Applying Statistical Methods for Quality Control: Techniques and Applications
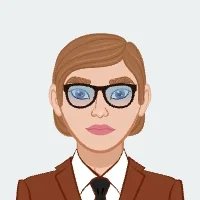
In the realm of quality control, statistical methods play a pivotal role in ensuring processes and products meet rigorous standards. Understanding these methods is crucial for both academic and professional success. Quality control involves monitoring processes, identifying variations, and making data-driven decisions to maintain high standards. Whether you're tackling a complex problem in a course or working on a real-world project, mastering these techniques will enhance your ability to solve your statistics assignment effectively. This blog explores essential statistical methods for quality control, including Statistical Process Control (SPC), process capability analysis, and Design of Experiments (DOE). By applying these techniques, you can improve process stability, analyze performance, and drive continuous improvements. Dive into the details of these methods and learn how to utilize them to solve your statistics assignment and address quality challenges in various fields.
1. Introduction to Quality Control in Statistics
Quality control (QC) is the process of ensuring that a product or service meets certain standards of quality. It involves using statistical methods to monitor and control processes, ensuring they operate within specified limits and produce consistent results. The primary goal of QC is to identify and address any deviations from quality standards before they affect the final product or service.
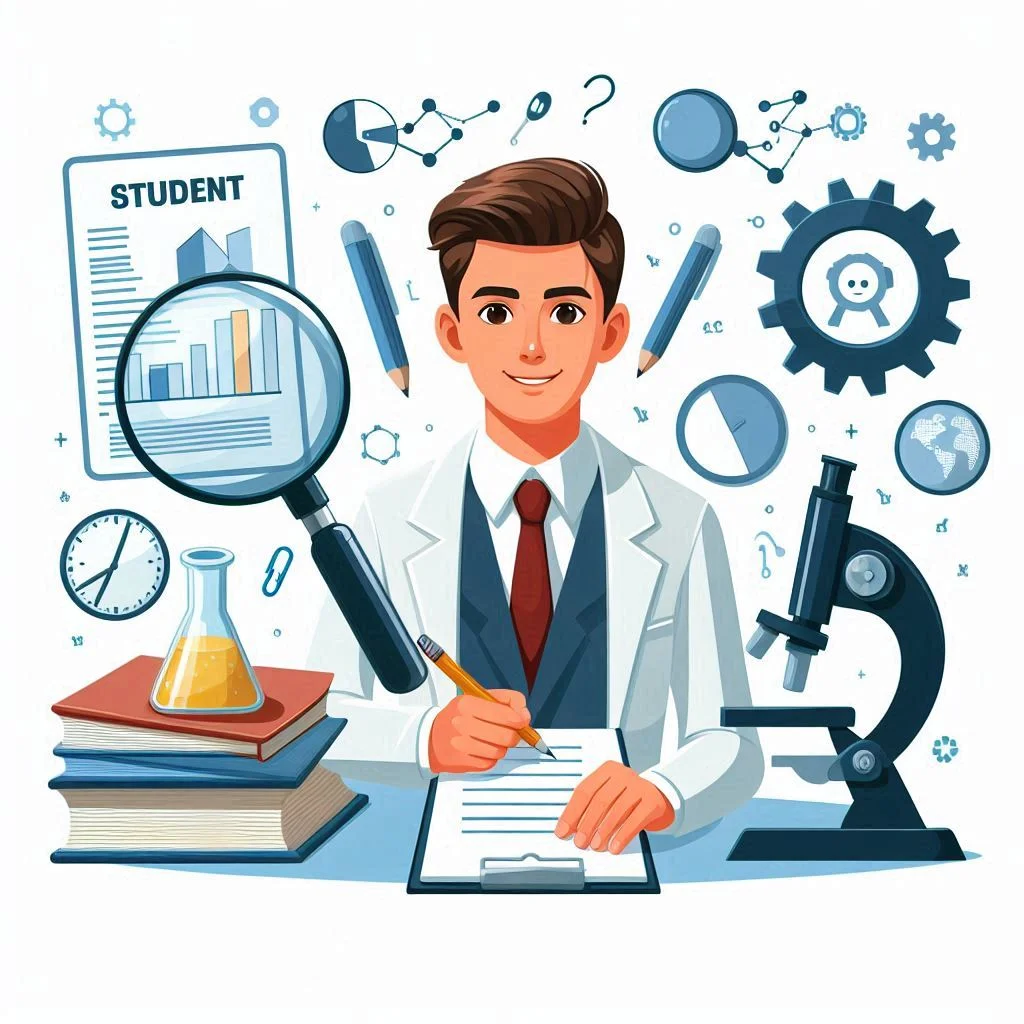
Key aspects of quality control include:
- Understanding Variability: All processes exhibit variability, and QC aims to distinguish between normal (common cause) variation and abnormal (special cause) variation.
- Process Stability: Ensuring that a process remains stable over time, producing consistent results.
- Continuous Improvement: Using data to identify areas for improvement and implement changes that enhance quality.
