Understanding Confidence Intervals and Coverage Probability in Normal Distributions
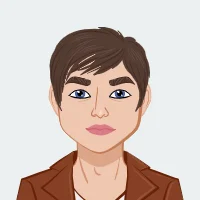
Solving your statistics assignment can be a daunting task, especially when it involves understanding and calculating confidence intervals. A common scenario you might encounter is determining the probability that a given interval will cover an unknown population parameter, such as the mean. This type of problem requires a solid grasp of normal distributions, sample means, and standard deviations. By mastering these concepts, you can confidently approach any statistics assignment that requires interval estimation. This blog will guide you through the process of calculating the coverage probability of confidence intervals, providing you with the tools and techniques needed to complete your distribution theory assignments. Whether you're dealing with a small sample size or a larger dataset, the principles discussed here will help you solve your statistics assignment efficiently and accurately.
Step-by-Step Guide for Solving Statistics Assignments
When calculating the coverage probability of a confidence interval for the mean of a normal distribution, follow these steps: understand the interval, transform the sample mean to a standard normal variable, and use statistical tables or software. This approach simplifies complex problems, making it easier to solve your statistics assignment accurately.
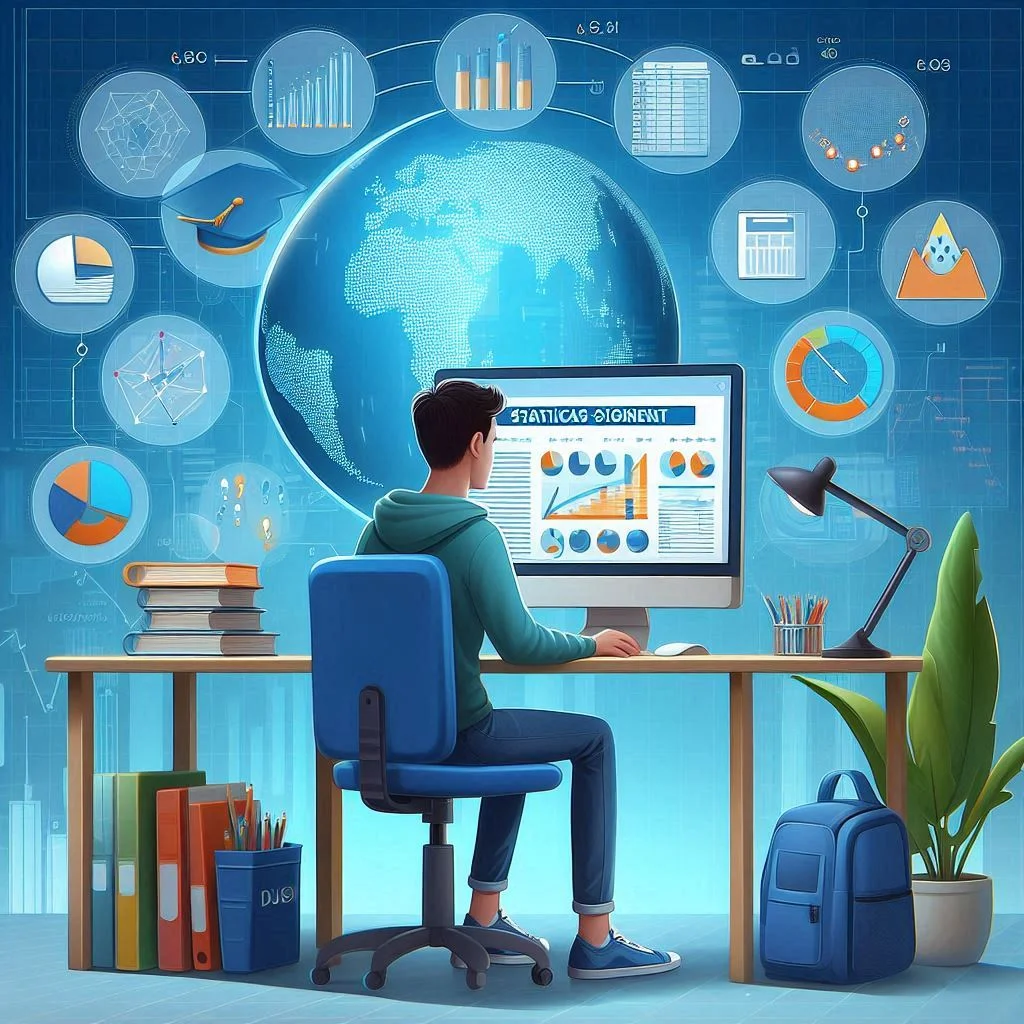
Step 1: Understanding the Interval:
The first step in solving a statistics assignment involving confidence intervals is to understand what a confidence interval represents and how it is constructed. A confidence interval provides a range of values within which the true population parameter, such as the mean (μ), is expected to lie with a certain level of confidence.
