Effective Data Management, Analysis, and Reporting Techniques in Statistics
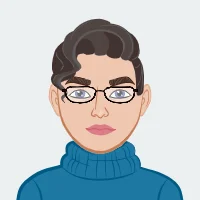
Statistics assignments often present a myriad of tasks that can seem daunting at first. However, by breaking these tasks down into manageable steps, you can approach them with confidence and clarity. Whether you are working on data management, descriptive statistics, or data analysis, mastering these techniques is crucial. This guide is designed to help you solve your statistics assignment efficiently and effectively. We will cover key topics such as data preparation, scale score calculation, variable transformation, and correlation analysis. By following this systematic approach, you will be able to handle any similar assignment with ease. The aim is to equip you with the knowledge and tools needed to navigate through complex statistical analyses and produce clear, well-documented reports..
1. Data Management and Preparation
Effective data management and preparation are crucial for accurate analysis. This involves cleaning the data, handling missing values, merging datasets, and ensuring proper data documentation. By organizing your data efficiently, you set a strong foundation for subsequent statistical analyses, leading to more reliable and insightful results.
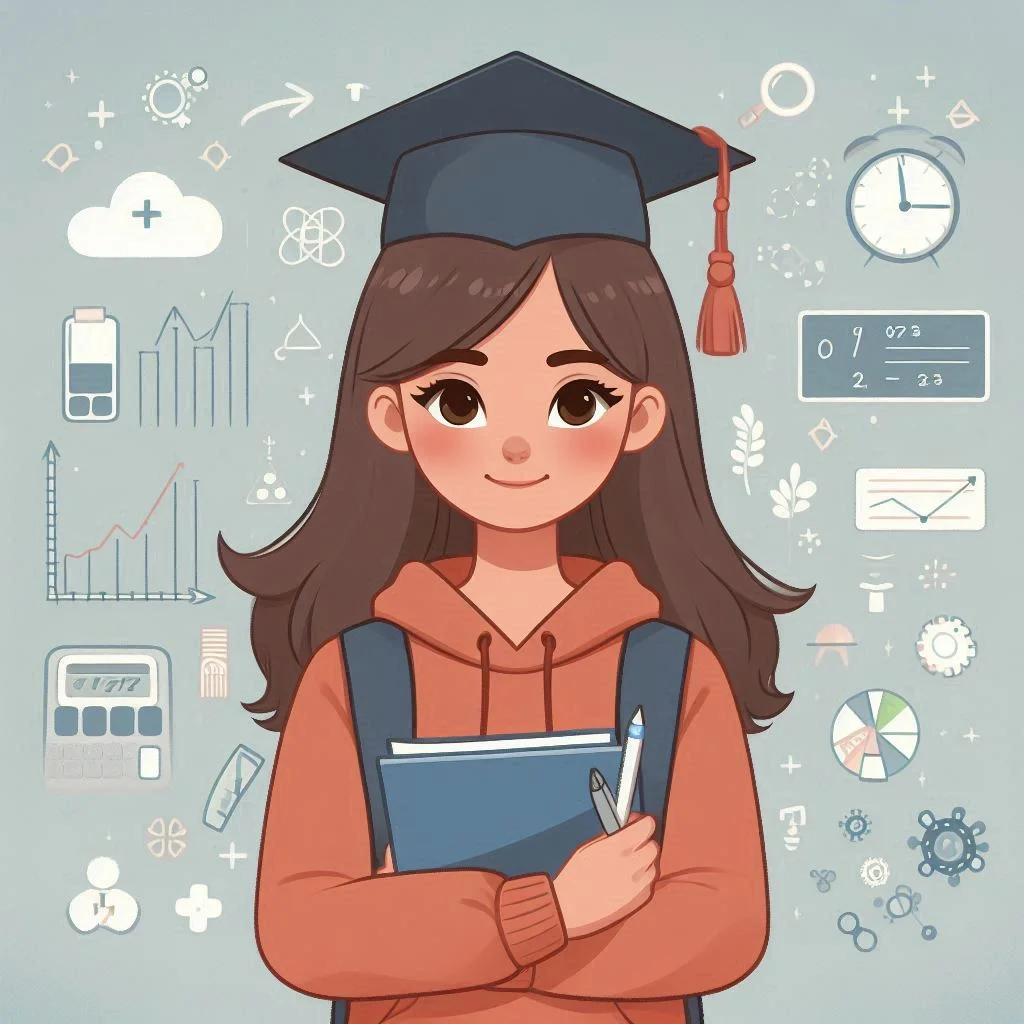
- Merging Datasets: When working with multiple datasets, it’s crucial to merge them correctly to ensure that all data points align properly. Use tools like SPSS, R, or Python to combine datasets using common keys or identifiers. Merging datasets involves combining different data files into a single file, ensuring that all the relevant information is available for analysis. This step is essential for comprehensive data analysis and helps in avoiding fragmented or incomplete data interpretation. Properly merging datasets requires careful attention to detail, ensuring that the keys or identifiers used for merging are consistent and accurately match across datasets.
