Repeated Measures ANOVA: Degrees of Freedom, Sphericity, and Correction Factors
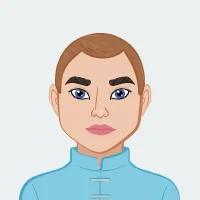
Solving your statistics assignment can be challenging, especially when dealing with complex analyses like repeated measures ANOVA. This statistical method is invaluable for analyzing data collected from the same subjects over multiple time points or under different conditions. By understanding and applying repeated measures ANOVA, you can draw meaningful conclusions from your data while accounting for variability between subjects. This blog will explore the essential components of repeated measure ANOVA, including degrees of freedom, the assumption of sphericity, Mauchly’s Test, and correction factors. Whether you are new to this statistical technique or looking to refine your skills, this comprehensive guide will provide the insights you need to effectively complete your statistics assignment and achieve accurate, reliable results.
1. Understanding Repeated Measures ANOVA
Repeated measures ANOVA is a statistical technique used to test for differences in the means of several related groups. These groups can be different measurements taken from the same subjects under different conditions or at different time points. This method is particularly useful because it accounts for the variability between subjects, leading to more powerful statistical tests.
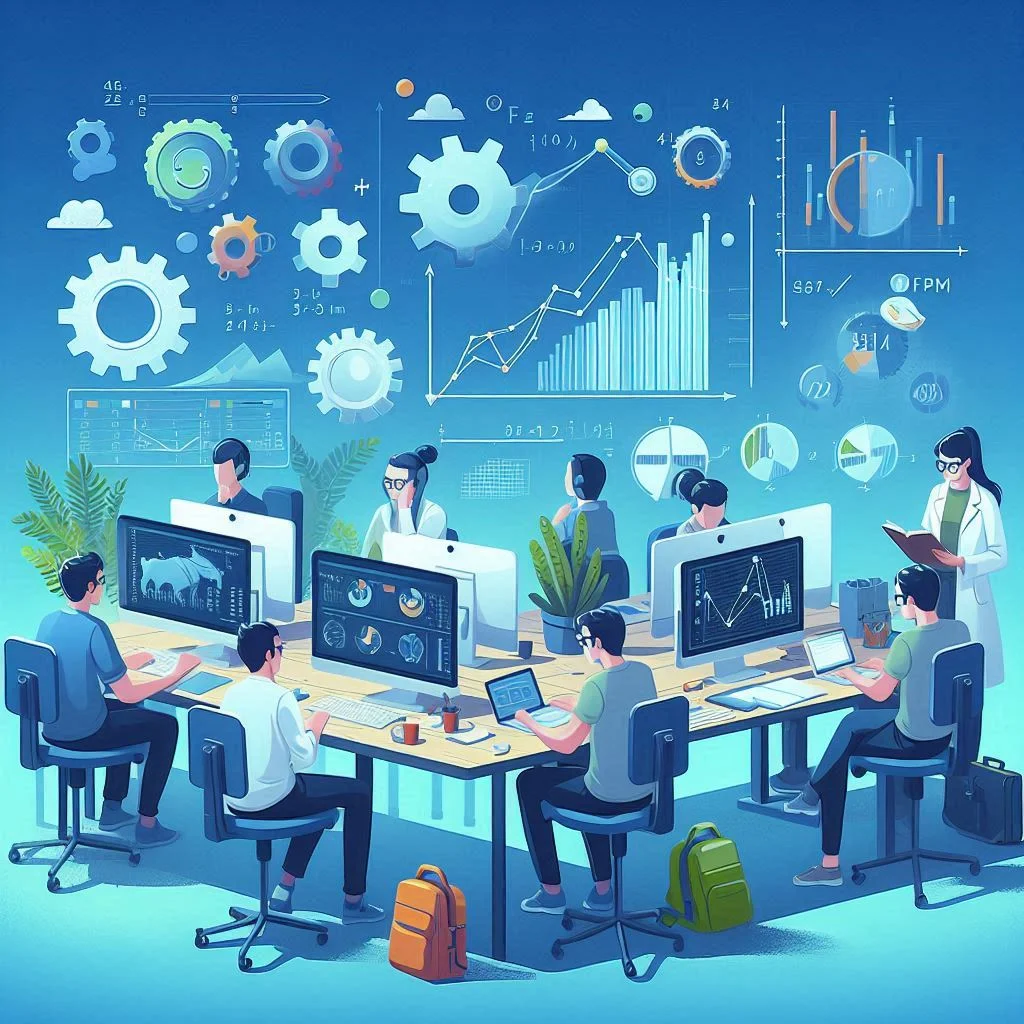
Key Steps in Conducting Repeated Measures ANOVA:
Step 1: Formulate Hypotheses
- Null Hypothesis (H0H_0H0): There are no differences in the means across the different conditions or time points.
