Nonparametric Methods in Data Analysis: Robust and Flexible Statistical Insights
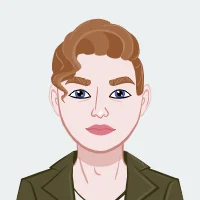
Nonparametric methods hold a vital place in data analysis due to their flexibility and robustness. Unlike parametric methods, which rely on specific assumptions about the population parameters and data distribution, nonparametric methods make fewer assumptions, allowing for broader application. This makes them especially useful when data doesn't meet the usual assumptions of normality, or when dealing with ordinal or nominal data. If you're looking to solve your statistics assignment effectively, understanding nonparametric methods is crucial. These methods provide reliable alternatives to traditional parametric techniques, making them indispensable tools for analyzing real-world data. This blog will guide you through the essentials of nonparametric methods, offering practical tips, detailed explanations, and examples. By mastering these techniques, you can confidently complete your data analysis assignment, ensuring accurate and meaningful insights from your data analysis.
Introduction to Nonparametric Methods
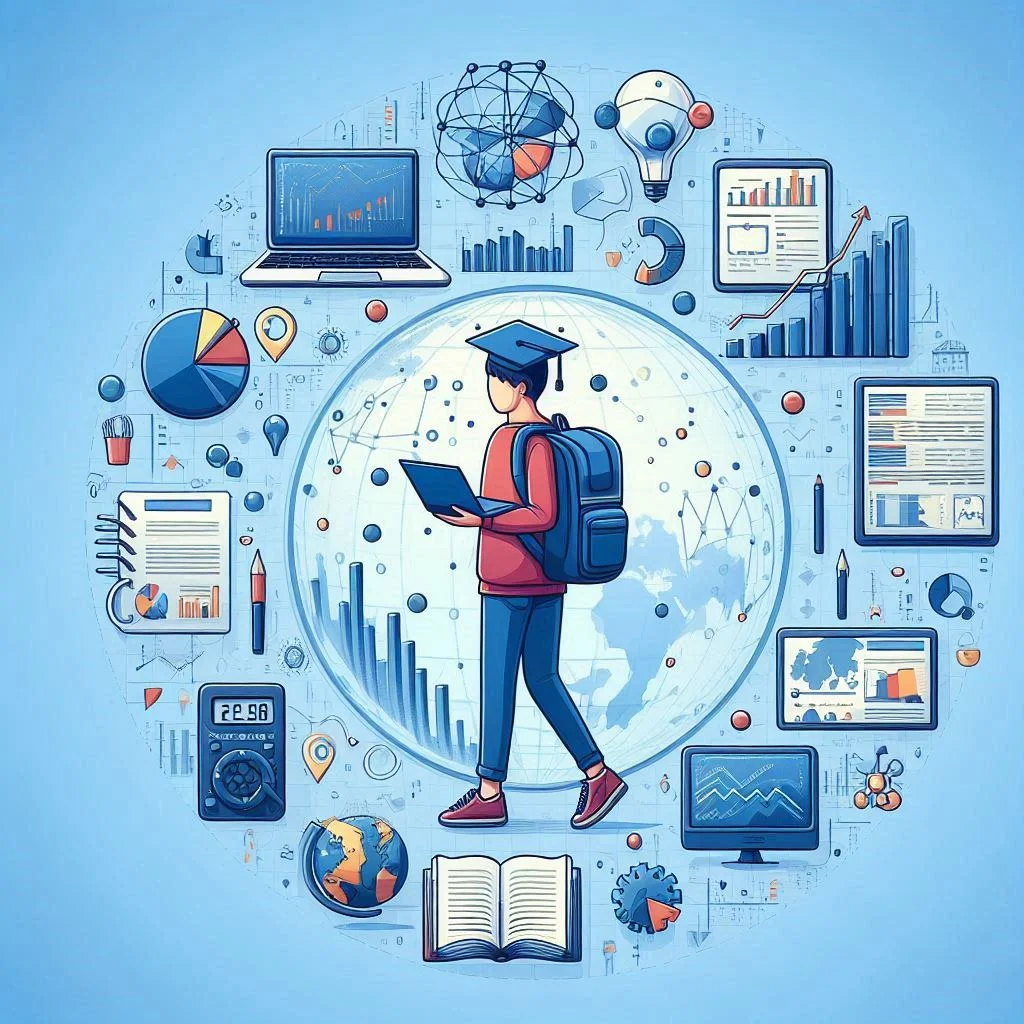
Nonparametric methods are designed to be used when the data do not meet the assumptions required for parametric tests, such as normal distribution, homoscedasticity, or linearity. These methods are particularly useful for analyzing ordinal data, ranks, or data with significant outliers.
