Handling Unbalanced Designs in Factorial ANOVA: Type I and Type III Sums of Squares Explained
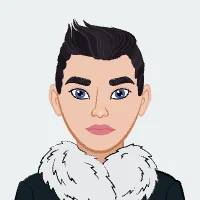
Understanding and conducting factorial ANOVA is essential for students tackling assignments involving multiple factors and their interactions. One of the key challenges is handling unbalanced designs, where the sample sizes across groups are unequal. This imbalance can complicate your analysis and interpretation, making it crucial to employ the right techniques. In this comprehensive guide, we delve into the intricacies of unbalanced designs in factorial ANOVA and explore the issues related to sums of squares (SS). We will cover the two primary approaches for addressing these challenges: Type I SS (Sequential SS) and Type III SS. By grasping these concepts, you'll be better equipped to solve your statistics assignment effectively, ensuring accurate and meaningful results. Whether you are a beginner or have some experience with ANOVA, this guide will provide you with the essential knowledge to navigate unbalanced designs confidently.
Introduction to Factorial ANOVA
Factorial ANOVA (Analysis of Variance) is a statistical method used to examine the influence of two or more categorical independent variables on a continuous dependent variable. It also allows for the investigation of interactions between the factors. This method is powerful for understanding how different factors and their combinations affect outcomes. However, when the groups involved in the analysis have unequal sample sizes, known as an unbalanced design, the analysis becomes more complex.
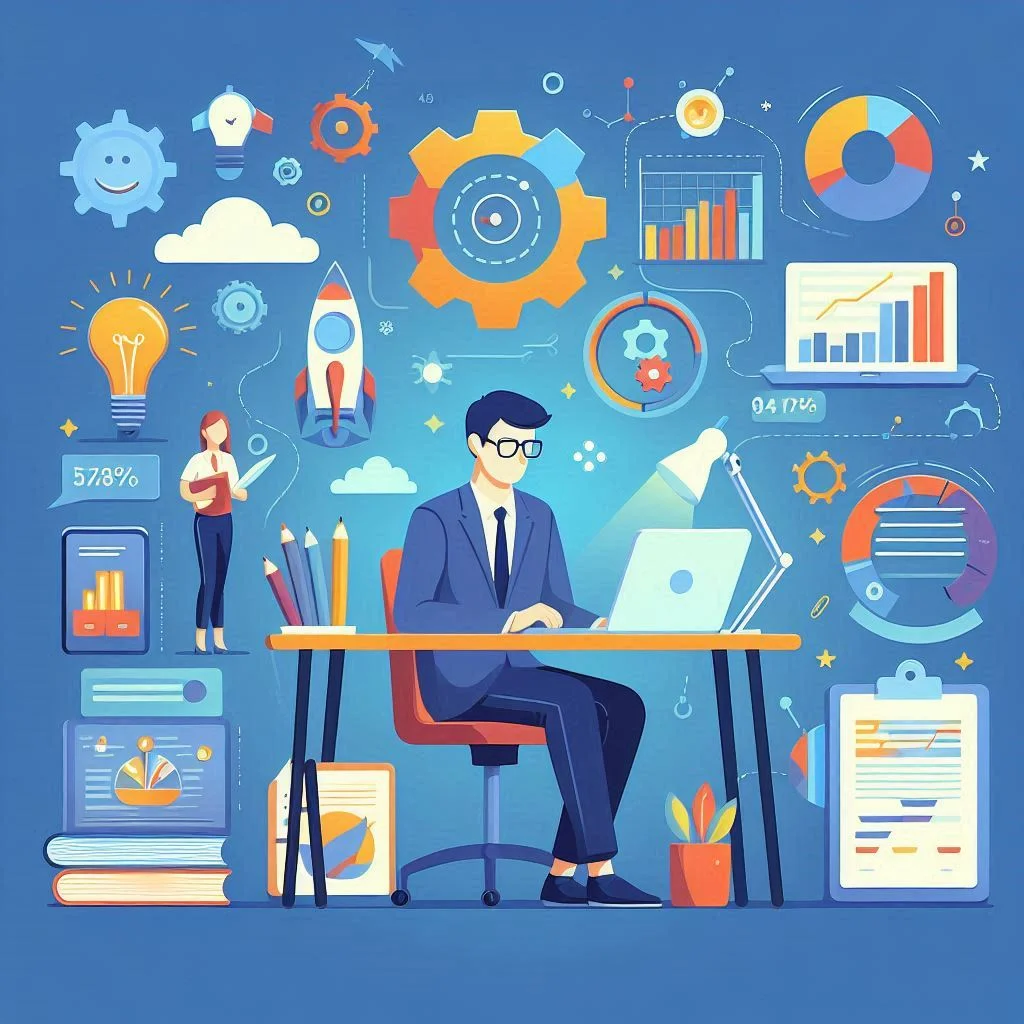
Understanding Unbalanced Designs
In a balanced design, each group or condition has the same number of observations. This uniformity simplifies the calculations and interpretation of results. In contrast, unbalanced designs occur when the number of observations in each group varies. This imbalance can arise due to various reasons, such as data collection constraints, missing data, or natural variations in the sample sizes.
Unbalanced designs present several challenges:
- Complexity in Calculations: The unequal sample sizes make the partitioning of sums of squares (SS) more complex.
- Potential Bias: If not handled correctly, unbalanced designs can introduce bias in the estimation of effects.
- Interpretation Difficulties: The interaction effects and main effects can be more difficult to interpret in the presence of unequal sample sizes.
Issues with Sums of Squares (SS) in Unbalanced Designs