- Alternative Hypothesis (H1H_1H1): There are significant differences in the means across the different conditions or time points.
Step 2: Collect and Organize Data
- Ensure that your data is collected consistently across all conditions or time points. Organize the data in a format suitable for analysis, typically with rows representing subjects and columns representing the different conditions or time points.
Step 3: Check Assumptions
- Normality: The differences between the paired observations should be approximately normally distributed.
- Sphericity: The variances of the differences between all combinations of related groups should be equal.
Step 4: Conduct the ANOVA
- Interpret the output to determine if there are significant differences between the conditions or time points.
2. Degrees of Freedom in Repeated Measures ANOVA
Degrees of Freedom(df) are a fundamental aspect of statistical analyses, including repeated measures ANOVA. They play a crucial role in determining the shape of the F-distribution used in hypothesis testing, which in turn affects the significance of the results. Understanding how to calculate and interpret degrees of freedom is essential for accurately solving your statistics assignment.
Types of Degrees of Freedom:
In repeated measures ANOVA, there are several types of degrees of freedom to consider:
- Between-Subjects Degrees of Freedom (df_{B})
- Within-Subjects Degrees of Freedom (df_{W})
- Error Degrees of Freedom (df_{E})
Between-Subjects Degrees of Freedom (df_{B}):
Between-subjects degrees of freedom relate to the number of subjects in the study. This type of degree of freedom accounts for the variability between different subjects.
- Formula: df_{B} = N - 1
- Example Calculation: If there are 10 subjects in the study, df_{B} = 10 - 1 = 9.
This calculation reflects the total number of subjects minus one. It essentially measures the number of independent pieces of information available to estimate the between-subjects variability.
Within-Subjects Degrees of Freedom (df_{W}):
Within-subjects degrees of freedom refer to the number of measurements or conditions applied to each subject. This degree of freedom captures the variability within the same subject across different conditions or time points.
- Formula: df_{W} = k - 1
- Example Calculation: If there are four time points (Baseline, 2 months, 4 months, and 6 months), df_{W} = 4 - 1 = 3.
This calculation indicates the number of conditions or time points minus one, representing the number of comparisons that can be made within subjects.
Error Degrees of Freedom (df_{E}):
Error degrees of freedom account for the residual variability after considering the within-subjects and between-subjects effects. This is also known as the residual degrees of freedom.
- Formula: df_{E} = (k−1)×(N−1)
- Example Calculation: With 10 subjects and four time points, df_{E} = (4−1)×(10−1)=3×9=27.
This calculation reflects the product of the within-subjects degrees of freedom and the between-subjects degrees of freedom. It measures the leftover variability that cannot be attributed to either the subjects or the conditions.
3. The Assumption of Sphericity
The assumption of sphericity is a crucial concept in repeated measures ANOVA, often challenging for students to grasp and apply. When solving your statistics assignment, understanding this assumption and its implications can significantly impact the accuracy of your analysis and the validity of your conclusions.
Definition of Sphericity:
Sphericity refers to the condition where the variances of the differences between all possible pairs of within-subject conditions (or time points) are equal. This assumption is essential because it ensures the validity of the F-ratios computed in repeated measures ANOVA. If sphericity is violated, the test statistics may be biased, leading to incorrect conclusions about the effects being studied.