Nonparametric methods are essential tools in the statistician’s arsenal because of their flexibility and applicability to various data types. They are less sensitive to outliers and can be used with small sample sizes, making them versatile and robust. Whether you are dealing with skewed data, ordinal data, or non-normally distributed data, nonparametric methods provide reliable alternatives to traditional parametric techniques.
Why Use Nonparametric Methods?
There are several reasons why nonparametric methods are preferred in certain situations:
- Flexibility: Nonparametric methods can be applied to a wide range of data types, including ordinal, nominal, and interval data. They do not require the data to conform to specific distributions, making them versatile tools in data analysis.
- Robustness: Nonparametric methods are less affected by outliers and skewed distributions. This robustness makes them suitable for real-world data, which often do not meet the ideal assumptions required for parametric tests.
- Simplicity: Many nonparametric methods are easier to understand and interpret compared to their parametric counterparts. They often involve simple rank-based calculations, making them accessible to a broader audience.
- Versatility: Nonparametric methods can be used when the sample size is small, or when the data does not meet the assumptions required for parametric tests. This makes them valuable tools for researchers dealing with limited or non-ideal datasets.
Common Nonparametric Methods
There are several commonly used nonparametric methods in data analysis. Understanding these methods and knowing when to apply them is crucial for tackling statistics assignments effectively. Here are a few key nonparametric methods:
- Mann-Whitney U Test: The Mann-Whitney U Test is a powerful nonparametric method used to compare differences between two independent groups. This test is particularly useful when the dependent variable is ordinal or continuous but not normally distributed. It assesses whether there is a significant difference in the distributions of the two groups, without assuming a normal distribution. To apply the Mann-Whitney U Test, rank all the observations from both groups together, then calculate the U statistic based on the sum of the ranks for each group. This test provides a robust alternative to the independent t-test, making it ideal for real-world data that often deviates from parametric assumptions. Using this test can help solve your statistics assignment with confidence.
- Wilcoxon Signed-Rank Test: The Wilcoxon Signed-Rank Test is a powerful nonparametric test used for comparing two related samples to assess whether their population mean ranks differ. It is the nonparametric alternative to the paired t-test and is particularly useful when the data do not meet the assumptions of normality. This test ranks the differences between paired observations, taking into account the direction of the differences. It then evaluates whether the ranks are symmetrically distributed around zero. By using the Wilcoxon Signed-Rank Test, you can solve your statistics assignment effectively, especially when dealing with small sample sizes or data with outliers. This method ensures robust and reliable results, providing deeper insights into the relationships between paired samples.
- Kruskal-Wallis H Test: The Kruskal-Wallis H Test is a powerful nonparametric method used to compare differences between more than two groups when the dependent variable is ordinal or continuous but not normally distributed. It serves as the nonparametric alternative to ANOVA (Analysis of Variance). By ranking the data and comparing the sum of ranks between groups, the Kruskal-Wallis H Test determines whether there are statistically significant differences among the groups. This test is particularly useful in scenarios where the assumptions of ANOVA are violated, such as unequal variances or non-normal distributions. Applying the Kruskal-Wallis H Test can help you solve your statistics assignment by providing robust and reliable results across multiple group comparisons.
- Friedman Test: The Friedman Test is a nonparametric statistical test used to compare more than two related groups or treatments. It is particularly useful when dealing with repeated measures or matched subjects, where the same individuals are measured under different conditions. Unlike parametric tests such as repeated measures ANOVA, the Friedman Test does not assume normal distribution and is ideal for ordinal data or data that do not meet parametric assumptions. The test ranks the data within each block and then assesses whether the ranks differ significantly across treatments. This approach provides a robust method for analyzing differences in treatments or conditions when the assumptions for parametric tests are not met, making it a valuable tool in data analysis.
- Spearman’s Rank Correlation: Spearman’s Rank Correlation is a nonparametric test used to measure the strength and direction of the association between two ranked variables. Unlike Pearson’s correlation, which requires interval data and assumes a linear relationship, Spearman’s Rank Correlation evaluates relationships based on the rank orders of the data rather than their raw values. This makes it particularly useful for ordinal data or when the data do not meet the assumptions of normality. The Spearman’s rank coefficient, denoted as ρ\rhoρ, ranges from -1 to 1, where -1 indicates a perfect negative correlation, 1 indicates a perfect positive correlation, and 0 indicates no correlation. By using Spearman’s Rank Correlation, you can assess how well the ranks of one variable predict the ranks of another, providing valuable insights into their relationship.
- Chi-Square Test: The Chi-Square Test is a fundamental nonparametric method used to examine the relationship between categorical variables. It assesses whether the observed frequencies in each category differ significantly from the frequencies expected under the null hypothesis of no association. This test is particularly useful in analyzing survey data, contingency tables, and categorical outcomes. For example, you might use a Chi-Square Test to determine if there’s a significant relationship between gender and preference for a product. To perform the test, you calculate the Chi-Square statistic, which measures the discrepancy between observed and expected frequencies. A high Chi-Square value indicates a significant difference, suggesting a relationship between the variables. By understanding and applying this test, you can solve your statistics assignment with precision and rigor.
