Efficient Techniques for Descriptive Statistics and Correlation Analysis in Statistics Assignments
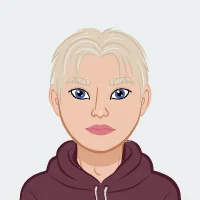
Statistics assignments often present a unique set of challenges that require a strategic approach to tackle effectively. Whether you are asked to calculate descriptive statistics such as the mean and standard deviation or perform correlation analysis, understanding the steps involved is crucial. This blog will walk you through the process of how to solve your statistics assignment efficiently. By breaking down the assignment into manageable tasks, you'll learn how to prepare your data, use the right tools, and interpret your findings accurately. Whether you're new to statistics or looking to improve your skills, these techniques will help you approach your assignments with confidence. With a structured plan and a clear understanding of the necessary calculations, you can solve your statistics assignment successfully and achieve the results you desire.
Step 1: Understand the Assignment Requirements
The first step in solving your statistics assignment is to thoroughly understand what is being asked of you. This may seem obvious, but it's a critical part of the process that sets the stage for the entire assignment. Here’s how you can effectively grasp the requirements:
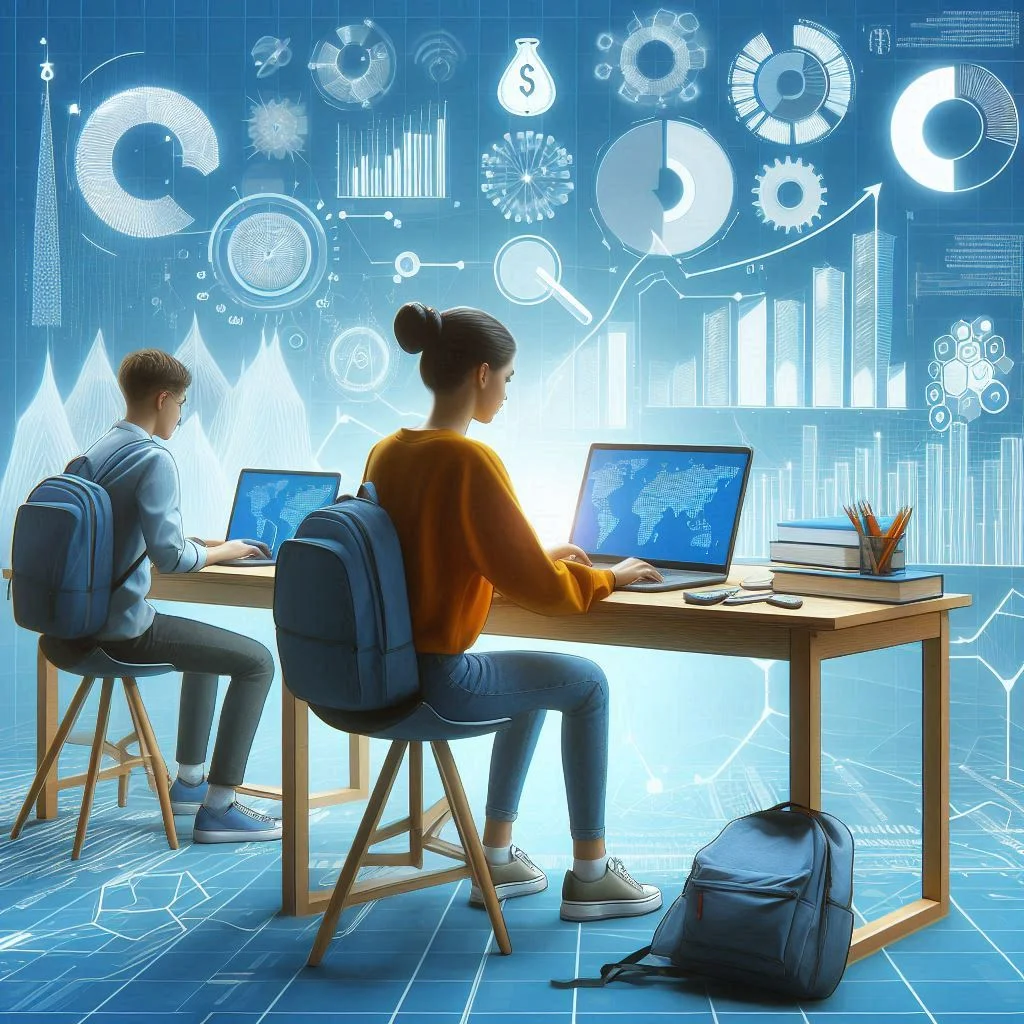
Read the Instructions Carefully
Carefully read through the assignment prompt. Identify key tasks and objectives. Look for specific instructions on the type of analysis required, such as calculating the mean, standard deviation, or performing correlation analysis.
