Demystifying the One-Sample t-Test: A Practical Approach to Solving Statistics Assignments
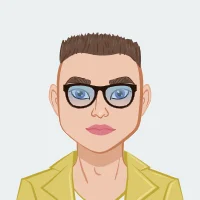
In the realm of statistical analysis, mastering tools like the one-sample t-test is pivotal for effectively interpreting data and making informed decisions. Whether you're examining sample means against population benchmarks or testing hypotheses, understanding this test empowers you to navigate complexities in research and t-test assignments. This blog serves as your comprehensive guide to mastering the one-sample t-test, offering step-by-step insights that will equip you to confidently solve your statistics assignments. By delving into its principles, applications, and interpretation, you'll gain the proficiency needed to apply this fundamental statistical tool across various scenarios, ensuring robust analyses and precise conclusions.
1. Understanding the One-Sample t-Test:
The one-sample t-test is a statistical procedure used to determine whether the mean of a single sample is significantly different from a known or hypothesized population mean. This test is particularly useful in scenarios where you have a sample and wish to compare its mean to a standard or expected value, such as in quality control, research studies, or academic assignments.
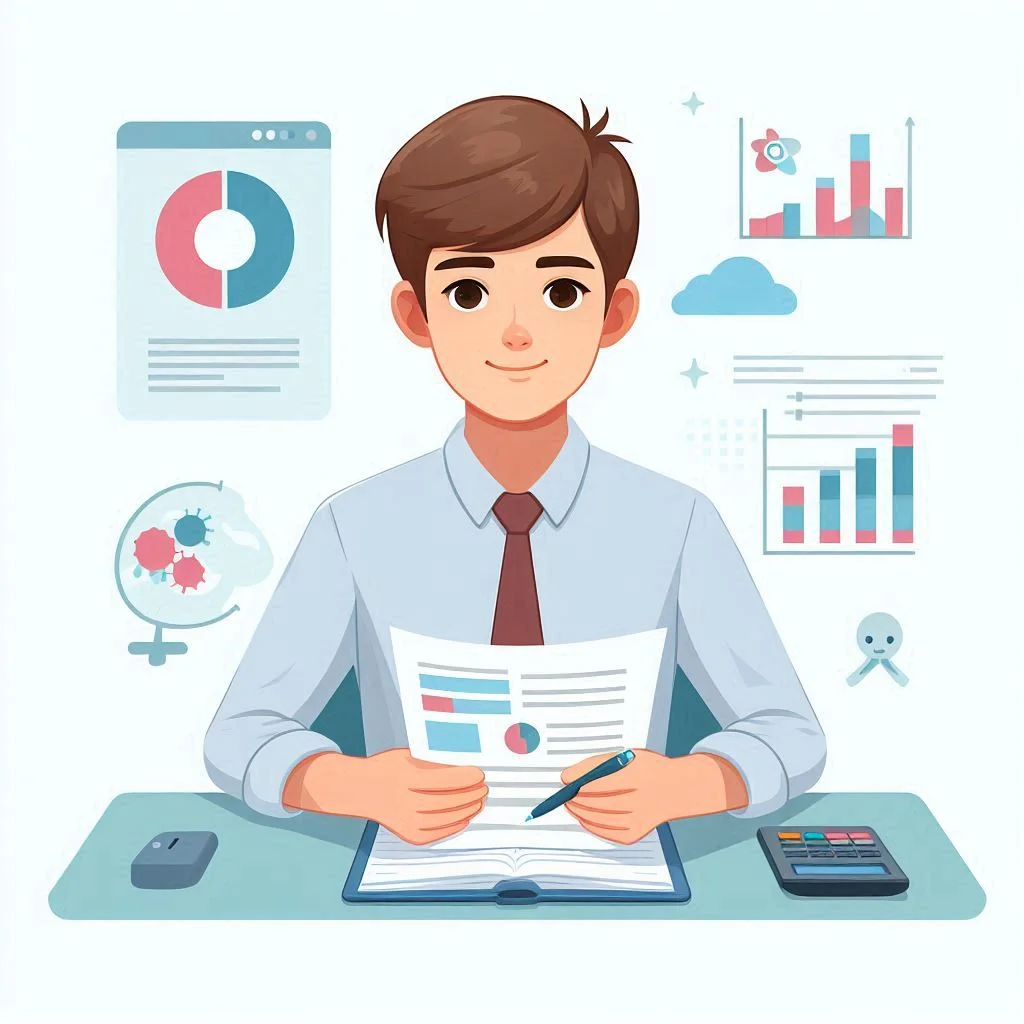
Key Concepts:
1. Purpose: The primary purpose of the one-sample t-test is to assess whether there is evidence to reject the null hypothesis that the sample mean is equal to a specified population mean. It helps in determining if any observed differences between the sample mean and the population mean are statistically significant or simply due to random chance.
2. Assumptions: Before conducting a one-sample t-test, it's crucial to ensure that the data meet certain assumptions. These include:
- Normality: The data should be approximately normally distributed.
