Variance Fundamentals: t-Distribution and Normal Distribution Explained
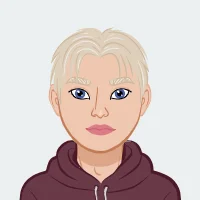
Variance is a key concept in statistics, representing how much the values of a random variable deviate from the mean. This blog explores the behavior of variance in two fundamental distributions: the t-distribution and the normal distribution. By understanding how variance operates in these distributions, students can better approach their distribution theory assignment and grasp essential statistical principles. We'll discuss the conditions under which variance is finite or infinite in the t-distribution and compare it with the constant variance in the normal distribution. This knowledge will empower students to handle various statistics problems effectively, providing a strong foundation for further statistical learning and application.
Var(X)=E[(X−μ)^2]
where E denotes the expected value, X is the random variable, and μ is the mean of X. Variance is crucial for understanding the dispersion and reliability of data.
The t-Distribution
The t-distribution, or Student's t-distribution, is used when estimating the mean of a normally distributed population when the sample size is small, and the population standard deviation is unknown. It is characterized by its degrees of freedom (n), which typically equals the sample size minus one.
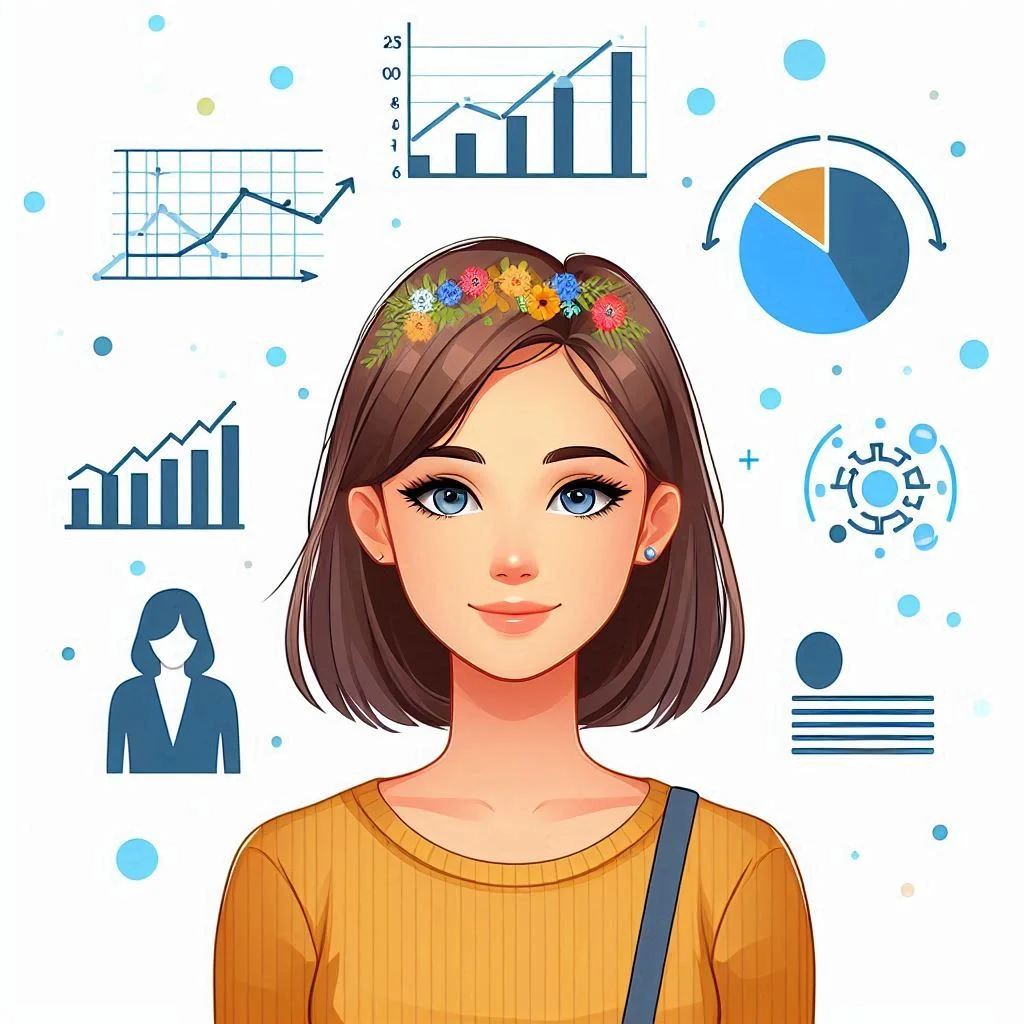
Variance in the t-Distribution
The variance of the t-distribution depends on its degrees of freedom. Specifically:
What is Variance?