Why Sphericity Matters:
In repeated measures ANOVA, the assumption of sphericity is similar to the assumption of homogeneity of variances in between-subjects ANOVA. It ensures that the covariance matrix of the repeated measures is proportional to an identity matrix, which allows for valid hypothesis testing. Violations of sphericity can lead to:
- Inflated Type I Error Rates: The likelihood of incorrectly rejecting a true null hypothesis increases, meaning you might conclude that there is a significant effect when there isn't one.
- Reduced Statistical Power: The ability to detect a true effect is diminished, potentially leading to a failure to identify significant differences when they exist.
Testing for Sphericity:
One of the common methods to test for sphericity is Mauchly’s Test of Sphericity. This test evaluates the null hypothesis that the variances of the differences are equal.
- Mauchly’s Test: If the test is significant (p-value < 0.05), it indicates a violation of the sphericity assumption, meaning the variances of the differences are not equal.
However, Mauchly’s Test is sensitive to sample size, and with small sample sizes, it may not detect violations of sphericity accurately. Conversely, with large sample sizes, even minor deviations from sphericity can lead to significant test results.
Dealing with Violations of Sphericity:
When Mauchly’s Test indicates a violation of sphericity, several corrective measures can be applied to adjust the degrees of freedom of the F-ratio, thereby reducing the likelihood of Type I errors. The most common correction methods include:
- Greenhouse-Geisser Correction: This method adjusts the degrees of freedom based on an estimated epsilon (ε), which ranges between 1/(k-1) and 1, where k is the number of measurements. The closer ε is to 1, the less severe the violation of sphericity.
- Huynh-Feldt Correction: This correction is generally less conservative than Greenhouse-Geisser and is used when the Greenhouse-Geisser epsilon estimate is too conservative (i.e., much less than 1).
Interpreting Corrected Results:
After applying a correction for the violation of sphericity, you should interpret the adjusted degrees of freedom and the corresponding F-ratios. The corrected F-ratios help mitigate the impact of the violation and provide a more accurate assessment of the within-subject effects.
4. Mauchly’s Test of Sphericity and Correction Factors
When conducting repeated measures ANOVA, one of the critical assumptions to test is sphericity, which ensures the equality of variances of the differences between all combinations of related groups. Mauchly’s Test of Sphericity is a standard procedure to assess this assumption. Understanding how to perform and interpret this test, along with applying necessary correction factors, is essential for accurately solving your statistics assignment.
Mauchly’s Test of Sphericity:
Mauchly’s Test evaluates the null hypothesis that the covariance matrix of the repeated measures is proportional to an identity matrix, implying that the variances of the differences are equal. Here's how to conduct and interpret the test:
- Null Hypothesis (H0): The variances of the differences between conditions are equal (sphericity is met).
- Alternative Hypothesis (H1): The variances of the differences between conditions are not equal (sphericity is violated).
Procedure:
- Calculate the Test Statistic: Mauchly's W statistic is computed, which follows a chi-square distribution under the null hypothesis.
- Compare to Critical Value: The W statistic is compared to a chi-square distribution with degrees of freedom equal to (k - 1)(k - 2)/2, where k is the number of levels or conditions.
- Determine Significance: If the p-value associated with the W statistic is less than the chosen significance level (typically 0.05), sphericity is violated.
Interpreting Mauchly’s Test Results:
- Non-Significant Result (p > 0.05): Sphericity is assumed, and no correction is needed. You can proceed with the standard repeated measures ANOVA.
- Significant Result (p < 0.05): Sphericity is violated, indicating that the variances of the differences are not equal. In this case, it is necessary to apply correction factors to adjust the degrees of freedom of the F-tests to avoid inflated Type I error rates.
Mauchly’s Test of Sphericity and the appropriate correction factors are vital components in repeated measures ANOVA. Understanding when and how to apply these corrections ensures the validity and reliability of your statistical analysis. Accurately solving your statistics assignment requires not only conducting these tests but also correctly interpreting their results and applying the necessary adjustments to avoid incorrect conclusions and inflated error rates. This approach ultimately leads to more robust and credible findings in your research.