- Independence: Data points should be independent of each other.
- Random Sampling: The sample should be randomly selected from the population of interest.
3. Formulating Hypotheses: In the context of the one-sample t-test:
- Null Hypothesis (H₀): This states that there is no significant difference between the sample mean and the population mean. It typically assumes equality, e.g., H₀: μ = μ₀.
- Alternative Hypothesis (H₁): This states that there is a significant difference between the sample mean and the population mean. It can be directional (e.g., μ > μ₀ or μ < μ₀) or non-directional (e.g., μ ≠ μ₀).
4. Calculating the t-statistic: The t-statistic measures how many standard errors the sample mean is away from the hypothesized population mean, accounting for sample size and variability. It is calculated using the formula:
t=(xˉ−μ0)/[s/sqrt(n)]
where:
- xˉ is the sample mean,
- μ₀ is the hypothesized population mean,
- s is the sample standard deviation,
- n is the sample size.
5. Interpreting Results: Once the t-statistic is calculated, it is compared to a critical value from the t-distribution or evaluated with a p-value to determine statistical significance. A smaller p-value indicates stronger evidence against the null hypothesis, suggesting that the sample mean differs significantly from the population mean.
Understanding these fundamental aspects of the one-sample t-test lays a solid foundation for effectively applying this statistical tool in solving various types of statistics assignments. By grasping its purpose, assumptions, hypothesis formulation, calculation, and result interpretation, you will be well-equipped to navigate and excel in statistical analyses related to mean comparisons in both academic and practical contexts.
2. Steps to Conduct a One-Sample t-Test:
To conduct a one-sample t-test, start by formulating clear hypotheses comparing your sample mean to a known population mean. Use statistical software like JMP or SPSS to input your data and execute the test. Interpret the resulting t-statistic and p-value to determine if your sample mean significantly differs from the population mean, ensuring robust conclusions in statistical analysis.
Step 1: Formulate Hypotheses:
In statistics, hypotheses are statements used to make assertions about a population based on sample data. Formulating clear and precise hypotheses is crucial before conducting any statistical test, including the one-sample t-test. Let’s delve into the steps involved:
a. Null Hypothesis (H₀):
The null hypothesis (H₀) states that there is no significant difference between the sample mean (xˉ) and a hypothesized population mean (μ₀). It represents the default position that there is no effect or relationship in the population being studied.
Example: If we are testing whether the average age of a population is 50 years, the null hypothesis would be: H₀: xˉ=50
b. Alternative Hypothesis (H₁):
The alternative hypothesis (H₁), sometimes referred to as the research hypothesis, proposes that there is a significant difference between the sample mean and the hypothesized population mean. It indicates the possibility of an effect or relationship in the population.
Example: The alternative hypothesis for the average age example can be: H₁ :xˉ=50
This two-tailed alternative hypothesis suggests that we are testing if the average age of the population is either significantly different from 50 years in either direction.
Key Considerations:
- Directionality: Depending on the nature of the research question, the alternative hypothesis can be one-tailed (directional) or two-tailed (non-directional).
- Precision: Hypotheses should be formulated with specific population parameters and clear expectations regarding their relationship to the sample data.
Step 2: Collect and Prepare Data:
Collecting and preparing data is a crucial step in conducting a one-sample t-test, ensuring that the sample accurately represents the population of interest and meets the assumptions necessary for valid statistical analysis.
1. Define the Population and Sample:
- Population: Identify the entire group or set of individuals, items, or data points that you want to make inferences about. For example, if you're studying the average age of adults in a city, the population would be all adults in that city.
- Sample: Select a subset of the population that will be included in your study. The sample should ideally be representative of the population to ensure that conclusions drawn from the sample can be generalized to the larger population.
2. Sampling Methods:
- Random Sampling: Ensure that your sample is randomly selected from the population to reduce bias and ensure representativeness. Random sampling involves each member of the population having an equal chance of being selected for the sample.
- Sample Size: Consider the size of your sample, as larger samples generally provide more reliable estimates of population parameters. The adequacy of the sample size depends on factors such as the variability of the population and the desired level of precision.
3. Data Collection:
- Data Variables: Clearly define and measure the variables of interest. In the context of a one-sample t-test, you'll typically have one continuous numerical variable (e.g., age, weight) that you're testing against a population mean.
- Data Sources: Determine where and how you will collect your data. This may involve surveys, experiments, databases, or other sources depending on the nature of your study.
4. Data Preparation:
- Data Cleaning: Check for errors or inconsistencies in your data and clean them to ensure accuracy and reliability. This may involve removing outliers, correcting missing values, or standardizing formats.
- Data Organization: Organize your data in a format suitable for statistical analysis. This may include creating a spreadsheet or database that includes all necessary variables and observations.
5. Assumptions Checking:
- Normality: Assess whether your data follow a normal distribution using graphical methods (e.g., histograms, Q- plots) or statistical tests (e.g., Shapiro-Wilk test).
- Independence: Ensure that data points are independent of each other, meaning that the value of one observation does not influence the value of another.
