The Role of Fixed and Random Factors in Effective Statistical Research Design
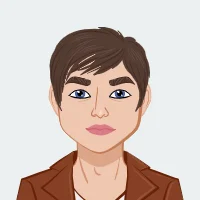
Designing a research study requires careful consideration of various factors that influence your data analysis and results. Among these, fixed and random factors play a crucial role in determining how you approach your study design and statistical analysis. Whether you're working on a research project or trying to solve your statistics assignment, understanding these concepts is essential for producing accurate and meaningful results. Fixed factors refer to variables with specific, predetermined levels that are of particular interest, while random factors involve levels that are randomly selected from a larger population, allowing for broader generalizations. This blog explores these concepts in depth, providing you with the knowledge needed to apply them effectively in your research.
Understanding Fixed and Random Factors
In statistical study design, factors are the variables that researchers manipulate or observe to determine their effect on the dependent variable. These factors can be classified into two categories: fixed and random.
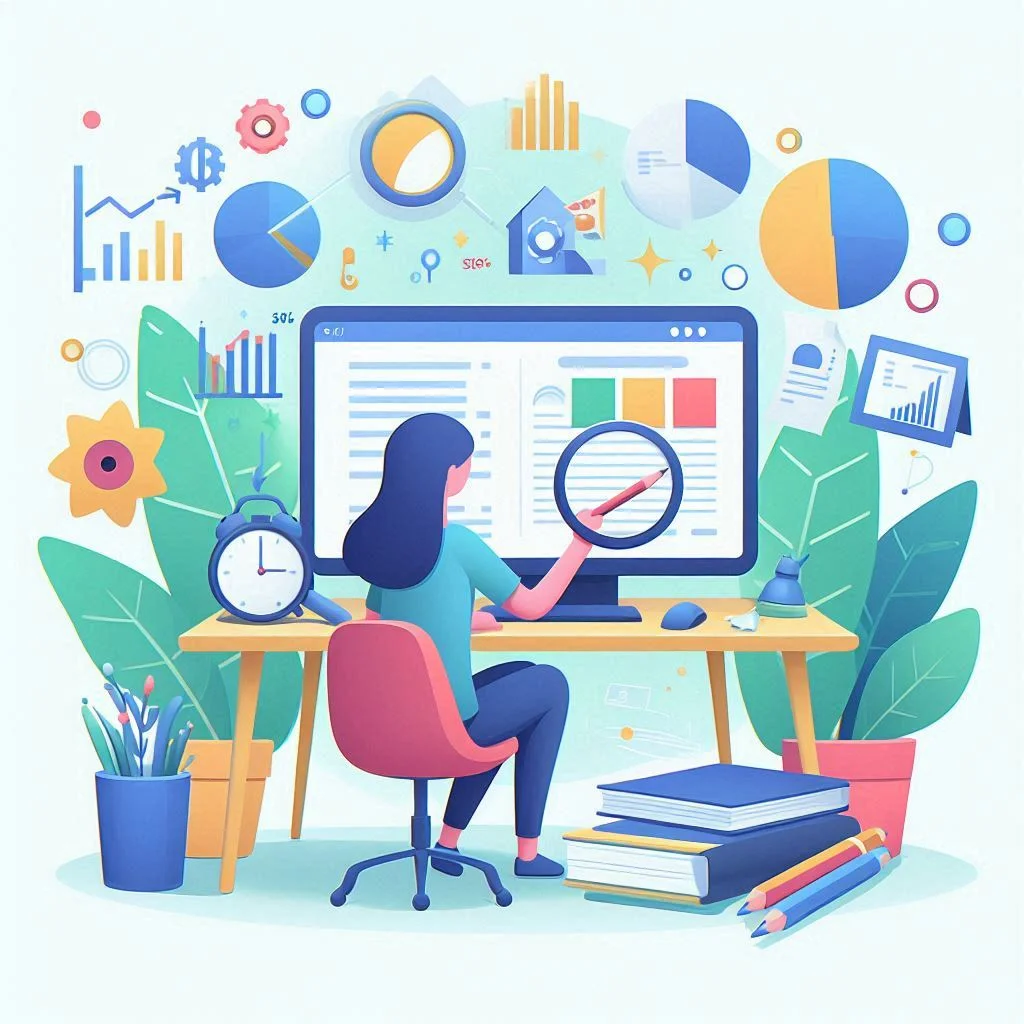
- Fixed Factors: A fixed factor is one where the levels of the factor are specifically chosen and of interest in the study. The researcher is typically interested in comparing these levels directly and the conclusions drawn from the analysis are only applicable to the specific levels included in the study.
- Random Factors: A random factor, on the other hand, includes levels that are randomly selected from a larger population. The primary interest is in generalizing the results beyond the specific levels studied, to the broader population from which they were drawn.
When to Use Fixed or Random Factors
The choice between fixed and random factors depends on the goals of your study.
