Demystifying Structural Equation Modeling (SEM) with AMOS: Solving Statistics Assignments
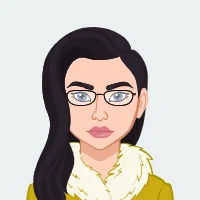
Structural Equation Modeling (SEM) is valuable in fields like social sciences, psychology, and business, where understanding these intricate connections is crucial. For students tasked with SEM assignments, mastering this method can be challenging but immensely rewarding. AMOS (Analysis of Moment Structures) is a powerful software that simplifies SEM, making it accessible for those who might not have extensive statistical backgrounds. This blog aims to guide you through the essential steps and best practices to help you solve your statistics assignment effectively. By understanding the intricacies of SEM and leveraging AMOS, you can gain deeper insights into your data and produce robust, reliable analyses. Whether you're new to SEM or looking to refine your skills, this guide will provide the foundational knowledge and practical tips you need to succeed.
Understanding Structural Equation Modeling (SEM)
Structural Equation Modeling (SEM) is used to analyze complex relationships among observed and latent variables. It combines elements of factor analysis and multiple regression, allowing for the simultaneous examination of multiple equations. This makes SEM particularly useful for testing theoretical models that involve multiple interrelated variables. By mastering SEM, students can conduct comprehensive analyses that go beyond traditional regression techniques, allowing for a deeper understanding of the underlying structures in their data. This foundation is essential for solving your AMOS-SEM assignments effectively, enabling you to apply these principles and methods to a wide range of research questions and datasets.
-with-AMOS.webp)
Getting Started with AMOS
AMOS (Analysis of Moment Structures) is a user-friendly software that facilitates SEM. Here’s how you can start using AMOS for your SEM projects:
Step 1: Model Specification:
Model specification is the foundational step in Structural Equation Modeling (SEM), where you define the relationships between variables based on theoretical frameworks or prior research. This process involves identifying the dependent and independent variables and establishing the expected connections among them.
- Defining Variables: Start by clearly defining the latent and observed variables in your study. Latent variables are unobserved constructs like intelligence or customer satisfaction, while observed variables are directly measured indicators like test scores or survey responses.
- Developing the Hypothesized Model: Formulate a hypothesized model that outlines how you believe the variables are related. This includes specifying which variables are dependent (outcome variables) and which are independent (predictor variables). For instance, in a study on customer satisfaction, you might hypothesize that trust in the company and loyalty are predictors of overall satisfaction.
- Creating a Path Diagram: Visualize your hypothesized model using a path diagram. Path diagrams are graphical representations that show the relationships between variables with arrows indicating the direction of influence. Direct paths represent hypothesized direct effects, while indirect paths may indicate mediated relationships.
- Establishing Relationships: Specify the nature of the relationships between variables. Determine which variables have direct effects on the dependent variable and identify any mediating or moderating variables that might influence these relationships. For example, you might posit that trust in the company directly affects customer satisfaction, while loyalty mediates the relationship between trust and satisfaction.
- Setting Parameters: Define the parameters to be estimated in your model. These parameters include the path coefficients (regression weights), variances, and covariances. Each parameter represents a hypothesized effect or relationship that you want to test.
- Assumptions and Constraints: Consider any assumptions or constraints in your model. SEM relies on several assumptions, including multivariate normality, linearity, and independence of observations. Ensure that your model meets these assumptions or adjust it accordingly. Constraints might involve fixing certain parameters to specific values based on theoretical justifications.
- Documenting the Model: Thoroughly document your model specification, including the rationale for choosing specific variables and relationships. This documentation is crucial for transparency and reproducibility, allowing others to understand and replicate your analysis.
Model specification is a critical step that sets the stage for the entire SEM analysis. A well-specified model, grounded in theory and clearly defined relationships, ensures that your subsequent analysis in AMOS will be robust and meaningful, helping you effectively solve your AMOS-SEM assignment.
Step 2: Data Preparation:
Data preparation is a crucial step in Structural Equation Modeling (SEM) as it ensures that your data is clean, well-organized, and ready for analysis in AMOS. Proper data preparation helps avoid errors during the analysis and ensures that the results are accurate and reliable.
First, gather all the necessary data relevant to your model. This data should include both observed and latent variables that you plan to analyze. Make sure the data is complete, as missing values can significantly affect the results of your SEM analysis.