5. Interpreting Corrected Results
When sphericity is violated, it is prudent to interpret the results using the corrected degrees of freedom rather than the sphericity-assumed results. The corrected results provide a more accurate reflection of the true statistical significance.
Choosing the Appropriate Correction:
- If the Greenhouse-Geisser ε is very small (close to 0), it indicates severe sphericity violation, and the Greenhouse-Geisser correction should be used.
- If the Greenhouse-Geisser ε is relatively large (close to 1), the Huynh-Feldt correction can be considered.
6. Practical Application: Conducting a Repeated Measures ANOVA
Let's walk through a practical example of conducting a repeated measures ANOVA. Assume you have data from a study measuring the working memory performance of older adults at four different time points.
Step-by-Step Guide:
Step 1: Data Preparation
- Organize your data in a spreadsheet or statistical software with rows representing subjects and columns representing the time points (e.g., Baseline, 2 months, 4 months, 6 months).
Step 2: Descriptive Statistics
- Calculate the mean and standard deviation for each time point to get an overview of the data.
Step 3: Test Assumptions
- Normality: Use graphical methods (e.g., Q-Q plots) and statistical tests (e.g., Shapiro-Wilk test) to check for normality of the differences.
- Sphericity: Conduct Mauchly’s Test of Sphericity to assess the assumption.
Step 4: Perform the ANOVA
- Use statistical software to conduct the repeated measures ANOVA. Input the data and specify the within-subjects factor (time points).
Step 5: Interpret the Output
- Examine the F-statistics and p-values for the within-subjects effects. If Mauchly’s Test indicates a violation of sphericity, use the Greenhouse-Geisser or Huynh-Feldt corrected results.
7. Reporting Results
When reporting the results of a repeated measures ANOVA, it is essential to include the following components:
- Descriptive Statistics: Provide the means and standard deviations for each time point or condition.
- ANOVA Results: Report the F-statistics, degrees of freedom, and p-values for the within-subjects effects. If corrections were applied, report the corrected results.
- Sphericity Assumption: Mention whether the assumption of sphericity was met and the results of Mauchly’s Test.
- Interpretation: Discuss the practical implications of the findings. If significant differences were found, describe the nature of these differences (e.g., which time points or conditions showed improvement).
Tips for Solving Similar Assignments
To successfully tackle assignments involving repeated measures ANOVA, consider the following tips:
- Understand the Research Context: Clearly understand the research question and the experimental design. Knowing the context helps in formulating appropriate hypotheses and interpreting the results.
- Check Assumptions Early: Assess the assumptions of normality and sphericity before performing the ANOVA. This proactive step can save time and ensure the validity of your results.
- Use Software Effectively: Familiarize yourself with statistical software (e.g., SPSS, R, SAS) to efficiently conduct the analysis and interpret the output. Many software packages provide detailed documentation and tutorials.
- Seek Help When Needed: Understanding complex statistical concepts like repeated measures ANOVA can be challenging. Don’t hesitate to seek help from instructors, peers, or online ANOVA assignment help services. These resources can provide valuable insights, clarify doubts, and guide you through intricate analyses, ensuring you accurately solve your statistics assignments and achieve better results.
- Practice Regularly: Like any skill, proficiency in statistical analysis comes with practice. Work on different datasets and practice conducting repeated measures ANOVAs to build confidence.
Conclusion
Successfully solving your statistics assignment involving repeated measures ANOVA requires a thorough understanding of the method’s key components. By mastering the calculation of degrees of freedom, testing the assumption of sphericity, interpreting Mauchly’s Test results, and applying appropriate correction factors, you can ensure the accuracy and validity of your analysis. This comprehensive guide has equipped you with the knowledge to confidently approach repeated measures ANOVA, helping you to solve your statistics assignment with precision. Regular practice and seeking assistance when needed will further enhance your proficiency in this essential statistical technique, enabling you to draw meaningful and reliable conclusions from your data.