Exploring ANOVA: Understanding and Reporting Group Differences in Statistics Assignments
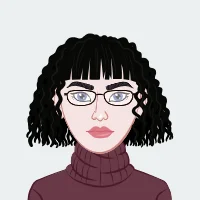
Analyzing group differences is a critical component in many statistical studies, and mastering this skill can significantly boost your academic performance. One of the most powerful tools for this purpose is the Analysis of Variance (ANOVA) test. Understanding how to conduct and interpret ANOVA can help you solve your statistics assignment with confidence and precision. This blog will provide a comprehensive guide to performing ANOVA tests, ensuring you can handle similar tasks with ease. By following the steps outlined here, you'll learn how to formulate hypotheses, run the test using statistical software, interpret the results, conduct post hoc analyses, and effectively report your findings. Whether you are comparing perceptions, measurements, or any other variables among multiple groups, mastering ANOVA is an essential skill that will help you solve your ANOVA assignment efficiently and accurately.
Step 1: Understanding ANOVA
The Analysis of Variance (ANOVA) is a statistical method used to compare the means of three or more groups to determine if there are any statistically significant differences between them. Unlike the t-test, which is used for comparing the means of two groups, ANOVA is specifically designed for scenarios involving multiple groups.
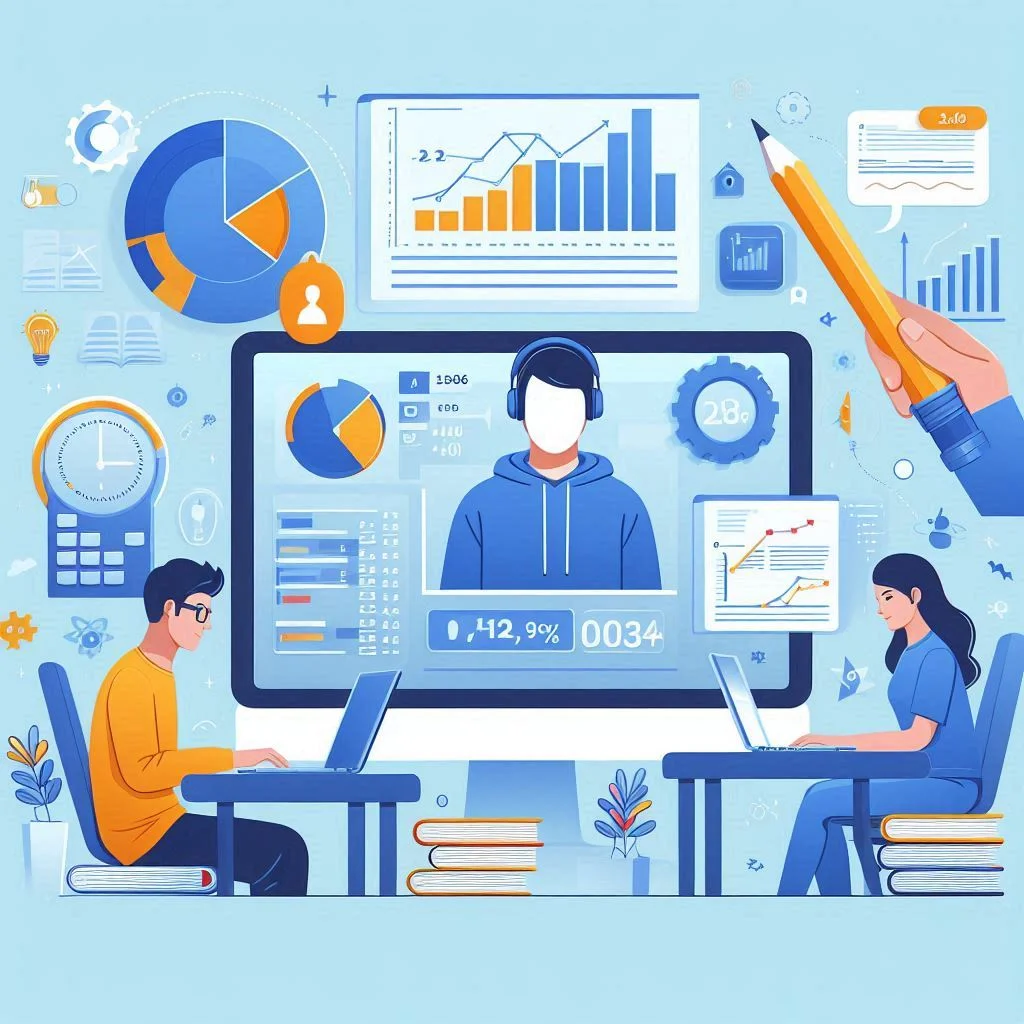
Key Concepts of ANOVA
1. Between-Group Variability:
- This measures the variation in the data due to the differences between the group means. It reflects how much the group means deviate from the overall mean of all groups combined.
2. Within-Group Variability:
- This measures the variation within each group. It shows how much the individual data points in each group deviate from their respective group mean.
3. F-Statistic:
- ANOVA uses the F-statistic to compare the between-group variability to the within-group variability. A higher F-statistic suggests a greater probability that the group means are significantly different.