Next, examine the data for any inconsistencies or outliers. Inconsistent data, such as incorrect entries or impossible values, need to be corrected or removed. Outliers should be carefully considered; while they can provide valuable insights, they might also skew your results if they are due to errors or anomalies.
Ensure that your data is in the correct format for AMOS. Typically, this involves using a statistical software package like SPSS to organize and format your dataset. AMOS requires data files to be in SPSS format, so save your cleaned data accordingly.
It's also important to check for multicollinearity among your independent variables. High multicollinearity can make it difficult to determine the individual effect of each variable on the dependent variable. Use variance inflation factor (VIF) or correlation matrices to identify and address multicollinearity issues.
Additionally, consider the distribution of your data. SEM assumes that the data is normally distributed. If your data deviates significantly from a normal distribution, you might need to transform the variables or use robust estimation techniques provided by AMOS.
Lastly, define and code your variables appropriately. Each variable should have a clear and consistent coding scheme to ensure accurate analysis. For example, categorical variables should be coded numerically (e.g., 0 and 1 for binary variables), and continuous variables should be scaled correctly.
Step 3: Running the Analysis:
Running the analysis in AMOS involves several key steps that allow you to estimate the parameters of your structural equation model and evaluate its fit to the data. Here’s how to proceed:
- Loading Your Data: Start by loading your prepared dataset into AMOS. Ensure that your data is in the correct format (usually SPSS) and that all necessary variables are included.
- Drawing the Model: Using the graphical interface in AMOS, draw your SEM model. This involves placing your variables (both observed and latent) onto the canvas and connecting them with arrows to represent hypothesized relationships. Ensure that you accurately depict the paths, distinguishing between direct effects (single-headed arrows) and correlations (double-headed arrows).
- Specifying the Model: After drawing the model, specify the relationships between variables. Define which variables are dependent and independent, and indicate any covariances or correlations among them. This step ensures that AMOS understands the structure of your model and the nature of the relationships you are analyzing.
- Setting Parameters: Specify initial parameter values if necessary, although AMOS typically assigns starting values automatically. You may also need to set constraints on certain parameters based on theoretical considerations or previous research.
- Running the Estimation: Execute the model by clicking the "Calculate Estimates" button. AMOS will then perform the necessary calculations to estimate the parameters of your model. This process involves iterative algorithms that seek to minimize the discrepancy between your hypothesized model and the observed data.
- Model Diagnostics: Examine diagnostic indices and modification indices provided by AMOS. These indices can help identify potential areas for model improvement, such as adding or removing paths to enhance model fit.
- Refining the Model: Based on the output, you may need to refine your model. This could involve modifying paths, adding new variables, or re-specifying relationships to improve the overall fit and validity of the model.
By carefully running and interpreting the analysis in AMOS, you can effectively solve your AMOS-SEM assignment, gaining valuable insights into the relationships among your variables and ensuring that your model provides a robust and accurate representation of the data.
Step 4: Interpreting AMOS Output:
Interpreting the output from AMOS is a critical step in the structural equation modeling process, as it allows you to understand the relationships between variables and assess the overall fit of your model. Here's how to make sense of the various elements in the AMOS output:
- Chi-Square Value and Degrees of Freedom: These are key indicators of model fit. A non-significant Chi-Square value suggests a good fit between your model and the data.
- Goodness-of-Fit Indices: Look at other indices like CFI (Comparative Fit Index), TLI (Tucker-Lewis Index), and RMSEA (Root Mean Square Error of Approximation) to assess model fit.
- Path Coefficients: Interpret the estimated path coefficients to understand the strength and direction of relationships between variables.
- Standardized Estimates: Standardized estimates provide a way to compare the strength of relationships on a common scale, usually ranging from -1 to 1. These can be particularly useful when dealing with variables measured on different scales.
- Residuals: Examining the residuals (the differences between observed and predicted values) helps identify areas where the model may not fit well. Large residuals may indicate model misspecification or the need for additional variables.
- Modification Indices: AMOS provides modification indices that suggest changes to improve model fit, such as adding or removing paths. While these suggestions can be helpful, they should be considered in the context of theoretical justification rather than purely statistical criteria.