- Cleaning Data: Before analysis, check for and handle missing or inconsistent data. This might involve imputing missing values, correcting errors, or removing irrelevant data points. Data cleaning ensures that the dataset is accurate, consistent, and free of errors. It involves several steps, including identifying and handling missing data, removing duplicates, and correcting errors or inconsistencies. Cleaning data is a critical step because the quality of the data directly impacts the accuracy and reliability of the analysis. Techniques such as imputation, outlier detection, and normalization are often used to clean and prepare the data for further analysis.
2. Descriptive Statistics
Descriptive statistics include measures such as means, medians, and standard deviations, which provide insights into the central tendency and variability of your data. By analyzing these statistics, you can uncover trends and patterns, making it easier to interpret and present your findings.
- Exploring Data: Start by obtaining descriptive statistics for all variables. This provides a summary of the data, including means, medians, modes, standard deviations, and frequency distributions. It helps you understand the basic features of the data and identify any anomalies. Descriptive statistics provide a snapshot of the data and help in understanding its distribution and central tendencies. By summarizing the data, you can identify patterns, trends, and outliers that might influence the analysis. Common descriptive statistics include measures of central tendency (mean, median, mode), measures of variability (range, variance, standard deviation), and frequency distributions. These statistics provide valuable insights into the data's overall structure and characteristics.
3. Calculating Value-Expectancies
Calculating value-expectancies involves assessing the perceived value of outcomes and their expected benefits. This is done by multiplying expectancy variables with value variables, allowing you to gauge how strongly different aspects of a variable are valued. This method helps in understanding the relative importance and anticipated impact of various factors in your analysis.
- Value-Expectancy Framework: In assignments that involve psychological or behavioral data, you might need to derive value-expectancies. This involves combining expectancy and value measures for different outcomes. For example, multiply the expectancy (e.g., likelihood of an event) by the value (e.g., importance of that event). The value-expectancy framework is a theoretical model that explains how individuals make decisions based on their expectations of outcomes and the value they place on those outcomes. By calculating value-expectancies, you can quantify the expected benefits or drawbacks of different actions or behaviors. This framework is commonly used in fields such as psychology, health, and education to predict and understand behavior.
4. Reliability Analysis
Reliability analysis evaluates the consistency and stability of measurement instruments. Cronbach's alpha is commonly used to assess the internal consistency of scales, indicating how well different items measure the same underlying construct. High reliability ensures that your measurement tools produce consistent results, enhancing the credibility of your research findings.
- Cronbach’s Alpha: To assess the internal consistency of scales (e.g., a set of survey items measuring the same construct), calculate Cronbach’s alpha. This statistic helps ensure that the items reliably measure the same underlying concept. Cronbach's alpha ranges from 0 to 1, with higher values indicating greater reliability. A high Cronbach's alpha suggests that the items on a scale measure the same underlying construct and are likely to produce consistent results. This analysis is crucial for validating the reliability of scales used in surveys and questionnaires.
5. Scale Score Calculation
Scale score calculation involves computing aggregate scores from individual indicators to represent broader constructs. By averaging or summing items within a scale, you obtain a comprehensive measure that reflects overall trends and patterns. This process simplifies complex data and facilitates comparison across different groups or time periods.
- Composite Scores: Combine individual items into composite scores. For example, if you have multiple items measuring self-efficacy, calculate the mean or sum to create a single self-efficacy score. Composite scores are created by aggregating multiple individual items into a single score. This is often done to simplify analysis and interpretation, especially when dealing with complex constructs that are measured using multiple items. Composite scores provide a more comprehensive measure of the underlying construct and can improve the reliability and validity of the analysis. Common methods for creating composite scores include calculating the mean, sum, or weighted average of the individual items.
6. Variable Transformation
Variable transformation adjusts data to better meet analytical requirements or to enhance interpretability. This may include normalizing, categorizing, or computing new variables based on existing ones. Effective transformation improves the accuracy of statistical models and provides clearer insights into relationships and trends within your dataset.
- Creating New Variables: Transform raw data into meaningful categories or scales. For instance, you might categorize a continuous variable (e.g., heart rate) into ordinal categories (e.g., excellent, good, average) or create dichotomous variables (e.g., high vs. low). Variable transformation involves converting raw data into new variables that are more meaningful or easier to analyze. This can include creating categorical variables from continuous data, calculating ratios or percentages, or applying mathematical transformations such as logarithms. Variable transformation is often used to meet the assumptions of statistical tests, improve the interpretability of the data, or highlight specific patterns or relationships within the data.
7. Data Labeling
Data labeling assigns meaningful names and descriptions to variables and categories in your dataset. Proper labeling ensures clarity and consistency, making it easier to understand and interpret the data. This practice helps prevent confusion and errors, and facilitates smoother communication of results to stakeholders.
