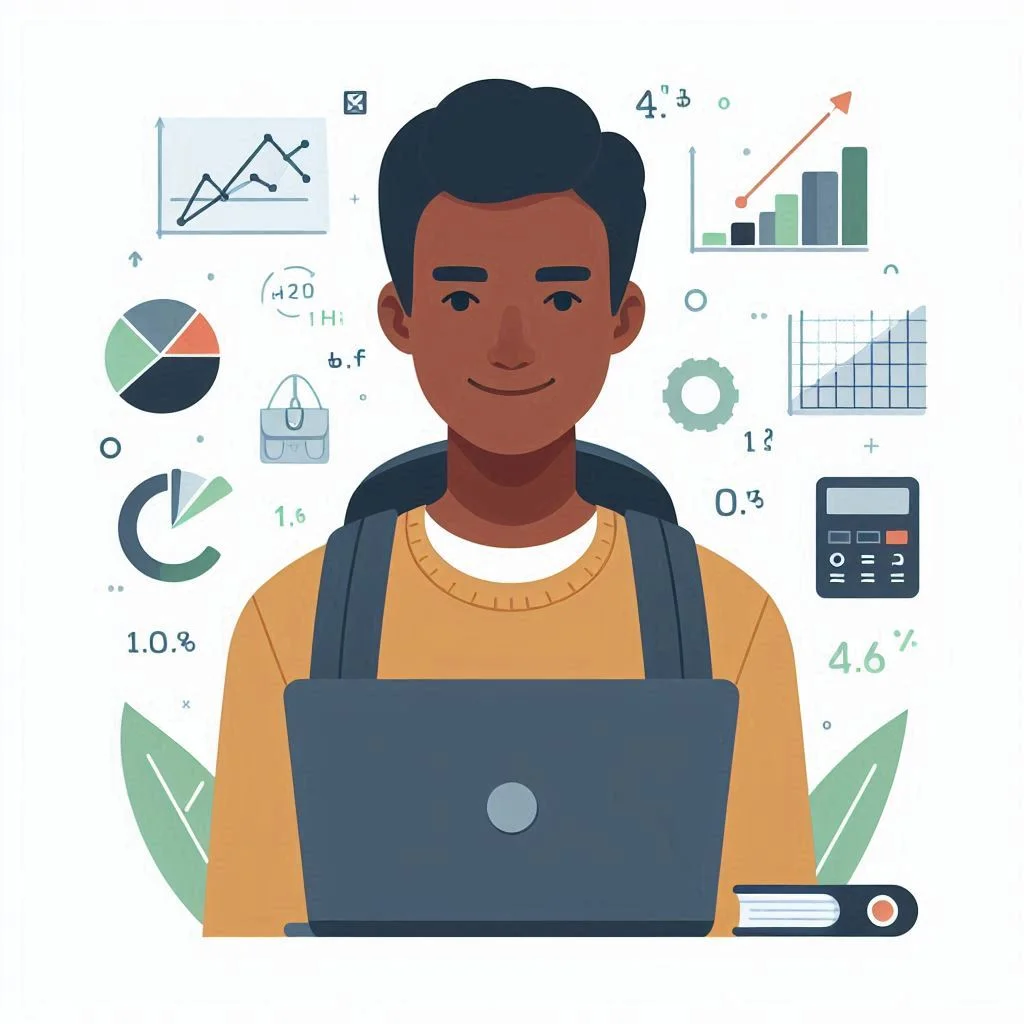
Key Concepts
- Point Estimate: A single value estimate of a population parameter. For the mean, this is the sample mean (xˉ).
- Margin of Error (MoE): The range within which we expect the population parameter to lie, calculated from the standard error and critical value.
- Confidence Level: The probability that the confidence interval contains the true population parameter (e.g., 95%, 98%).
- Critical Value (z or t): A value from the standard normal (z) or t-distribution used to calculate the margin of error.
- Standard Error (SE): The standard deviation of the sampling distribution, calculated as σ sqrt{n} for known population standard deviation or s sqrt{n} for sample standard deviation.
Step-by-Step Guide to Solving Confidence Interval Problems
This section breaks down the process of solving confidence interval problems into clear, manageable steps. By following this guide, you will learn how to gather data, calculate key statistics, determine critical values, and interpret your results. These steps will help you efficiently and accurately complete your data analysis assignment.