By carefully interpreting these outputs, you can assess the adequacy of your SEM model, understand the relationships between variables, and make informed decisions about model modifications. This comprehensive interpretation is essential for solving your AMOS-SEM assignment and ensuring that your analysis is both robust and meaningful.
Step 5: Model Refinement:
Model refinement is a crucial step in Structural Equation Modeling (SEM) to ensure that the model accurately represents the data and fits well statistically. After the initial model estimation, it’s often necessary to make adjustments based on the output and diagnostics provided by AMOS. Here’s how to approach this process:
- Assessing Model Fit: Start by examining the fit indices from the AMOS output. Key indicators such as the Chi-Square value, Comparative Fit Index (CFI), Tucker-Lewis Index (TLI), and Root Mean Square Error of Approximation (RMSEA) provide insight into how well your model fits the data. A good model fit typically has a non-significant Chi-Square, CFI and TLI values close to 1, and an RMSEA value below 0.06.
- Interpreting Modification Indices: AMOS provides modification indices, which suggest potential changes to improve model fit. These indices indicate where additional paths might be added or constraints might be relaxed. However, it’s essential to make theoretical sense of these suggestions before implementing them. Adding paths purely to improve fit without theoretical justification can lead to overfitting and reduce the model’s generalizability.
- Re-estimating the Model: After making adjustments, re-estimate the model to see if the refinements have improved the fit. Compare the new fit indices with the previous ones to ensure that the changes have led to a better model. It may be necessary to go through several iterations of refinement and re-estimation to achieve an optimal model.
- Checking for Overfitting: While it’s important to improve model fit, be cautious of overfitting, where the model becomes too tailored to the sample data and loses its applicability to other datasets. To avoid this, ensure that the refinements are theoretically justified and not just data-driven adjustments.
In summary, refining your SEM model involves a combination of examining fit indices, interpreting modification suggestions, making theoretically justified adjustments, and re-estimating the model. This process ensures that your SEM analysis is both statistically sound and theoretically robust, helping you solve your AMOS-SEM assignment with confidence.
Step 6: Reporting Results:
Reporting the results of your Structural Equation Modeling (SEM) analysis is a critical step that communicates the findings of your research. Here’s how to present your results:
- Interpret Path Coefficients: When reporting SEM results, start by interpreting the path coefficients. These coefficients indicate the strength and direction of relationships between variables. For example, a path coefficient of 0.60 suggests a moderate positive relationship between variables.
- Discuss Model Fit Indices: Include a discussion of the model fit indices, such as the Chi-Square test, Comparative Fit Index (CFI), Tucker-Lewis Index (TLI), and Root Mean Square Error of Approximation (RMSEA). Explain what each index measures and how it reflects the goodness of fit of your model to the data.
- Evaluate Significance Levels: Highlight the significance levels of path coefficients and other relevant statistical tests. Significance indicates whether the observed relationships in your model are likely to be real and not due to random chance.
- Provide Contextual Interpretation: Interpret the results in the context of your research question or hypothesis. Discuss the implications of the findings and how they contribute to existing literature or theory in your field.
- Visualize Results: Use visual aids such as path diagrams, charts, or tables to present complex relationships and make the results more accessible to your audience.
- Discuss Limitations: Acknowledge any limitations or assumptions of your SEM analysis. This might include issues with model specification, data quality, or the generalizability of findings.
Effective reporting of SEM results not only demonstrates your understanding of statistical analysis but also contributes to the broader academic or professional discourse in your field. By following these steps, you can ensure that your findings are clear, meaningful, and appropriately contextualized within the scope of your research.
Conclusion
Mastering Structural Equation Modeling (SEM) using AMOS is an invaluable skill for any student engaged in complex statistical analyses. This guide has outlined the key steps and considerations to help you solve your AMOS-SEM assignment with confidence and precision. From specifying your model and preparing your data to interpreting AMOS output and refining your analysis, each step is crucial in producing accurate and meaningful results. While challenges such as convergence issues and model identification can arise, understanding these concepts and applying best practices will enhance your proficiency in SEM. Remember, solving your AMOS-SEM assignment is not just about completing a task; it's about developing a deeper understanding of statistical relationships and improving your analytical capabilities. With practice and dedication, you can master AMOS and SEM, paving the way for academic and professional success in fields that rely heavily on robust statistical analysis.