Identify the Key Variables
Determine which variables or constructs are involved in the assignment. For example, you might need to analyze variables such as trust, loyalty, and overall value. Knowing which variables to focus on will guide your data preparation and analysis steps.
Clarify the Goals
Understand the goals of the assignment. Are you expected to summarize data using descriptive statistics? Are you looking to explore relationships between variables through correlation analysis? Having a clear picture of the goals will help you stay focused and organized.
By taking the time to understand the assignment requirements fully, you lay the groundwork for a successful analysis. This initial step ensures that you are clear on what needs to be done and helps you approach the assignment methodically, increasing your chances of solving your statistics assignment effectively.
Step 2: Prepare Your Tools
Having the right tools is essential for efficiently solving your statistics assignment. Depending on the complexity of your assignment and your personal preference, you might choose from a range of software and tools that facilitate data analysis and statistical calculations. Here are some commonly used tools:
Spreadsheet Software: Excel or Google Sheets
Spreadsheet software like Excel and Google Sheets is user-friendly and widely accessible. These tools are ideal for basic statistical analyses and are often sufficient for most student assignments. They come equipped with built-in functions for calculating means, standard deviations, and correlations.
- Excel: Known for its powerful data analysis capabilities, Excel offers various statistical functions and the Data Analysis Toolpak add-in, which simplifies more advanced calculations.
- Google Sheets: Similar to Excel, Google Sheets is a cloud-based alternative that allows for easy sharing and collaboration. It also supports essential statistical functions and offers add-ons for enhanced capabilities.
Statistical Software: SPSS, R, SAS
For more advanced or complex statistical analyses, dedicated statistical software can be invaluable. These tools offer extensive functionality and are designed specifically for data analysis.
- SPSS: A user-friendly interface makes SPSS popular among students and researchers. It provides comprehensive options for statistical analysis and is particularly well-suited for handling large datasets.
- R: An open-source programming language, R is highly flexible and powerful for statistical computing and graphics. It requires some programming knowledge but offers vast libraries and community support.
- SAS: Known for its robustness and ability to handle large datasets, SAS is a powerful tool for advanced statistical analysis. It is widely used in industry and academia for its comprehensive analytics capabilities.
Choosing the Right Tool
Selecting the appropriate tool depends on the assignment's requirements and your comfort level with the software. For simpler tasks, spreadsheet software like Excel or Google Sheets may be sufficient. For more complex analyses or larger datasets, consider using SPSS, R, SAS, or Python. Familiarize yourself with the tools beforehand to ensure you can efficiently navigate and utilize their features.
Step 3: Load and Explore the Dataset
Loading and exploring your dataset is a crucial initial step in any statistics assignment. This process not only familiarizes you with the data but also helps you identify potential challenges and insights that will guide your analysis.
Understanding Dataset Structure
Begin by loading your dataset into your chosen statistical tool or software. Take a moment to review the dataset's structure, including the number of variables (columns) and observations (rows). Understanding these basics allows you to plan your analysis effectively.
Exploring Variables
Next, explore the variables within the dataset. Identify the types of data each variable contains—whether numerical (quantitative) or categorical (qualitative). This exploration helps you determine which statistical methods and visualizations are appropriate for your analysis.