4. P-Value:
- The p-value indicates the probability that the observed differences in means occurred by chance. A p-value less than the significance level (usually 0.05) suggests that there are significant differences between the groups.
By understanding these fundamental aspects of ANOVA, you can apply this statistical technique to a wide range of scenarios, enabling you to effectively solve your statistics assignment.
Step 2: Formulating Hypotheses
Formulating hypotheses is a crucial step in any statistical analysis, including ANOVA. Hypotheses provide a framework for testing and guide the interpretation of your results. In the context of ANOVA, you will typically formulate both a null hypothesis and an alternative hypothesis.
Null Hypothesis (H0)
The null hypothesis states that there are no differences in the means of the groups being compared. Essentially, it posits that any observed differences are due to random chance rather than a true effect. For an ANOVA test, the null hypothesis can be written as:
H0:μ1=μ2=μ3=…=μk
Where μ represents the mean of each group and k is the number of groups.
Example: If you are comparing the perception of bias levels among three different religious groups, your null hypothesis would be: H0:μ1=μ2=μ3
This means that the average perception of bias is the same across all three groups.
Alternative Hypothesis (H1)
The alternative hypothesis suggests that at least one group mean is different from the others. It does not specify which groups are different or how many groups differ, just that not all group means are equal. For an ANOVA test, the alternative hypothesis can be written as:
H1: At least one μi is different
Steps to Formulate Hypotheses
- Identify the Groups: Clearly define the groups you are comparing. For example, different religious groups, different treatment groups, or different time points.
- Determine the Dependent Variable: Identify the variable that you are measuring and comparing across groups. This is the variable whose means you will compare using ANOVA.
- State the Null Hypothesis (H0): Formulate the null hypothesis to state that all group means are equal.
- State the Alternative Hypothesis (H1): Formulate the alternative hypothesis to state that at least one group mean is different.
By correctly formulating your null and alternative hypotheses, you set the stage for a robust and meaningful ANOVA test, ensuring that you can effectively solve your statistics assignment and draw accurate conclusions from your data.
Step 3: Conducting the ANOVA Test
Conducting an ANOVA test involves several systematic steps to ensure accurate and reliable results. Here’s a detailed guide on how to perform the ANOVA test effectively.
Prepare Your Data
- Data Cleaning: Ensure your dataset is free from errors, missing values, and outliers. Clean data is crucial for accurate analysis.
- Data Coding: Properly code your categorical variables. For example, if you are comparing different groups, each group should be assigned a unique identifier.
- Check Assumptions: Verify that your data meets the assumptions of ANOVA, including normality, homogeneity of variances, and independence of observations.
Use Statistical Software
- Importing Data: Load your dataset into statistical software like JMP, SPSS, R, or Python. Most software programs have user-friendly interfaces for data import.
- Selecting Variables: Identify and select the dependent variable (the variable you are measuring) and the independent variable (the groups you are comparing).
- Navigating to ANOVA: Locate the ANOVA function within the software. This is often found under analysis or statistics menus.
Running the Test
- Set Up the Analysis: Specify the model by selecting the dependent variable and the independent variable(s). For example, in JMP, you might use the Fit Model platform.
- Execute the Test: Run the ANOVA test. The software will generate output that includes the F-statistic, p-value, and other relevant statistics.
- Review Output: Carefully examine the output to understand the results. Key outputs include:
- F-Statistic: Indicates the ratio of between-group variance to within-group variance.
- P-Value: Determines the significance of the results. A p-value less than 0.05 typically indicates significant differences between groups.
- Means and Standard Deviations: Provides descriptive statistics for each group.
By following these steps, you can accurately conduct an ANOVA test and obtain meaningful insights from your data. This structured approach will help you solve your statistics assignment efficiently, ensuring that you understand and can explain the differences between groups in your study.
Step 4: Interpreting ANOVA Results
Interpreting the results of an ANOVA test is crucial for understanding whether there are significant differences between the groups you are studying. Here’s how to effectively interpret ANOVA results to solve your statistics assignment:
Examining the ANOVA Table
- Sum of Squares (S):
- Between-Groups (SSB): Measures the variation due to the differences between the group means.
- Within-Groups (SSW): Measures the variation within each group.
- Total (SST): The sum of SSB and SSW.
- Degrees of Freedom (df):
- Between-Groups (dfB): Calculated as the number of groups minus one (k-1, where k is the number of groups).
- Within-Groups (dfW): Calculated as the total number of observations minus the number of groups (N-k, where N is the total number of observations).
- Mean Squares (MS):
- Between-Groups (MSB): Calculated as SSB divided by dfB.
- Within-Groups (MSW): Calculated as SSW divided by dfW.
- F-Statistic: Calculated as MSB divided by MSW. This statistic is used to determine whether the group means are significantly different.
- P-Value: Indicates the probability that the observed differences in group means occurred by chance. If the p-value is less than the significance level (typically 0.05), the null hypothesis is rejected, indicating that there are significant differences between the group means.
Steps to Interpret the ANOVA Output
1. Identify the P-Value:
- Locate the p-value in the ANOVA table. Compare it to your significance level (α, usually set at 0.05).