2. Key Statistical Methods for Quality Control
Key statistical methods for quality control help monitor and enhance process performance. Techniques like Statistical Process Control (SPC) use control charts to track process variations, while Process Capability Analysis evaluates how well processes meet specifications. Design of Experiments (DOE) systematically explores factors to optimize processes, ensuring consistent quality and reliability across various applications.
Statistical Process Control (SPC)
Statistical Process Control (SPC) helps in understanding process behavior, detecting problems early, and ensuring that processes operate within acceptable limits.
Components of SPC:
- Control Charts: These are graphical tools used to monitor the variation in a process over time. They help in identifying trends, shifts, or any unusual patterns. Common types of control charts include:
- X-bar Chart: Monitors the average of a process over time.
- R-chart: Tracks the range within a sample. It is used in conjunction with the X-bar chart to monitor process variability.
- P-chart: Used for proportion data, such as the percentage of defective items in a sample.
How to Use Control Charts:
- Data Collection: Collect data from the process at regular intervals.
- Plot Data: Plot the data on the control chart to visualize the process behavior.
- Interpret Results: Analyze the chart to determine if the process is in control or if there are any signals of instability.
Control Charts for Attributes and Variables
Control charts can be categorized based on the type of data they handle:
- Control Charts for Variables: Used when data is measured on a continuous scale (e.g., weight, temperature). Examples include X-bar and R-charts.
- Control Charts for Attributes: Used when data is categorical or counts of occurrences (e.g., number of defects). Examples include P-charts and C-charts.
Process Capability Analysis
Process capability analysis evaluates how well a process meets specified requirements. It provides insights into the process’s ability to produce products within tolerance limits.
Key Metrics:
- Cp (Process Capability Index): Measures the potential capability of a process by comparing the spread of the process to the specification limits. It assumes that the process is centered within the limits.
- Cpk (Process Capability Performance Index): Takes into account the process mean and how well it is centered within the specification limits. It is a more accurate reflection of process capability.
- Pp and Ppk: Similar to Cp and Cpk but use long-term data to assess process capability over time. Pp and Ppk provide insights into how well the process performs in real-world conditions.
How to Conduct Process Capability Analysis:
- Collect Data: Gather data from the process.
- Calculate Metrics: Use statistical formulas to calculate Cp, Cpk, Pp, and Ppk.
- Interpret Results: Compare the calculated values to industry standards to determine if the process is capable.
Design of Experiments (DOE)
Design of Experiments (DOE) is a systematic approach used to understand the relationship between factors affecting a process and the output of that process. DOE helps in optimizing processes by systematically varying factors and observing their effects.
Key Aspects of DOE:
- Factorial Designs: Explore the effects of multiple factors and their interactions on the output. Full factorial designs examine all possible combinations of factors, while fractional factorial designs focus on a subset to reduce complexity.
- Response Surface Methodology (RSM): Used to explore the relationships between factors and responses and to optimize process conditions.
- Randomization and Replication: Ensuring that experiments are randomized to avoid bias and replicated to verify results.
How to Use DOE:
- Define Objectives: Clearly state the goals of the experiment.
- Design the Experiment: Select factors, levels, and experimental runs.
- Conduct the Experiment: Perform the experiment as per the design.
- Analyze Data: Use statistical methods to analyze the results and determine the effects of factors.
3. Practical Applications of Quality Control Techniques
Quality control techniques have broad applications across various industries. In manufacturing, they help ensure product consistency, while in service industries, they improve service delivery and customer satisfaction. Healthcare uses these methods to maintain high standards in patient care, and research and development leverage them to optimize processes and product formulations.
Manufacturing
In manufacturing, quality control techniques are used to monitor production processes, reduce defects, and maintain consistent quality. Control charts help in identifying deviations from the desired quality standards, while process capability analysis ensures that the manufacturing process meets customer requirements.
Example: A company manufacturing electronic components uses X-bar and R-charts to monitor the dimensions of components. By analyzing the control charts, the company can detect deviations and make adjustments to maintain product quality.
Service Industries
In service industries, quality control methods help in managing service delivery processes and ensuring customer satisfaction. Techniques such as control charts and process capability analysis can be applied to service metrics like response time and customer satisfaction scores.
Example: A call center uses P-charts to monitor the proportion of calls resolved within a specified time frame. By analyzing the data, the call center can identify areas for improvement and enhance service quality.
Healthcare
Quality control methods are employed in healthcare to ensure the accuracy of medical tests, patient care processes, and overall service quality. Techniques such as control charts and process capability analysis help in maintaining high standards in healthcare delivery.
Example: A hospital uses control charts to monitor the accuracy of diagnostic tests. By analyzing the charts, the hospital can identify any issues with test performance and take corrective actions to ensure reliable results.
Research and Development
In research and development, DOE is used to design experiments that optimize product formulations, processes, and innovations. DOE helps in systematically exploring factors and their effects to achieve desired outcomes.