Checking for Missing Data
Check for missing values within the dataset. Missing data can impact the accuracy of your analysis and conclusions. Depending on the software you are using, you can often generate summary statistics that include counts of missing values per variable, helping you decide how to handle them.
Visualizing Data Distribution
Use histograms, box plots, or scatter plots to visualize the distribution of data across variables. Visualizations provide insights into the data's spread, central tendencies, and potential outliers. Understanding these aspects early on can guide your decisions during the analysis phase.
Preliminary Insights
As you explore the dataset, note any preliminary insights or patterns that catch your attention. These initial observations can shape your hypotheses and guide more focused exploration and analysis later on.
Documentation
Lastly, document your exploration process. Record any data preprocessing steps you take, initial visualizations, and notes on dataset characteristics. Documentation ensures transparency and reproducibility in your analysis, which is crucial for academic assignments and research.
Step 4: Data Preparation
Before diving into calculations, it's crucial to ensure your dataset is clean and ready for analysis. Here’s how you can effectively prepare your data:
- Check for Missing Values: Start by examining your dataset for any missing values. If you find missing data points, decide on the appropriate method to handle them. Common approaches include removing the rows with missing values or imputing them using mean, median, or mode values depending on the nature of your data.
- Verify Data Types: Confirm that each variable is in the correct format for analysis. Numerical variables should be numeric, dates should be in date format, and categorical variables should be appropriately labeled.
- Handle Outliers: Identify and assess any outliers in your dataset. Depending on your analysis goals, outliers may need to be removed or adjusted to minimize their impact on statistical calculations.
- Data Transformation: If necessary, transform your data to meet the assumptions of the statistical tests you plan to perform. Common transformations include logarithmic, square root, or reciprocal transformations to achieve normality or stability of variance.
- Ensure Data Consistency: Check for inconsistencies or errors in data entry. Misentered data can skew results and lead to inaccurate conclusions.
By investing time in thorough data preparation, you lay a solid foundation for accurate and meaningful statistical analysis. This step not only enhances the reliability of your findings but also streamlines the overall assignment process, making it easier to interpret and present your results effectively.
Step 5: Calculate Descriptive Statistics
Descriptive statistics are fundamental in understanding the characteristics of your data. They provide insights into central tendency and variability, essential for any statistical analysis.
- Mean Calculation: The mean, or average, is calculated by summing all values in a dataset and dividing by the number of values. It gives a sense of the central value of the data.
- Standard Deviation: Standard deviation measures the amount of variation or dispersion of a set of values. It indicates how much the data deviates from the mean.
- Interpretation: Understanding these statistics helps in interpreting the data more effectively. A higher standard deviation indicates greater variability among data points, while a lower standard deviation suggests data points are closer to the mean.
By mastering these calculations, you'll be equipped to analyze datasets more comprehensively, making informed decisions based on robust statistical insights.
Step 6: Perform Correlation Analysis
Correlation analysis is a fundamental statistical technique used to measure the strength and direction of the relationship between two or more variables. In the context of your statistics assignment, performing correlation analysis involves calculating correlation coefficients to determine how variables move in relation to each other.
Understanding Correlation Coefficients:
- Pearson Correlation Coefficient: This coefficient measures the linear relationship between two variables. It ranges from -1 (perfect negative correlation) to 1 (perfect positive correlation), with 0 indicating no linear correlation.
Steps to Calculate Correlation Coefficients:
- Identify Variables: Select the pairs of variables you want to analyze for correlation.
- Calculate the Correlation Coefficient: Use statistical software or spreadsheet tools (like Excel) to compute the Pearson correlation coefficient. The formula for Pearson correlation coefficient r between variables X and Y is:
r=∑((X_i−Xˉ)(Y_i−Yˉ))/sqrt[∑(Xi−Xˉ)^2∑(Yi−Yˉ)^2]
Here, X_i and Y_i are individual data points, and Xˉ and Yˉ are the means of variables X and Y, respectively.