Key Components of a Confidence Interval:
- Sample Mean (Xˉ): The sample mean is the average of the data points in your sample. It serves as the central point of the confidence interval.
- Margin of Error: The margin of error reflects the range within which the true population parameter is expected to fall. It accounts for the variability in the sample and the desired confidence level.
- Standard Deviation (σ): The standard deviation measures the dispersion of the sample data. In cases where the population standard deviation is unknown, the sample standard deviation (s) is used.
- Sample Size (n): The sample size impacts the width of the confidence interval. Larger samples provide more precise estimates of the population parameter, resulting in narrower intervals.
- Confidence Level: The confidence level, often expressed as a percentage (e.g., 95%), indicates the degree of certainty that the interval contains the true population parameter. Higher confidence levels result in wider intervals.
Understanding these components and how they interact is crucial for accurately constructing and interpreting confidence intervals. This foundational knowledge will help you solve your statistics assignment more effectively.
Step 2: Normal Distribution and Sample Mean:
Understanding the relationship between the normal distribution and the sample mean is essential when working with confidence intervals in statistics. This step involves recognizing how the properties of the normal distribution apply to the sample mean and how this knowledge helps in constructing confidence intervals.
The Normal Distribution:
- Definition: The normal distribution, also known as the Gaussian distribution, is a continuous probability distribution characterized by its bell-shaped curve. It is defined by two parameters: the mean (μ) and the standard deviation (σ).
- Properties:
- Symmetry: The normal distribution is symmetric about its mean.
- 68-95-99.7 Rule: Approximately 68% of the data falls within one standard deviation of the mean, 95% within two, and 99.7% within three.
Sample Mean and Its Distribution:
- Sample Mean (Xˉ): The sample mean is the average of a set of observations drawn from the population. It serves as an estimate of the population mean.
- Distribution of the Sample Mean: When you draw multiple samples of size n from a population, the sample means will themselves form a distribution. According to the Central Limit Theorem, regardless of the population's distribution, the distribution of the sample means will be approximately normal if the sample size is sufficiently large (typically n>30).
- Mean and Standard Deviation of the Sample Mean:
- Mean: The mean of the sample mean distribution is equal to the population mean (μ).
- Standard Deviation: The standard deviation of the sample mean distribution, also known as the standard error, is given by σ\sqrt{n}, where σ is the population standard deviation and n is the sample size.
Step 3: Transformation to Standard Normal Distribution:
Transforming the sample mean to a standard normal distribution is a crucial step in solving statistics assignments involving confidence intervals for the mean of a normal distribution. This transformation simplifies the process of finding probabilities and constructing confidence intervals by leveraging the well-known properties of the standard normal distribution.
Standard Normal Distribution:
The standard normal distribution, denoted as N(0,1), is a special case of the normal distribution with a mean of 0 and a standard deviation of 1. It is used as a reference to convert any normal distribution to a standard form, allowing for easier calculation of probabilities.