Steps to Solve Assignments Using Nonparametric Methods
To complete your assignments on nonparametric methods, follow these steps: first, identify your research question and hypotheses. Next, select the appropriate nonparametric test based on your data type and research goals. Conduct the test carefully, check assumptions, and interpret results in context. Finally, report your findings clearly and accurately to ensure effective analysis.
- Identify the Research Question and Hypotheses: Clearly define the research question and formulate the null and alternative hypotheses. This step is crucial as it guides the selection of the appropriate nonparametric method.
- Choose the Appropriate Nonparametric Method: Based on the type of data and the research question, select the most suitable nonparametric test. Consider factors such as the number of groups, the relationship between the groups (independent or related), and the level of measurement of the data (ordinal, nominal, interval).
- Check Assumptions: Although nonparametric methods make fewer assumptions, it is still important to ensure that the data meet the assumptions required for the chosen test. For example, the Mann-Whitney U test assumes that the two samples are independent, and the observations are ordinal or continuous.
- Conduct the Test: Perform the chosen nonparametric test using statistical software or manual calculations. Ensure that the test is conducted correctly, following the steps required for the specific method.
- Interpret the Results: Interpret the results in the context of the research question and hypotheses. Nonparametric tests often provide p-values and test statistics, which help determine whether to reject the null hypothesis.
- Report the Findings: Clearly report the findings, including the test used, the test statistics, p-values, and the conclusion. Ensure that the report is comprehensive and provides enough detail for the reader to understand the analysis and results.
Practical Tips for Solving Nonparametric Testing Assignments
When tackling nonparametric testing assignments, start by thoroughly understanding your data and the research question. Choose the right test based on the data type and hypothesis. Use statistical software for accuracy and efficiency. Practice regularly to build confidence, and seek guidance if needed. These steps will help you solve your statistics assignment effectively.
- Understand the Data: Before applying any statistical test, it is essential to understand the nature of the data. This includes knowing the level of measurement, the distribution, and any potential outliers.
- Use Statistical Software: While it is important to understand the underlying calculations, using statistical software can save time and reduce the risk of errors. Popular software such as R, SPSS, and Python can perform nonparametric tests efficiently.
- Practice: The best way to master nonparametric methods is through practice. Work on multiple assignments and datasets to gain confidence and proficiency in applying these methods.
- Seek Help When Needed: If you encounter difficulties, do not hesitate to seek help from your instructors, peers, or online resources. You can also get additional assistance from non-parametric testing assignment help services. There are numerous services and forums that can provide guidance and support.
- Stay Updated: Nonparametric methods, like other statistical techniques, continue to evolve. Stay updated with the latest developments and advancements in the field to enhance your knowledge and skills.
Examples of Nonparametric Methods in Action
To illustrate the application of nonparametric methods, let’s consider a few examples:
- Mann-Whitney U Test: Suppose you are comparing the test scores of two independent groups of students, where the scores are not normally distributed. The Mann-Whitney U test can be used to determine whether there is a significant difference between the two groups.
- Wilcoxon Signed-Rank Test: Imagine you have pre-test and post-test scores for a group of students who participated in a training program. The Wilcoxon Signed-Rank test can be used to assess whether the training program had a significant effect on their scores.
- Kruskal-Wallis H Test: If you are comparing the satisfaction levels of customers across three different stores, and the satisfaction scores are ordinal, the Kruskal-Wallis H test can be used to determine whether there are significant differences between the stores.
- Friedman Test: Suppose you have three different teaching methods and you want to compare their effectiveness based on students’ test scores. The Friedman test can be used to analyze the differences between the teaching methods when the data is related (e.g., the same students are tested under each method).
- Spearman’s Rank Correlation: If you are studying the relationship between the ranks of students in two different subjects, Spearman’s Rank Correlation can be used to measure the strength and direction of the association between the ranks.
- Chi-Square Test: Imagine you are testing whether there is an association between gender and preference for a new product. The Chi-Square test can be used to analyze the relationship between these categorical variables.
Conclusion
Nonparametric methods are indispensable for data analysis, offering flexibility and robustness when parametric assumptions are not met. These methods are less sensitive to outliers, applicable to a variety of data types, and suitable for small sample sizes, making them ideal for real-world data analysis. By understanding and applying nonparametric methods, you can solve your statistics assignment with greater confidence and accuracy. Selecting the appropriate nonparametric test, conducting it correctly, and interpreting the results effectively are crucial steps in the process. Remember to stay updated with the latest advancements in nonparametric methods to enhance your skills. With practice and the right approach, you can leverage nonparametric methods to derive meaningful insights from your data, ensuring your statistics assignments are handled proficiently and successfully.