- If the p-value is less than α, reject the null hypothesis.
- If the p-value is greater than α, fail to reject the null hypothesis. This suggests that there are no significant differences between the group means.
2. Analyze the F-Statistic:
- A large F-statistic indicates that the between-group variability is much greater than the within-group variability, suggesting significant differences between the group means.
- A small F-statistic suggests that the group means are not significantly different from each other.
3. Post Hoc Analysis (if applicable):
- If the ANOVA indicates significant differences, conduct post hoc tests to determine which specific groups differ. Common post hoc tests include Tukey’s HSD, Bonferroni, and Scheffé.
- Interpret the post hoc results to identify which pairs of group means are significantly different.
4. Reporting the Results:
- Clearly report the F-statistic, degrees of freedom, and p-value. For example: "An ANOVA test revealed significant differences between the groups (F(2, 57) = 5.67, p = 0.004)."
- Summarize the findings from the post hoc tests, highlighting which groups showed significant differences.
By carefully interpreting the ANOVA results, you can draw meaningful conclusions about your data, which is essential to solve your statistics assignment effectively. This step-by-step approach ensures that you understand the significance of your findings and can communicate them clearly in your report.
Step 5: Conducting Post Hoc Analysis
Once you have determined that there are significant differences between groups using an ANOVA test, the next step is to identify which specific groups differ from each other. This is done through post hoc analysis. Post hoc tests are conducted after an ANOVA to make pairwise comparisons between group means while controlling for the overall type I error rate.
Importance of Post Hoc Analysis
Post hoc analysis is essential because while ANOVA tells you that at least one group mean is different, it does not specify which groups differ. Post hoc tests help pinpoint these differences, providing a more detailed understanding of your data.
Steps for Conducting Post Hoc Analysis
- Choose the Appropriate Test: Select a post hoc test based on the specifics of your data and the number of comparisons. Tukey’s HSD and Bonferroni are good general choices, while Scheffé’s test is useful for many comparisons.
- Run the Test Using Statistical Software: Use statistical software like JMP, SPSS, or R to perform the post hoc analysis. Most software packages offer these tests as part of their ANOVA output options.
- Interpret the Results: Review the output matrix from the post hoc test. Identify which pairs of group means are significantly different. Note the p-values and confidence intervals provided for each comparison.
- Report the Findings: Clearly present the results of the post hoc analysis in your report. Include which groups were compared, the differences found, and their statistical significance. Example: “Post hoc analysis using Tukey’s HSD indicated that Group A differs significantly from Group B (p < 0.01) but not from Group C (p = 0.15).”
By thoroughly conducting post hoc analysis, you can provide a detailed and accurate interpretation of your ANOVA results, enabling you to solve your statistics assignment with a deeper understanding of the differences between your groups.
Step 6: Reporting Results
Properly reporting the results of your ANOVA test is crucial for conveying your findings clearly and accurately. Here’s how to structure your results section to ensure clarity and precision:
1. Restate the Hypotheses
Begin by restating your null and alternative hypotheses to remind your readers of the context of your analysis.
2. Present the ANOVA Results
Summarize the key statistics from your ANOVA test:
- F-Value: The test statistic from the ANOVA test, indicating the ratio of between-group variance to within-group variance.
- Degrees of Freedom (df): The df for the numerator (between groups) and the denominator (within groups).
- P-Value: The probability that the observed differences between group means occurred by chance.
3. Interpret the Results
Explain what the results mean in the context of your study:
- If the p-value is less than the significance level (usually 0.05), indicate that you reject the null hypothesis and conclude that there are significant differences between the groups.
- If the p-value is greater than the significance level, indicate that you fail to reject the null hypothesis and conclude that there are no significant differences between the groups.
4. Post Hoc Analysis
If you found significant differences, report the results of your post hoc analysis:
- Mention the post hoc test used (e.g., Tukey's HSD, Bonferroni).
5. Include Descriptive Statistics
Provide descriptive statistics for each group to give a complete picture:
- Report the mean and standard deviation for each group.
6. Graphical Representation
Include a graph to visually represent the differences between groups:
- Use bar charts, line graphs, or box plots to show the mean scores and variability within each group.
- Make sure the graph is clearly labeled and includes error bars to indicate variability.
By following these steps, you can effectively report your ANOVA results, making your analysis clear and comprehensible to your audience. This structured approach will help you solve your statistics assignment and present your findings professionally.
Conclusion
Mastering the ANOVA test is a valuable skill that will enhance your ability to analyze and compare group differences in various statistical assignments. By following the comprehensive guide provided in this blog, you will be well-equipped to solve your statistics assignment with greater accuracy and confidence. From formulating hypotheses and conducting the test to interpreting results and performing post hoc analyses, each step is crucial in delivering precise and meaningful conclusions. Additionally, learning how to effectively report and visualize your findings will further solidify your understanding and communication of statistical data. Remember, practice and familiarity with statistical software will improve your proficiency over time. By incorporating these techniques, you can efficiently solve your statistics assignment, ensuring academic success and a deeper understanding of statistical analysis.