Transformation Process:
1. Standardizing the Sample Mean: To transform the sample mean (Xˉ) to a standard normal variable (Z), we use the following formula:
Z=(Xˉ−μ)/σsqrt{n}
Where:
- Xˉ is the sample mean.
- μ is the population mean.
- σ is the population standard deviation.
- on is the sample size.
2. Interpreting Z-Score: The Z-score represents the number of standard errors that the sample mean (Xˉ) is away from the population mean (μ). It standardizes the distribution of the sample mean, allowing us to use standard normal distribution tables to find probabilities and critical values.
3. Using Z-Score for Confidence Intervals: Once the sample mean is transformed into the Z-score, we can determine the probability that a given interval will cover the population mean (μ) by looking up the Z-score in standard normal distribution tables.
Practical Steps in Assignments:
- Calculate the Sample Mean: Begin by calculating the sample mean (Xˉ) from your data.
- Determine the Standard Error: Compute the standard error of the sample mean using σsqrt{n}}.
- Standardize the Sample Mean: Use the formula Z=(Xˉ−μ)/σsqrt{n} to convert the sample mean to a Z-score.
- Use Z-Tables or Software: Refer to Z-tables or statistical software to find the probabilities associated with the calculated Z-score. This helps in determining the confidence interval and its coverage probability.
- Construct the Confidence Interval: Based on the Z-score and the desired confidence level, construct the confidence interval for the population mean.
By transforming the sample mean to a standard normal distribution, you simplify the process of finding probabilities and constructing confidence intervals. This approach is fundamental in statistics and is essential for solving your statistics assignment accurately and efficiently. Understanding this transformation allows you to leverage the standard normal distribution's properties, making complex statistical problems more manageable.
Step 4: Setting Up the Probability:
Setting up the probability in statistics assignments involves defining the interval within which we expect the unknown population parameter to lie with a specified level of confidence. This step focuses on interpreting the confidence interval and determining the probability that it covers the true population mean (μ).
Confidence Interval Definition:
- Interval Definition: A confidence interval is a range of values constructed from sample data that is likely to contain the true population parameter, such as the mean (μ), with a certain level of confidence. For example, a 95% confidence interval implies that if we were to take 100 different samples and construct confidence intervals in the same way, approximately 95 of those intervals would contain the true population mean.
- Components:
- Sample Mean (Xˉ): The central point of the confidence interval, estimated from sample data.
- Margin of Error: The range around the sample mean that accounts for sampling variability and is determined by the standard error.
- Confidence Level: The probability (expressed as a percentage) that the interval will contain the true population parameter.
Probability Setup:
1. Formulating the Interval: To set up the probability, we define the confidence interval as:
(Xˉ−z(σ/{sqrt{n}}, Xˉ+znσ/{sqrt{n})
Where:
- X is the sample mean.
- z is the critical value from the standard normal distribution corresponding to the desired confidence level (e.g., 1.96 for 95% confidence).
- σ is the population standard deviation (or sample standard deviation if unknown).
- n is the sample size.
2. Interpreting the Probability: The probability is interpreted as the likelihood that this interval contains the true population mean (μ). For instance, a 95% confidence interval suggests that there is a 95% probability that the interval covers the true value of μ.
3. Using Statistical Tables or Software: Statistical tables or software are employed to find the critical value zzz corresponding to the desired confidence level. This critical value determines the width of the interval and ensures that the specified confidence level is achieved.
By setting up the probability in this manner, you ensure that your confidence intervals are accurately constructed and interpreted in statistics assignments. This approach provides a clear framework for estimating population parameters from sample data and enhances your ability to solve your statistics assignment with confidence and precision.
Step 5: Expressing the Interval in Terms of Z:
Expressing the confidence interval in terms of the standard normal variable Z is a fundamental step in solving statistics assignments involving interval estimation for the mean of a normal distribution. This transformation allows us to leverage the properties of the standard normal distribution to determine the probability that the interval covers the unknown population parameter.