Variance measures how much the values of a random variable differ from the mean. It is calculated as follows:
- Finite Variance: When n>2, the variance of the t-distribution is finite.
- Infinite Variance: When n≤2, the variance becomes infinite, indicating an undefined spread.
The formula for the variance of the t-distribution when it is finite is:
Var(T_n)=n/(n−2)
The Normal Distribution
The normal distribution, also known as the Gaussian distribution, is a continuous probability distribution characterized by its mean (μ) and standard deviation (σ). It is symmetric and forms a bell-shaped curve.
Variance in the Normal Distribution
The variance of a normal distribution is constant and finite. For a standard normal distribution N(0,1) (mean = 0, standard deviation = 1), the variance is:
Var(X)=1
For a general normal distribution N(μ,σ2):
Var(X)=σ^2
Key Differences Between t-Distribution and Normal Distribution
1. Shape and Spread:
- The t-distribution has heavier tails and is more spread out compared to the normal distribution, especially for small sample sizes.
- As the degrees of freedom increase, the t-distribution approaches the shape of the normal distribution.
2. Variance Behavior:
- In the normal distribution, variance is constant and finite.
- In the t-distribution, variance depends on the degrees of freedom and is only finite when n>2.
3. Practical Use:
- The t-distribution is used for hypothesis testing and confidence intervals in small samples.
- For larger samples, the normal distribution can be used due to the Central Limit Theorem.
Practical Steps for Solving These Assignments
To solve assignments involving variance in t-distribution and normal distribution, follow these steps: identify the distribution type, determine the degrees of freedom, apply the appropriate variance formula, and analyze the results. This methodical approach ensures accurate understanding and application, aiding in effectively tackling various statistics problems with confidence and precision.
1. Identify the Distribution:
Identifying the distribution is the first and most critical step in solving statistical assignments involving variance. This involves understanding the context of the problem and recognizing which distribution is appropriate for the data at hand. Here's how to proceed:
a. Understand the Context
Examine the problem statement carefully to determine the nature of the data and the requirements of the analysis. Pay attention to details such as sample size, known parameters (mean and standard deviation), and the type of inference being made (e.g., estimating a population mean, testing a hypothesis).
b. Determine Sample Size and Population Standard Deviation
- Small Sample Size and Unknown Population Standard Deviation: If the sample size is small (typically n < 30) and the population standard deviation is unknown, the t-distribution is usually appropriate. This scenario often arises in real-world applications where obtaining large samples is challenging.
- Large Sample Size or Known Population Standard Deviation: If the sample size is large (n ≥ 30) or the population standard deviation is known, the normal distribution is often suitable.
c. Hypothesis Testing and Confidence Intervals
- T-Distribution: Used for hypothesis testing and constructing confidence intervals for the mean when dealing with small sample sizes and unknown population standard deviation. The t-distribution accounts for additional uncertainty introduced by estimating the standard deviation from the sample.
- Normal Distribution: Applied in hypothesis testing and confidence intervals for large samples or when the population standard deviation is known. The normal distribution provides a precise and straightforward analysis due to its well-defined properties.
d. Check for Assumptions
Ensure that the data meet the assumptions required for the chosen distribution:
- T-Distribution: Assumes the data are approximately normally distributed, especially important for small sample sizes. If the data significantly deviate from normality, consider using a different method or transforming the data.
- Normal Distribution: Assumes that the data or the sampling distribution of the sample mean is normally distributed. For large samples, this assumption is generally satisfied due to the Central Limit Theorem.
By accurately identifying the appropriate distribution, you set the foundation for correctly applying the subsequent steps in the statistical analysis, leading to reliable and valid results. This step ensures that the chosen methods are suitable for the data and the problem context, enhancing the accuracy and credibility of your conclusions.
2. Determine Degrees of Freedom:
Degrees of freedom (df) are a critical concept in statistics, particularly when dealing with the t-distribution. Here’s how you determine them:
t-Distribution:
- Definition: Degrees of freedom in a t-distribution are typically n−1, where n is the sample size.
- Calculation: For a given problem, if n represents the sample size, then degrees of freedom df=n−1.