- Labeling Variables and Values: Ensure all variables and values are appropriately labeled in your dataset. This makes the data easier to understand and interpret, especially when sharing with others or when referring to it in reports. Proper labeling of variables and values is essential for clear communication and accurate analysis. Labels should be descriptive and meaningful, providing enough information to understand the variable's content and significance. In SPSS, you can assign labels to variables and their values using the VALUE LABELS and VARIABLE LABELS commands. Clear and consistent labeling helps prevent misunderstandings and errors in data interpretation and reporting.
8. Saving Modified Datasets
Saving modified datasets involves storing updated versions of your data after making changes. This step ensures that all modifications, such as new variables or corrected entries, are preserved for future analysis. By saving these versions, you maintain an accurate record of your data processing steps and ensure reproducibility of your results.
- Documenting Changes: After making modifications, save the updated dataset with a new name to preserve the original data. Maintain clear documentation of the changes made, including the steps and rationale. Documenting changes ensures transparency and reproducibility in your analysis. It allows others to understand the modifications made to the data and the reasons behind those changes. Keeping detailed records of the steps taken, the transformations applied, and any assumptions made is essential for ensuring the integrity of the analysis. Saving modified datasets with descriptive names helps track different versions of the data and facilitates future analysis or review.
9. Subgroup Analysis
Subgroup analysis involves examining specific segments within your data to uncover patterns or differences. By analyzing subgroups, you can identify variations across different categories such as age, gender, or treatment groups. This approach enhances the depth of your analysis and provides more detailed insights relevant to diverse populations or conditions.
- Comparing Groups: Analyze data for different subgroups (e.g., treatment vs. control groups). Obtain descriptive statistics for each group to identify patterns or differences. This step is crucial for understanding the impact of interventions or identifying trends within specific populations. Subgroup analysis involves breaking down the data into smaller groups based on specific characteristics or criteria. By comparing these subgroups, you can identify differences or similarities that might be masked in the overall analysis. This analysis is particularly important in studies involving interventions, where it is essential to understand how different groups respond to the treatment or control conditions.
10. Correlation Analysis
By calculating correlation coefficients, you can determine how closely two variables move together. This technique helps in identifying trends and associations, aiding in the understanding of underlying relationships and potential causal factors within your dataset.
- Exploring Relationships: Run correlation analyses to explore relationships between key variables. For example, examine how self-efficacy correlates with physical activity levels. This helps identify potential predictors or influences on the outcome variables. Correlation analysis measures the strength and direction of the relationship between two or more variables. It provides insights into how variables are related and whether changes in one variable are associated with changes in another. Common correlation coefficients include Pearson's r, Spearman's rho, and Kendall's tau. Correlation analysis is a fundamental tool for exploring relationships in the data and identifying potential causal factors.
11. Reporting and Interpretation
Reporting and interpretation involve summarizing and communicating your findings effectively. This includes presenting descriptive statistics, reliability measures, and correlations in a clear and concise manner. Accurate reporting ensures that insights are actionable and understandable, while thoughtful interpretation provides context and implications, guiding future research or decision-making.
- Preparing Reports: Prepare a detailed report summarizing your findings. Include tables and charts to present descriptive statistics, reliability analysis, subgroup comparisons, and correlation results. Interpret the results in the context of the assignment objectives, discussing the implications and any limitations. A well-prepared report presents the analysis and findings clearly and concisely. It should include an introduction, methodology, results, discussion, and conclusion. Use tables, graphs, and charts to illustrate key findings and enhance the readability of the report. Providing a thorough interpretation of the results, along with a discussion of their implications and limitations, is essential for a comprehensive and meaningful report.
- Writing Clearly: Clear and effective writing is essential for presenting your statistical findings in a way that is both understandable and impactful. Start by organizing your thoughts and structuring your report logically. Use straightforward language and define any technical terms to ensure clarity. Provide a clear introduction, detailed methods, and a concise summary of your results. When writing, focus on being precise and avoiding jargon that may confuse readers.
If you find yourself struggling with the writing process or need assistance in articulating complex statistical concepts, don't hesitate to seek help from statistical analysis assignment help services. These services offer expert guidance in drafting and refining your reports, ensuring that your findings are communicated clearly and professionally. Utilizing such support can enhance the quality of your work and ensure that your statistical analyses are presented in the best possible manner.
Conclusion
Solving your statistics assignment requires a methodical approach and a solid understanding of key techniques. By starting with thorough data preparation and exploring descriptive statistics, you lay a strong foundation for more advanced analyses. Calculating value-expectancies, performing reliability analysis, and transforming variables are crucial steps that enhance the depth and accuracy of your work. Additionally, conducting subgroup analyses and correlation studies helps you uncover meaningful relationships within your data. Finally, preparing a comprehensive report that clearly presents your findings and interpretations is essential. This systematic approach not only helps you solve your statistics assignment but also builds your confidence and proficiency in handling complex statistical tasks. Remember, each step is vital in ensuring the integrity and clarity of your analysis. With these techniques, you are well-equipped to tackle any statistics assignment that comes your way, leading to successful and insightful outcomes.