In ANOVA, the total variation in the dependent variable is partitioned into sums of squares attributable to different sources: main effects, interactions, and error. In balanced designs, this partitioning is straightforward and unambiguous. However, in unbalanced designs, the partitioning of SS becomes problematic.
Why is SS Calculation Problematic in Unbalanced Designs?
In unbalanced designs, the contribution of each factor to the total variation is not as clear-cut. The presence of unequal sample sizes can lead to ambiguity in attributing the variation to specific factors. This is because the sums of squares are not orthogonal, meaning that the variation explained by one factor may overlap with the variation explained by another factor.
Approaches to Calculating Sums of Squares in Unbalanced Designs
To address the complexities introduced by unbalanced designs, several approaches to calculating sums of squares have been developed. The two most common are Type I SS (Sequential SS) and Type III SS.
Type I Sums of Squares (Sequential SS)
Explanation: Type I SS, also known as Sequential SS, calculates the sums of squares sequentially. Each factor is adjusted for the preceding factors in the model. This means the order in which factors are entered into the model matters significantly. The first factor is adjusted only for the grand mean, the second factor is adjusted for the grand mean and the first factor, and so on.
Advantages:
- Simplicity: Type I SS is relatively straightforward to calculate.
- Interpretability in Specific Contexts: This approach is useful when there is a logical or theoretical order to the factors. For example, in an experimental design where factors are introduced sequentially, Type I SS can provide meaningful insights.
Disadvantages:
- Order Sensitivity: The results can vary depending on the order in which factors are entered into the model. This can lead to misleading conclusions if the order is not carefully considered.
- Not Suitable for Interaction Interpretation: Type I SS is not ideal for interpreting interaction effects because it does not adjust for all other factors simultaneously.
Example: Consider a study examining the effect of two factors, A and B, on a dependent variable. If Factor A is entered into the model first, the SS for Factor A will be calculated without considering Factor B. When Factor B is entered, its SS will be calculated considering Factor A. The interpretation of results will depend heavily on this order.
Type III Sums of Squares
Explanation: Type III SS calculates the sums of squares by adjusting each factor for all other factors in the model, regardless of the order in which they are entered. This approach simultaneously considers all factors, making it the most commonly recommended method for unbalanced designs.