- Importance: Degrees of freedom dictate the shape and behavior of the t-distribution. A larger sample size (higher n) results in more degrees of freedom, leading the t-distribution to approximate the normal distribution.
Example:
- If you have a sample size n=10, the degrees of freedom df=10−1=9.
- For n=5, degrees of freedom df=5−1=4.
Understanding degrees of freedom helps in applying the correct variance formula and interpreting statistical results accurately. In contrast, the normal distribution’s degrees of freedom concept isn’t directly applicable, as it’s primarily used for the t-distribution in smaller sample sizes.
3. Apply the Correct Formula:
Once you have identified whether the problem involves a t-distribution or a normal distribution and determined the degrees of freedom for the t-distribution, the next step is to apply the correct variance formula.
For the t-Distribution:
The variance of a t-distributed random variable T with n degrees of freedom is given by:
Var(Tn)=n/(n−2) for n>2
This formula applies specifically when n>2, ensuring that the variance remains finite. It arises from the properties of the t-distribution and is crucial for accurately assessing the spread of data in small sample sizes.
Example Application: If your assignment involves calculating the variance of a t-distributed variable with n=10, you would use:
Var(T10)=10/(10−2)=10/8=1.25
This step ensures that you apply the correct statistical tool to analyze data reliability and significance in hypothesis testing and confidence intervals.
For the Normal Distribution:
The variance of a normal distribution X with mean μ and variance σ^2 is straightforward:
Var(X)=σ^2
This formula applies universally to normal distributions, regardless of mean or specific distribution parameters. It signifies the consistency in data dispersion typical of the normal curve.
Example Application: For a standard normal distribution N(0,1), the variance is:
Var(X)=1
This simplicity allows for straightforward interpretation and comparison across different datasets and scenarios.
By mastering these formulas and their applications, students develop a robust foundation in statistical methodology, empowering them to handle diverse statistical problems effectively and confidently.
4. Analyze the Results:
After calculating the variance for either the t-distribution or the normal distribution, it's crucial to interpret and analyze the results within the context of the problem:
- Interpretation: Understand what the variance value signifies. In the t-distribution, a finite variance (when n>2) indicates a measurable spread of data points around the mean, influenced by the degrees of freedom. An infinite variance (when n≤2n) suggests that the data points are too widely dispersed relative to the sample size, making precise estimation challenging.
- Comparison: Compare the variance values between the t-distribution and the normal distribution. Note how the t-distribution's variance changes with varying degrees of freedom, emphasizing its sensitivity to sample size. Contrast this with the constant variance of 1 in the standard normal distribution.
- Practical Implications: Discuss how variance impacts statistical inference and decision-making. For instance, in hypothesis testing or confidence interval construction, understanding the distribution's variance helps determine the reliability and precision of the estimates. A lower variance generally indicates tighter clustering of data around the mean, implying greater confidence in the results.
- Real-World Application: Relate the computed variance to practical scenarios. For example, in medical research, understanding the variance in patient responses to a treatment can influence treatment efficacy assessments. Similarly, in financial analysis, variance helps gauge risk and volatility in investment returns.
By thoroughly analyzing the variance results, students gain deeper insights into the behavior of different distributions, enhancing their ability to apply statistical concepts effectively in diverse academic and professional contexts.
Example Application
Suppose you encounter an assignment where you need to compare the variance of a random variable T following a t-distribution with n degrees of freedom and a random variable X following a standard normal distribution N(0,1):
1. t-Distribution:
- Identify n. If n>2, calculate the variance using n/(n−2).If n≤2, note that the variance is infinite.
2. Normal Distribution:
- Recognize that the variance is 1 for N(0,1).
3. Comparison:
- Discuss how the finite/infinite nature of the t-distribution's variance affects its application compared to the normal distribution.
By understanding these concepts and following these steps, students can confidently approach assignments involving variance in the t-distribution and normal distribution, enhancing their statistical analysis skills and comprehension.
Conclusion
Understanding the behavior of variance in the t-distribution and normal distribution is crucial for tackling statistics assignments. The t-distribution's variance depends on degrees of freedom and is finite only when n>2. In contrast, the normal distribution has a constant, finite variance. Recognizing these differences helps students apply the appropriate distribution based on sample size and known parameters. By following the outlined steps—identifying the distribution, determining degrees of freedom, applying the correct formula, and analyzing results—students can confidently solve related problems. This foundational knowledge enhances statistical analysis skills, making it easier to approach a wide range of assignments and real-world applications.