Step 1: Understand the Problem Statement
Understanding the problem statement is the first crucial step in solving confidence interval problems. Carefully read the assignment to identify what is being asked. Determine the population parameter of interest, such as the mean or proportion, and recognize any given data points, such as the sample size, sample mean, or standard deviation. Understanding the confidence level required is also essential, as this will affect your calculations and interpretations.
Clarify any assumptions or constraints mentioned in the problem, such as the distribution of the population or any known population parameters. This step ensures that you are clear on the objectives and requirements before proceeding with data analysis. By thoroughly understanding the problem statement, you lay a solid foundation for accurate and efficient statistical analysis, enabling you to solve your statistics assignment with confidence and precision.
Step 2: Gather Data and Calculate Sample Statistics
Once you have a clear understanding of the problem statement, the next step is to gather the necessary data and calculate the sample statistics. Begin by collecting the sample data provided in the assignment. This data could be a set of measurements, observations, or any relevant figures.
Calculate the sample mean (average) by summing all the data points and dividing by the number of observations. The sample mean provides an estimate of the central tendency of the data. Next, determine the sample standard deviation, which measures the dispersion or spread of the data points around the mean.
Additionally, identify the sample size, which is the total number of observations in your dataset. These statistics—sample mean, sample standard deviation, and sample size—are essential for constructing confidence intervals.
Accurate calculation of these sample statistics is critical, as they form the basis for further steps in solving your statistics assignment. Utilizing tools like Excel can streamline these calculations, ensuring precision and efficiency in your analysis.
Step 3: Determine the Confidence Level
Determining the confidence level is a critical step in calculating confidence intervals. Common confidence levels include 90%, 95%, and 99%, with higher levels indicating greater confidence but resulting in wider intervals.
To determine the confidence level for your assignment, refer to the problem statement or guidelines provided. This percentage reflects how often the true parameter is expected to fall within the calculated interval if the experiment were repeated multiple times. For instance, a 95% confidence level implies that in 95 out of 100 samples, the interval will contain the true parameter.
Once you have identified the required confidence level, you can use it to find the corresponding critical value, which is essential for calculating the margin of error. Understanding and correctly applying the confidence level is vital to solve your statistics assignment accurately and draw reliable conclusions from your data.
Step 4: Calculate the Standard Error
The standard error (SE) quantifies the variability of the sample mean and is crucial for constructing confidence intervals. Calculating the standard error involves the following steps:
1. Identify the Standard Deviation: Determine whether you are using the population standard deviation (σ) or the sample standard deviation (s). Use the population standard deviation if it's provided; otherwise, use the sample standard deviation calculated from your data.
2. Calculate the Standard Error:
- If the population standard deviation is known, use the formula: SE=σ sqrt{n}}
- If the population standard deviation is unknown and only the sample standard deviation is available, use: SE= {s}{\sqrt{n}. Here, n is the sample size.
3. Interpret the Result: The standard error reflects the precision of your sample mean as an estimate of the population mean. A smaller standard error indicates a more precise estimate.
By accurately calculating the standard error, you ensure that your confidence interval reflects the true variability and reliability of your sample data.
Step 5: Find the Critical Value (z-score or t-score)
Finding the critical value is a pivotal step in constructing confidence intervals. The critical value corresponds to the desired confidence level and helps determine the margin of error. The choice between using a z-score or a t-score depends on sample size and whether the population standard deviation is known.
- For Large Samples or Known Population Standard Deviation: Use the z-score, which comes from the standard normal distribution. For example, a 95% confidence level corresponds to a z-score of approximately 1.96. You can find this using statistical tables or Excel’s NORM.S.INV function by inputting 0.975 (since 0.95 confidence level leaves 0.05 in the tails, divided equally into 0.025 for each tail).
- For Small Samples or Unknown Population Standard Deviation: Use the t-score from the t-distribution, which accounts for additional variability. The t-score is determined based on the sample size and degrees of freedom (n-1). Use Excel’s T.INV.2T function to find the critical t-value by inputting the significance level (e.g., 0.05 for a 95% confidence interval) and degrees of freedom.
Selecting the correct critical value is crucial for accurate confidence interval calculation, ensuring your results are reliable and reflective of the desired confidence level.
Step 6: Calculate the Margin of Error (MoE)
Calculating the Margin of Error (MoE) is a key step in constructing a confidence interval, as it determines the range within which the population parameter is likely to lie. To compute the MoE, you need the critical value and the standard error.
- Determine the Critical Value: This value, which corresponds to the desired confidence level (e.g., 95% or 99%), can be found using the standard normal distribution (z-score) or the t-distribution, depending on your sample size and whether the population standard deviation is known. For example, a 95% confidence level corresponds to a z-score of approximately 1.96.