Advantages:
- Order Independence: Type III SS is not affected by the order of factors in the model. This makes the results more robust and interpretable.
- Suitable for Interaction Interpretation: This approach is better suited for interpreting interaction effects because it adjusts for all other factors simultaneously.
Disadvantages:
- Complexity: Type III SS calculations are more complex than Type I SS.
- Software Dependency: Accurate computation of Type III SS often relies on statistical software.
Example: Using the same study examining the effect of two factors, A and B, on a dependent variable, Type III SS would calculate the SS for Factor A by adjusting for Factor B and vice versa. This simultaneous adjustment provides a more accurate estimate of the effects of each factor and their interaction.
Preferred Approach and Reasons
In most cases, Type III SS is preferred for analyzing unbalanced designs in factorial ANOVA. The main reasons are:
- Order Independence: Type III SS provides consistent results regardless of the order in which factors are entered into the model. This reduces the potential for bias and misinterpretation.
- Simultaneous Adjustment: By adjusting each factor for all other factors simultaneously, Type III SS offers a more accurate estimate of main effects and interactions. This is particularly important in complex designs with multiple factors and interactions.
- Robustness: Type III SS is generally more robust and reliable for analyzing unbalanced designs, making it the preferred choice for most researchers and statisticians.
Practical Steps for Analyzing Unbalanced Designs
When faced with an unbalanced design in factorial ANOVA, students can follow these practical steps to ensure accurate and meaningful analysis: data preparation, choosing the right software, model specification, SS calculation, interpreting results, and reporting findings. These steps will help you complete your ANOVA assignment with precision and confidence.
1. Data Preparation:
Data preparation is a crucial first step in analyzing unbalanced designs in factorial ANOVA. Start by ensuring your data is clean and properly formatted. This includes checking for and addressing any missing values, outliers, or errors in data entry. Use descriptive statistics and visualizations to understand the distribution of your data and identify any potential issues. Additionally, ensure that your data meets the assumptions of ANOVA, such as normality, homogeneity of variances, and independence of observations. Address any violations of these assumptions appropriately, such as by transforming variables or using robust statistical techniques. Proper data preparation lays the foundation for accurate analysis, helping you solve your statistics assignment effectively and confidently.
2. Choosing the Right Software:
Selecting the appropriate statistical software is crucial for handling unbalanced designs in factorial ANOVA. Reliable software such as SPSS, R, SAS, or Python (using libraries like statsmodels) can accurately calculate Type III SS and provide robust analysis tools. Ensure the software you choose is capable of handling unbalanced designs and offers comprehensive documentation and support. Familiarize yourself with the software's features, settings, and capabilities, especially those related to ANOVA. Using reliable software will not only simplify your calculations but also enhance the accuracy and reliability of your results. This preparation is essential to successfully solve your statistics assignment and produce meaningful insights from your data analysis.
3. Model Specification:
Accurate model specification is crucial when analyzing unbalanced designs in factorial ANOVA. Start by clearly defining your independent variables (factors) and dependent variable. Ensure that all relevant main effects and interaction terms are included in the model to capture the complexity of your data. In unbalanced designs, it's essential to specify the model correctly to avoid biased estimates. Use statistical software to build your model, paying close attention to the syntax and options for handling unbalanced data. Proper model specification allows you to account for the unequal sample sizes and accurately estimate the effects of each factor and their interactions. This careful approach will help you solve your statistics assignment effectively and achieve reliable results.
4. SS Calculation:
Using statistical software to calculate sums of squares (SS) is crucial for handling unbalanced designs accurately. Ensure the software is configured to use Type III SS, which adjusts each factor for all other factors in the model, regardless of their order. This approach is essential for obtaining accurate estimates of main effects and interactions in unbalanced designs. Familiarize yourself with the software’s documentation and settings to ensure proper calculations. Double-check the output to confirm that Type III SS has been used, and review the sums of squares, degrees of freedom, F-values, and p-values provided by the software. Accurate SS calculation is fundamental to deriving meaningful insights from your ANOVA analysis.