Example: A pharmaceutical company uses DOE to optimize the formulation of a new drug. By systematically varying factors such as ingredient concentrations and processing conditions, the company can identify the optimal formulation for the drug.
4. Tips for Effective Data Analysis in Quality Control
To excel in data analysis for quality control, start with clear objectives and choose the appropriate statistical tools. Ensure data accuracy, interpret results carefully, and document your analysis comprehensively. Seeking feedback can also provide valuable insights. These steps will enhance the effectiveness and reliability of your quality control analysis.
Understand the Process
Before applying quality control methods, gain a thorough understanding of the process or system being analyzed. This includes knowing the input factors, output variables, and potential sources of variation. A clear understanding of the process helps in selecting appropriate tools and interpreting results accurately.
Select Appropriate Tools
Choose the right statistical tools and techniques based on the type of data and the specific objectives of the analysis. For example, use control charts for monitoring process variation and process capability analysis for assessing process performance.
Collect Accurate Data
Ensure that data collection methods are reliable and that the data accurately reflects the process being studied. Accurate data is essential for meaningful analysis and effective quality control.
Interpret Results Correctly
Analyze the results of control charts, capability indices, and experiments with a clear understanding of what they indicate about the process. Avoid misinterpretation by referring to industry standards and seeking guidance if needed.
Implement Improvements
Quality control is not just about monitoring but also about using data to drive continuous improvement and enhance process performance.
5. Common Challenges and How to Overcome Them
Common challenges in quality control data analysis include data quality issues, misinterpretation of results, and resistance to change. To overcome these, ensure robust data collection, accurately interpret statistical results, and communicate effectively with stakeholders. Addressing variability and designing experiments carefully will help mitigate these challenges and improve the reliability and effectiveness of your quality control efforts.
Data Quality Issues
To overcome this challenge, ensure that data collection methods are robust, and address any issues with data accuracy and completeness.
Misinterpretation of Control Charts
Misreading control charts can result in incorrect conclusions about process stability. Seek training or consult experts if unsure about interpreting charts. Understanding the fundamentals of control charts and their interpretation is crucial for effective quality control.
Overlooking Variability
Ignoring variability in the process can lead to ineffective quality control measures. Always consider both common cause and special cause variations. Identifying the sources of variation and addressing them appropriately is key to maintaining process stability.
Inadequate Experiment Design
Poorly designed experiments can yield unreliable results and lead to incorrect conclusions. Ensure that experiments are well-planned, follow best practices in DOE, and consider factors such as randomization and replication.
Resistance to Change
Implementing changes based on statistical analysis may face resistance from stakeholders. Communicate the benefits of improvements clearly and involve stakeholders in the process. Demonstrating the value of data-driven decisions can help in overcoming resistance.
6. Strategies for Effective Data Analysis in Statistics Assignments
For effective data analysis in applied statistics assignments, focus on defining clear objectives and selecting suitable analytical methods. Ensure data accuracy through careful collection and preprocessing. Interpret results within the context of your assignment, and thoroughly document your process. Seek feedback to refine your approach and validate your findings. These strategies will enhance the quality and reliability of your analysis.
- Clearly Define Objectives: Begin by understanding the specific goals of your analysis. Clearly defined objectives guide your choice of statistical methods and ensure that your analysis addresses the key questions of your assignment.
- Choose Appropriate Statistical Tools: Select the right statistical methods based on the type of data and the analysis requirements. For instance, use control charts for monitoring process variations and process capability analysis for assessing performance against specifications.
- Ensure Data Accuracy: Accurate data is crucial for reliable analysis. Verify that your data collection methods are robust, and that the data accurately reflects the process or phenomenon being studied.
- Interpret Results Wisely: Carefully analyze the results of your statistical tests and control charts. Look for patterns, trends, or anomalies that can provide insights into the process or quality issues.
- Document Your Analysis: Maintain thorough documentation of your data, methods, and findings. Clear documentation supports reproducibility and helps in communicating your results effectively.
- Seek Feedback: Obtaining feedback is crucial for refining your data analysis and ensuring accuracy. Engage with peers, instructors, or applied statistics assignment help services to gain valuable insights and perspectives. These external inputs can help identify areas for improvement, validate your approach, and enhance the overall quality of your work, leading to more effective and reliable analysis.
These tips will help you tackle quality control assignments with greater confidence and precision, ensuring that your analyses are both accurate and insightful.
Conclusion
Mastering quality control with statistical methods involves understanding and applying various techniques to monitor and improve processes effectively. By leveraging tools like Statistical Process Control (SPC), control charts, process capability analysis, and Design of Experiments (DOE), students and professionals can ensure high-quality outcomes and drive continuous improvement in their respective fields. Quality control is not just about applying statistical methods; it is about using data to make informed decisions, identify areas for improvement, and implement changes that enhance quality. Whether you are tackling assignments or applying these methods in real-world scenarios, a solid grasp of these concepts will equip you to handle quality control challenges effectively. Embrace these techniques, apply them thoughtfully, and contribute to maintaining high standards of quality in your endeavors.