- Fixed Factors: Use fixed factors when you are interested in understanding the effects of specific, predetermined levels of a variable. For example, if you want to compare the efficacy of three different teaching methods, and you are only interested in those three methods, the teaching method would be considered a fixed factor.
- Random Factors: Random factors are used when the levels of a variable are randomly sampled from a larger population, and you want to generalize the results to the entire population. For instance, if you were studying the effect of different schools on student performance and the schools were randomly selected from all schools in a region, then the school variable could be considered a random factor.
Designing a Study with Fixed Factors
When designing a study with fixed factors, it’s essential to clearly define the levels of each factor and ensure that these levels are consistently applied throughout the research. The analysis should focus on comparing these specific levels to determine if there is a significant effect.
Example: Suppose you are studying the effect of different training programs on employee productivity. You have three distinct programs that you want to compare. Since you are specifically interested in these three programs, they are considered fixed factors.
1. Step 1: Define the Factor Levels
In this step, clearly identify and define the specific levels of the fixed factor that will be included in your study. For instance, if you are examining the effects of different training programs, specify each program as a distinct level. Ensure these levels are relevant to your research question and are consistently applied throughout the study. This precise definition is crucial for accurately comparing the outcomes associated with each level and drawing valid conclusions.
2. Step 2: Ensure Consistency in Application
To maintain the integrity of your study with fixed factors, apply each level of the fixed factor consistently across all experimental conditions. Ensure that every participant or observation is exposed to only one level of the fixed factor, avoiding any overlap or variation. Consistency in application is crucial for accurately assessing the effects of the fixed factor and ensuring that any observed differences are due to the factor itself, not variations in how it was applied.
3. Step 3: Analyze the Data
Once the study is designed and data collected, it’s crucial to analyze the data using appropriate statistical methods. For fixed factors, conduct an ANOVA to assess whether there are significant differences between the levels. Ensure that the analysis accounts for any potential interactions between factors. Properly interpreting these results will help determine the impact of each fixed factor on the dependent variable and provide insights into the effectiveness of the study conditions.
4. Step 4: Interpret the Results
Interpreting results from a study with fixed factors involves comparing the outcomes across different levels of the factor to determine if there are significant differences. Analyze the statistical outputs, such as p-values and confidence intervals, to assess the significance of the effects. Ensure that the conclusions drawn are supported by the data and reflect the practical implications of the findings. Clear interpretation helps in understanding the impact of each fixed factor on the dependent variable.
Designing a Study with Random Factors
Designing a study with random factors requires a different approach. Here, the focus is on ensuring that the levels of the factor are randomly selected and represent a broader population.
Example: Imagine you are researching the impact of different classroom environments on student engagement. If you randomly select classrooms from a large number of schools, the classroom variable is a random factor.
1. Step 1: Random Selection of Factor Levels
When designing a study with random factors, start by randomly selecting the levels from a larger population to ensure they are representative. This random selection helps mitigate biases and allows your findings to generalize to a broader context. Use random sampling techniques to choose these levels, ensuring that each level has an equal chance of being included. This approach enhances the validity of your study and supports more robust and generalizable conclusions.
2. Step 2: Incorporate Randomness into the Study Design
To incorporate randomness, ensure that the selection of levels for random factors is truly random and representative of the broader population. Implement random sampling techniques to avoid bias and ensure that your sample reflects the diversity of the population. Integrate these random levels into your study design and use statistical methods, such as mixed-effects models, to account for the variability introduced by these random factors. This enhances the generalizability and validity of your findings.
3. Step 3: Use Mixed-Effects Models
When working with random factors, apply mixed-effects models to account for both fixed and random effects. These models help analyze data by incorporating variability from random factors, such as differences across schools or subjects. Use software tools to fit the model, estimate variance components, and assess the impact of both fixed and random factors. Mixed-effects models provide a comprehensive analysis, allowing for more accurate interpretation of how random factors influence the overall outcomes.
4. Step 4: Generalize the Findings
When generalizing findings from a study with random factors, extend the results to the broader population from which the random levels were sampled. Assess how the random factor contributes to overall variability and ensure that your conclusions are applicable beyond the specific sample. Consider the representativeness of your random levels and the external validity of your results. Proper generalization provides valuable insights that can be applied to similar contexts or populations.
Formulating Hypotheses for Fixed and Random Factors
One of the key steps in any statistical analysis is formulating the null and alternative hypotheses. These hypotheses will differ depending on whether you are dealing with fixed or random factors.
- Fixed Factors: The null hypothesis typically states that there is no effect of the fixed factor on the dependent variable. For example, if you are comparing three teaching methods, the null hypothesis would be that all methods result in the same average outcome.
- Null Hypothesis (H₀): μ₁ = μ₂ = μ₃ (where μ represents the mean outcome for each teaching method).