Transforming the Confidence Interval:
1. Standardizing the Interval: To express the confidence interval in terms of Z, we use the formula:
Z=(Xˉ−μ)σ{sqrt[n]}
Where:
- Xˉ is the sample mean.
- μ is the population mean.
- σ is the population standard deviation (or sample standard deviation if unknown).
- n is the sample size.
2. Interpreting the Z-Score: The Z-score represents the number of standard deviations that the sample mean (Xˉ) is away from the population mean (μ). It allows us to standardize the distribution of the sample mean to a standard normal distribution N(0,1).
3. Setting Up the Interval: Once Z is calculated, the confidence interval can be expressed as:
(Xˉ−Z⋅σsqrt{n}, Xˉ+Z⋅σsqrt{n})
This interval represents the range within which we are confident that the true population mean (μ) lies, based on the sample data.
By expressing the confidence interval in terms of Z, you transform the problem into one that can be readily addressed using standard normal distribution tables or statistical software. This approach simplifies the calculation of probabilities and enhances your ability to accurately solve your statistics assignment involving interval estimation.
Step 6: Simplifying the Probability Expression:
Simplifying the probability expression involves refining the calculation to determine the likelihood that a confidence interval covers the unknown population parameter, such as the mean (μ), in statistics assignments. This step focuses on using the properties of the standard normal distribution to derive a straightforward probability statement.
Refining the Probability Calculation:
1. Using the Standard Normal Variable Z: Once the confidence interval is expressed in terms of the standard normal variable Z, we can simplify the probability expression. Recall that Z is calculated as:
Z=(Xˉ−μ)σ{sqrt[n]}
Where:
- Xˉ is the sample mean.
- μ is the population mean.
- σ is the population standard deviation (or sample standard deviation if unknown).
- n is the sample size.
2. Probability Interpretation: The probability that the confidence interval covers the true population mean μ can be interpreted as the area under the standard normal curve between two Z-scores, corresponding to the lower and upper bounds of the interval.
3. Critical Values Z_α/2: For a confidence level 1−α, where α is the significance level (e.g., α=0.05 for 95% confidence), the critical values Zα_/2 are determined from standard normal distribution tables or statistical software. These values divide the area under the curve, leaving 1−α in the middle.
This interval represents the range within which we are 1−α confident that the true population mean μ lies.
Step 7: Calculating the Coverage Probability:
Calculating the coverage probability involves determining the likelihood that a confidence interval constructed from sample data covers the unknown population parameter, such as the mean (μ), in statistics assignments. This step focuses on applying the critical values of the standard normal distribution to quantify the interval's effectiveness in capturing the true population mean.
Calculating the Probability:
- Interpreting Coverage Probability: The coverage probability is the proportion of times that the confidence interval constructed in this manner will contain the true population mean μ, over an infinite number of repeated samples.
Simplifying this gives you the interval within which you can be 95% confident that the true population mean μ lies.
- Coverage Interpretation: In practice, if you were to repeat the sampling process many times and construct confidence intervals in the same manner, approximately 95% of those intervals would contain the true population mean μ. This illustrates the effectiveness and reliability of the confidence interval in capturing the population parameter.
By calculating the coverage probability in this structured approach, you ensure that your confidence intervals are statistically valid and provide meaningful estimates of the population parameter in statistics assignments. This methodological approach enhances your ability to interpret and apply confidence intervals effectively, contributing to accurate solutions in your statistics assignment tasks.
Conclusion
Understanding how to calculate the coverage probability of confidence intervals is a crucial skill for anyone looking to solve their statistics assignment effectively. By breaking down the problem into manageable steps, such as transforming variables and using standard normal distribution tables, you can simplify even the most complex assignments. Remember, the key to success in statistics is practice and familiarity with the underlying concepts. As you continue to work on these types of problems, you'll find that your confidence grows, and your ability to solve your statistics assignment improves significantly. Use this guide as a reference, and don't hesitate to seek additional resources or help if needed. With dedication and the right approach, you'll be well-equipped to handle any statistical challenge that comes your way.