By carefully collecting and preparing your data according to these guidelines, you set a strong foundation for conducting a one-sample t-test. This ensures that your analysis is based on reliable data that meets the assumptions necessary for valid statistical inference.
Step 3: Calculate Descriptive Statistics:
Before performing a one-sample t-test, it's essential to compute descriptive statistics from your data set. These statistics provide a summary of the sample data and are crucial for calculating the t-statistic in the next step.
1. Calculate the Sample Mean (xˉ:
The sample mean (xˉ) is the average value of the observations in your sample.
2. Calculate the Sample Standard Deviation (s):
The sample standard deviation (s) measures the dispersion or spread of the data points around the sample mean.
3. Interpretation:
- Sample Mean (xˉ): Represents the central tendency of the sample data. It is the point around which the data points are distributed.
- Sample Standard Deviation (s): Indicates the extent of variability or dispersion among the data points.
By calculating and interpreting descriptive statistics accurately, you ensure that your subsequent statistical analysis, such as the one-sample t-test, is based on a solid understanding of the sample data's distribution and characteristics.
Step 4: Set Significance Level (α):
Setting the significance level (α) is a critical step in hypothesis testing, including the one-sample t-test.
1. Definition:
- Significance Level (α): It is the threshold used to determine whether the results of a statistical test are statistically significant. It represents the maximum allowable probability of committing a Type I error, which is rejecting the null hypothesis when it is true.
2. Common Values:
- The significance level (α) is typically set at 0.05 or 5%, although other common values include 0.01 (1%) or 0.10 (10%).
- The choice of α depends on the desired level of confidence in the results and the consequences of making a Type I error.
3. Interpretation:
- If the p-value (probability value) obtained from the statistical test is less than or equal to the significance level (α), we reject the null hypothesis.
- If the p-value is greater than the significance level (α), we fail to reject the null hypothesis.
By understanding and appropriately setting the significance level (α), you establish the criteria for evaluating the strength of evidence against the null hypothesis in a one-sample t-test and other statistical analyses.
Step 5: Calculate the t-Statistic:
Calculating the t-statistic is a fundamental step in conducting a one-sample t-test. This statistic quantifies how different the sample mean (xˉ) is from the hypothesized population mean (μ₀), accounting for the variability within the sample.
1. Formula:
The t-statistic for a one-sample t-test is calculated using the following formula:
t=(xˉ− μ₀)/s[sqrt n]
Where:
- xˉ is the sample mean,
- μ₀ is the hypothesized population mean (specified in the null hypothesis),
- s is the sample standard deviation,
- n is the sample size.
2. Interpretation:
- The numerator (xˉ−μ₀) represents the difference between the sample mean and the hypothesized population mean.
- The denominator {s}{\sqrt{n}}n) is the standard error of the mean, which measures the variability of sample means around the population mean.
3. Degrees of Freedom:
- Degrees of freedom (df) for a one-sample t-test are calculated as df=n−1, where n is the sample size. Degrees of freedom are important in determining the critical value from the t-distribution table or calculating the p-value.
4. Interpretation of Results:
- Once the t-statistic is calculated, it is compared to critical values from the t-distribution table (based on degrees of freedom) or evaluated with a p-value.
- A larger absolute value of t indicates a greater difference between the sample mean and the hypothesized population mean.
- If the calculated t-statistic falls in the critical region (critical value approach) or if the p-value is less than the significance level (α), we reject the null hypothesis.
By calculating the t-statistic accurately and interpreting its results appropriately, researchers and analysts can draw meaningful conclusions about population parameters based on sample data in a one-sample t-test scenario.
Step 7: Find Critical Value or p-Value:
Based on the calculated t-statistic and degrees of freedom, find the corresponding critical value from the t-distribution table or use statistical software to find the p-value associated with the t-statistic
Compare the calculated t-statistic to the critical value (for a critical value approach) or compare the p-value to the significance level (α).
- Make a Decision: If the calculated t-statistic falls within the critical region (critical value approach) or if the p-value is less than α, reject the null hypothesis. Otherwise, fail to reject the null hypothesis.
- Report the Results: Present the findings of the one-sample t-test, including the calculated t-statistic, degrees of freedom, and the decision regarding the null hypothesis. Provide a brief interpretation of the results in the context of the study or assignment.
Following these steps ensures a systematic approach to conducting a one-sample t-test, enabling you to confidently analyze data and draw meaningful conclusions in your statistics assignments or research endeavors.
Conclusion
Harnessing the one-sample t-test in your statistical toolkit opens doors to insightful data analysis and rigorous hypothesis testing. Armed with the knowledge imparted in this guide, you are better equipped to tackle and solve your statistics assignments with confidence. From formulating hypotheses to interpreting test results, each step outlined here aims to streamline your approach and enhance your understanding. By practicing these techniques and applying them across different datasets, you'll develop a proficiency that extends beyond the classroom, empowering you to excel in both academic pursuits and practical research endeavors. Master the one-sample t-test today to elevate your statistical prowess and conquer any assignment with clarity and precision.