5. Interpreting Results:
Interpreting results from a factorial ANOVA with unbalanced designs involves carefully examining the output from your analysis to understand the effects of each factor and their interactions. Begin by assessing the main effects and interaction terms to determine how each factor influences the dependent variable. Pay close attention to the sums of squares, F-values, and p-values to evaluate the significance and strength of these effects. Consider the context of your study and how the results align with your hypotheses or research questions. It’s crucial to interpret the findings in light of the unbalanced design, acknowledging any potential biases or limitations. Clear, thoughtful interpretation will ensure that your conclusions are both meaningful and actionable.
6. Reporting Results:
Accurate reporting of results is crucial for effectively communicating your findings in factorial ANOVA. Begin by clearly presenting the sums of squares (SS), degrees of freedom, F-values, and p-values for each factor and interaction. Ensure that your results are organized in a way that highlights the main effects and interactions of interest. Include detailed tables and charts where appropriate, and provide a thorough interpretation of these results in the context of your study. Address any significant findings and discuss their implications, considering both practical and theoretical aspects. Effective reporting not only demonstrates your analytical skills but also helps in drawing clear conclusions and making informed decisions based on your data.
Tips for Students
To excel in analyzing unbalanced designs, ensure you understand factorial ANOVA concepts thoroughly. Use reliable statistical software and verify that it handles unbalanced designs correctly. Don’t hesitate to consult resources or seek help if you encounter challenges during your analysis.
1. Understand the Theory:
Grasping the theory behind factorial ANOVA and unbalanced designs is essential for accurate analysis. Familiarize yourself with the fundamental principles, including how sums of squares are calculated and the implications of unbalanced designs. This foundational knowledge will help you make informed decisions about which methods to use and how to interpret your results effectively. A solid understanding of the theory ensures more reliable and insightful analysis.
2. Use Reliable Software:
Choosing the right statistical software is crucial for accurate analysis of unbalanced designs in factorial ANOVA. Opt for software with robust capabilities for handling unbalanced data and calculating sums of squares, such as SPSS, R, or SAS. Familiarize yourself with the software’s features and settings to ensure correct implementation of Type III sums of squares. Reliable software will streamline your analysis and enhance the accuracy of your results.
3. Check Assumptions:
Verifying the assumptions of ANOVA is critical for valid results. Ensure your data meets the assumptions of normality, homogeneity of variances, and independence of observations. Normality can be checked using plots or statistical tests, while homogeneity can be assessed with tests like Levene’s test. Address any violations by transforming data or using robust statistical methods to ensure reliable and accurate analysis.
4. Seek Help:
If you find yourself struggling with unbalanced designs or any aspect of factorial ANOVA, consider reaching out for assistance. ANOVA assignment help services can provide valuable support and guidance, offering expert advice tailored to your specific needs. Whether you need clarification on concepts or help with calculations, leveraging these services can enhance your understanding and improve your analysis, ensuring more accurate and reliable results.
Conclusion
Handling unbalanced designs in factorial ANOVA requires careful consideration and the right approach to calculating sums of squares. While Type I SS can be useful in specific contexts, Type III SS is generally preferred for its robustness, order independence, and suitability for interpreting interactions. By following practical steps and leveraging reliable software, you can effectively analyze unbalanced designs and derive meaningful insights from your data. Understanding these concepts and techniques will not only help you tackle and solve your statistics assignment but also prepare you for real-world research scenarios where unbalanced designs are common. With a solid grasp of factorial ANOVA and the challenges of unbalanced designs, you’ll be well-equipped to conduct rigorous and insightful analyses, ensuring that your findings are both accurate and reliable.