- Calculate the Standard Error (SE): The standard error is calculated as the standard deviation of the sample mean, given by SE=σ{\sqrt{n} for a known population standard deviation, or SE= {s}{\sqrt{n}} for an unknown population standard deviation.
- Compute the MoE: Multiply the critical value by the standard error:
MoE=Critical Value × SE
This result gives you the range around the sample mean where the true population parameter is expected to lie with the specified level of confidence.
By accurately calculating the MoE, you ensure that your confidence interval reflects the precision of your estimate and the reliability of your data analysis.
Step 7: Construct the Confidence Interval
Constructing the confidence interval involves calculating the range within which the population parameter is likely to lie based on your sample data. Follow these steps to accurately build your confidence interval:
1. Calculate the Margin of Error (MoE): Multiply the standard error (SE) by the critical value (z-score or t-score) that corresponds to your confidence level. The formula is:
MoE=Critical Value × SE
2. Determine the Interval Bounds: Use the sample mean (xˉ) and the margin of error to find the lower and upper bounds of the confidence interval. The formulas are:
Lower Bound=xˉ−MoE
Upper Bound=xˉ+MoE
3. Interpret the Interval: The confidence interval is now complete and represents the range in which you expect the true population parameter to fall with the specified confidence level. Clearly articulate this range in your results.
By constructing the confidence interval, you provide a statistically sound estimate of where the true parameter lies, enhancing the reliability of your conclusions and making it easier to solve your statistics assignment effectively.
Step 8: Interpret the Results
Start by examining the range defined by the confidence interval, which includes a lower and an upper bound. This interval provides an estimated range within which the true population parameter is likely to lie, based on your sample data.
Clearly articulate what the confidence interval means in the context of your assignment. For instance, if you calculated a 95% confidence interval for the mean, explain that you are 95% confident the true population mean falls within this interval. Avoid misinterpretations; remember that the confidence level reflects the reliability of the interval estimate across many samples, not the probability that any single interval contains the parameter.
Provide a concise summary of your findings and their implications, considering the context of the problem. This helps ensure that your results are understood and applied correctly, allowing for informed decisions and analyses based on your statistical findings.
Practical Tips for Using Excel
Excel is a powerful tool for statistical analysis. Here are some practical tips for using Excel functions to perform statistical calculations for confidence intervals:
Using Excel Functions for Statistical Analysis
- COUNT: To find the sample size.
- AVERAGE: To calculate the sample mean.
- STDEV.P or STDEV.S: To calculate the population or sample standard deviation.
- NORM.S.INV: To find the critical value (z-score) for the given confidence level.
- Formulas: Use cell references to ensure dynamic calculations that update if the data changes.
Common Pitfalls and How to Avoid Them
Understanding common pitfalls in confidence interval calculations can prevent errors in your analysis. Common issues include misinterpreting confidence levels, ignoring sample size effects, and overlooking assumptions. By being aware of these pitfalls and applying correct methodologies, you can avoid inaccuracies and enhance the reliability of your results in solving your statistics assignment.
Misinterpreting Confidence Intervals
One common mistake is to interpret a 95% confidence interval as meaning that there is a 95% probability that the population parameter lies within the interval. Instead, it means that if we were to take many samples and construct a confidence interval from each, 95% of those intervals would contain the population parameter.
Ignoring Sample Size
The sample size has a significant impact on the confidence interval. Larger samples provide more precise estimates, leading to narrower confidence intervals. Always ensure your sample size is adequate for reliable estimates.
Not Checking Assumptions
Confidence intervals assume that the sample data is randomly selected and follows a normal distribution (or the sample size is large enough for the Central Limit Theorem to apply). Verify these assumptions before proceeding with calculations.
Conclusion
Confidence intervals are powerful tools that allow us to make informed estimates about population parameters based on sample data. Whether you are working on research, business analytics, or quality control, mastering the calculation and interpretation of confidence intervals is crucial for data-driven decision-making. By following the structured approach outlined in this blog, you can solve your statistics assignment with confidence. Remember to carefully check assumptions, leverage tools like Excel for efficient calculations, and interpret your results accurately. As you continue to apply these concepts, you will become more adept at handling statistical challenges, leading to more precise and reliable conclusions. With practice and the right techniques, solving your statistics assignment will become a more manageable and rewarding task, enhancing your overall understanding of statistical data analysis.