- Random Factors: For random factors, the null hypothesis often states that the variance components associated with the random factor are zero, meaning that the levels of the random factor do not contribute to the variability in the outcome.
- Null Hypothesis (H₀): σ²₀ = 0 (where σ²₀ represents the variance component of the random factor).
Practical Applications and Considerations
When approaching assignments or research projects involving fixed and random factors, it’s important to consider the practical implications of your study design.
- Sample Size Considerations: Ensure that you have an adequate sample size to detect effects, especially when dealing with random factors, as they require larger samples to accurately estimate variance components.
- Data Collection: Pay close attention to how data is collected, ensuring that the random factors are truly random and that fixed factors are applied consistently.
- Software and Tools: Utilize statistical software like R, SPSS, or SAS that offer advanced capabilities for analyzing data with both fixed and random factors. Mixed-effects models are particularly useful for these types of analyses.
- Interpreting Results: Be cautious when interpreting results, especially with random factors, as the conclusions may extend beyond the specific data collected. Always consider the broader context of the findings.
- Reporting and Documentation: Clearly document your study design, including how factors were classified as fixed or random, and ensure transparency in how the data was analyzed. This is crucial for the reproducibility and validity of your research.
Common Challenges and Solutions
When working on statistics assignments that involve mixed-effects models, students often face challenges like misclassifying factors, inadequate sample sizes, and complexity in data analysis. Addressing these issues requires careful study design, appropriate statistical methods, and sometimes, seeking expert assistance with mixed-effects models assignments to ensure accurate and meaningful results.
Challenge: Misclassification of Factors
Misclassifying factors as fixed or random is a common issue in research design, leading to incorrect data analysis and misleading conclusions. Fixed factors should be specifically chosen and relevant to the study, while random factors are randomly selected and represent a broader population. Misclassification often occurs when researchers are unclear about the nature of their variables or lack familiarity with these concepts.
Solution: To avoid misclassification, it's crucial to clearly define your study objectives and consult relevant literature or experts in the field. Take the time to understand whether your factors should be treated as fixed or random based on the research question and the generalizability of the results. If in doubt, consider using mixed-effects models that can accommodate both fixed and random factors, providing more accurate analysis.
Challenge: Inadequate Sample Size for Random Factors
One common challenge when working with random factors in research design is having an inadequate sample size. Random factors require a sufficient number of observations to accurately estimate variance components and ensure that the results are reliable and generalizable. Small sample sizes can lead to biased estimates and reduced statistical power, making it difficult to detect true effects.
Solution: To address this issue, conduct a power analysis before data collection to determine the required sample size for your study. Power analysis helps you estimate the minimum sample size needed to achieve reliable results, considering the effect size, significance level, and desired power. Additionally, consider increasing the sample size if feasible, or utilize statistical techniques that can handle smaller sample sizes, such as bootstrapping or Bayesian methods.
Challenge: Complexity in Data Analysis
Data analysis involving fixed and random factors can be complex due to the need for advanced statistical models and the potential for intricate interactions between factors. Understanding how to appropriately apply mixed-effects models or hierarchical models can be daunting. Additionally, interpreting the results requires a strong grasp of statistical theory and the ability to manage multiple layers of variability.
Solution: To navigate this complexity, it is essential to utilize statistical software that can handle mixed-effects models, such as R, SPSS, or SAS. These tools provide built-in functions for analyzing data with both fixed and random effects, simplifying the process. Additionally, investing time in learning about these models through tutorials or seeking help from a statistician can greatly enhance your ability to perform accurate and efficient data analysis.
Challenge: Interpretation of Interaction Effects
Interpreting interaction effects between fixed and random factors can be challenging because these interactions often involve complex relationships between variables. In studies with multiple factors, the effects of one factor may depend on the levels of another, complicating the interpretation of results. For instance, when examining how different instructional methods impact performance across various grade levels, the interaction effect can reveal if certain methods work better in specific grades.
Solution: To address this challenge, use statistical software to perform detailed analyses of interaction effects. Visualize the interactions using graphs to understand how factors influence each other. Additionally, consult with statistical guidelines or seek expert help with mixed-effects models assignments if needed to ensure accurate interpretation. Properly addressing interaction effects will enhance the clarity and validity of your findings.
Conclusion
Successfully incorporating fixed and random factors into your research design is key to producing reliable and generalizable results. Whether you’re dealing with specific variables of interest or working with randomly selected samples, understanding these concepts will enhance the quality of your analysis. By correctly identifying and applying fixed and random factors, you can ensure that your study design is robust and your conclusions are sound. This knowledge is not only crucial for academic research but also essential when you need to solve your statistics assignment. As you continue to work on similar projects, remember that the clarity and accuracy of your study design significantly impact the outcomes of your research. With the insights gained from this blog, you’re better equipped to tackle complex statistical challenges and solve your statistics assignment effectively.