- Interpret the Coefficient: Once calculated, interpret the coefficient:
- A coefficient close to 1 or -1 indicates a strong positive or negative correlation, respectively.
- A coefficient close to 0 suggests no significant linear relationship between the variables.
Step 7: Determine Statistical Significance
To determine the statistical significance of correlation coefficients, you need to assess whether the observed relationships between variables are likely to be real or simply due to random chance. This step is crucial in interpreting the strength and direction of these relationships.
Statistical significance is typically assessed using the p-value. The p-value indicates the probability of obtaining a correlation coefficient as extreme as the one observed in your sample data, assuming that there is no true correlation in the population. In most statistical analyses, a p-value of less than 0.05 is considered statistically significant, suggesting that the observed correlation is unlikely to have occurred by chance alone.
In Excel, you can calculate the p-value using the Data Analysis Toolpak. Here’s how you can proceed:
- Open Excel: Ensure the Data Analysis Toolpak is enabled under the Data tab in Excel.
- Data Analysis Toolpak: Go to Data > Data Analysis > Correlation.
- Input Data: Select the ranges of data for the variables you want to analyze.
- Output: The tool will provide you with correlation coefficients and their corresponding p-values.
- Interpretation: Look at the p-values associated with each correlation coefficient. If a p-value is less than 0.05, you can conclude that there is a statistically significant correlation between the variables.
Understanding statistical significance ensures that your analysis is robust and meaningful. It helps you confidently interpret the relationships between variables in your statistics assignment, providing valuable insights into your dataset.
Step 8: Interpret the Results
Once you've calculated the mean, standard deviation, and correlation coefficients for your variables, the next crucial step is to interpret what these results signify. Interpretation involves understanding the implications of your statistical findings and how they relate to the real-world context of your data.
Understanding Mean and Standard Deviation:
- Mean: The average value of a set of numbers. It provides a central tendency measure, indicating the typical value in your dataset for a particular variable.
- Standard Deviation: Measures the dispersion or spread of data points around the mean. A higher standard deviation indicates greater variability in the data.
Interpreting Correlation Coefficients:
- Pearson Correlation Coefficient: Ranges from -1 to 1, where:
- Positive values indicate a positive correlation (both variables increase together).
- Negative values indicate a negative correlation (one variable increases as the other decreases).
- Values closer to 1 or -1 indicate a stronger relationship.
- Values closer to 0 indicate a weaker or no linear relationship.
Significance of Correlation:
- Statistical Significance: Determine the significance of your correlation coefficients using p-values (typically p < 0.05 is considered significant). Significant correlations suggest that the relationship observed in your sample is likely to exist in the population.
Real-World Implications:
- Discuss how your findings can be applied or interpreted in a real-world context. For example, if you find a strong positive correlation between customer satisfaction scores and repeat purchases in a retail dataset, this suggests that higher satisfaction levels may lead to increased customer loyalty.
Visualization (Optional):
- Use graphs or charts to visually represent your findings. Scatter plots are often used to visualize correlations, showing how two variables relate to each other across the dataset.
Conclusion:
- Summarize the key findings and their implications. Explain how your interpretation aligns with the initial objectives of the analysis and what insights it provides into the dataset.
Interpreting the results of your statistical analysis is crucial for deriving meaningful conclusions and actionable insights from your data.
Conclusion
In conclusion, solving your statistics assignment can be a straightforward and rewarding process if you follow a structured approach. By thoroughly understanding the assignment requirements, preparing your tools, and cleaning your data, you set a solid foundation for your analysis. Calculating descriptive statistics and performing correlation analysis are essential steps that provide valuable insights into your dataset. Creating a clear summary table and interpreting your results accurately will help you present your findings effectively. Reviewing and proofreading your work ensures that your analysis is precise and your conclusions are logical. By applying these techniques, you can solve your statistics assignment with confidence and accuracy. Remember, practice makes perfect, and with each assignment, you'll become more proficient in handling statistical tasks.