In-Depth Analysis of Variance, Covariance, and Correlation in Binomial Distributions
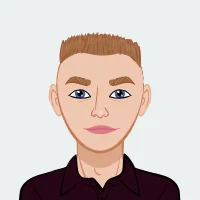
Statistics assignments often present challenges that require a deep understanding of various fundamental concepts. Key among these are variance, covariance, and correlation, especially in the context of binomial distributions. Whether you're dealing with probabilities of rolling dice or other discrete outcomes, mastering these concepts is crucial. This blog provides a structured approach to help you solve your statistics assignment efficiently. We will explore how to calculate variance, understand covariance, and determine correlation for binomially distributed random variables. By grasping these concepts, you can tackle a wide range of problems with confidence. Whether you're a student grappling with complex statistical problems or simply looking to reinforce your knowledge, this guide will provide the necessary insights to approach your assignments methodically and effectively.
Understanding Variance in Binomial Distributions
Variance measures the spread of a binomially distributed random variable's possible outcomes. It helps quantify the expected fluctuation around the mean number of successes in a given number of trials. By understanding variance, you can better interpret data variability and apply this knowledge to solve your statistics assignment with greater precision.
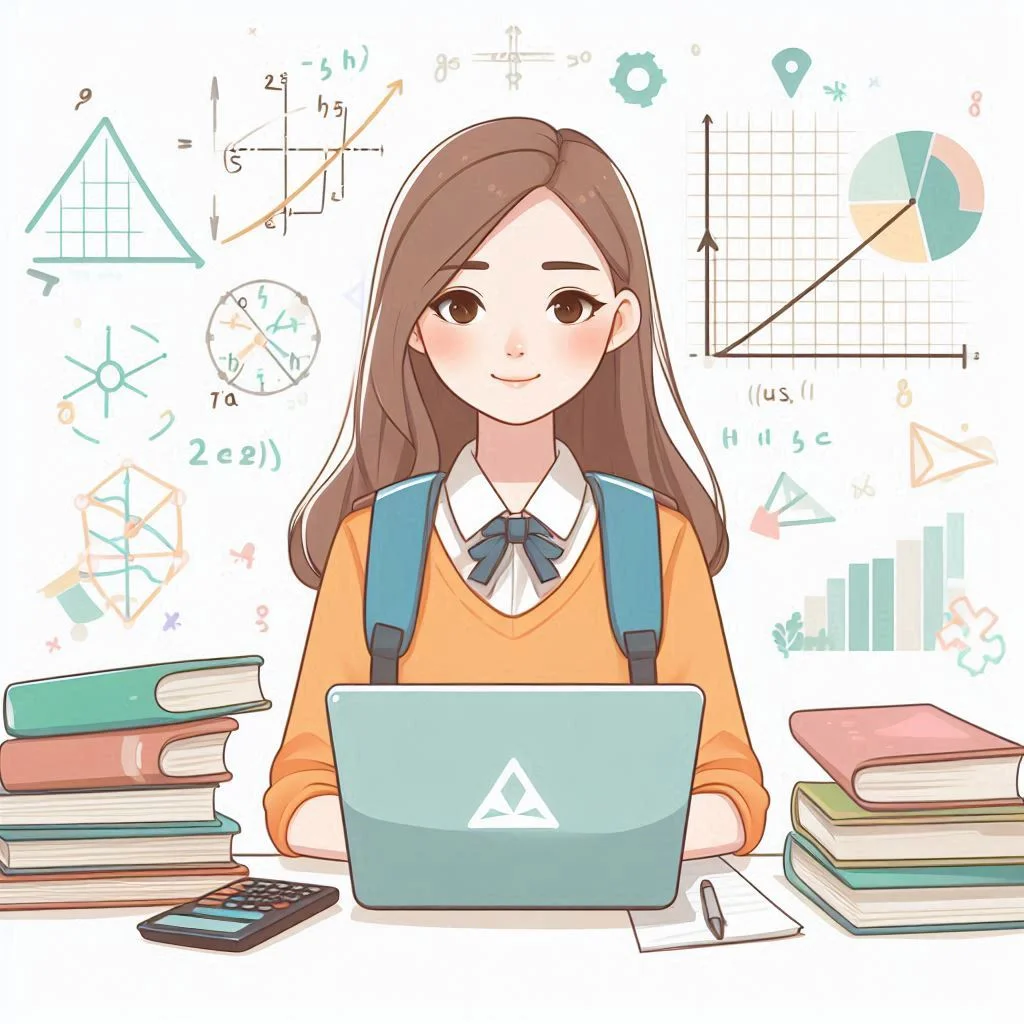
Key Concepts:
1. Binomial Distribution:
- A binomial distribution describes the number of successes in a fixed number of independent trials of a binary experiment.
- It is characterized by two parameters: n, the number of trials, and p, the probability of success in each trial.
2. Variance Formula:
- The variance of a binomially distributed random variable X is given by: Var(X)=np(1−p)
- Here, n is the number of trials and p is the probability of success. The term (1−p) represents the probability of failure.
Steps to Calculate Variance:
- Identify the Parameters: Determine the number of trials (n) and the probability of success (p) for your specific problem.
- Apply the Formula: Plug these values into the variance formula. For example, if you are rolling a fair six-sided die n times and counting the number of times you roll a 5, p would be 1/6.
- Interpret the Result: The resulting variance provides insight into the variability of the number of successes. A higher variance indicates more spread out data, while a lower variance indicates data that is more tightly clustered around the mean.
Understanding and Calculating Covariance
Covariance is a statistical measure that describes the extent to which two random variables change together. If the variables tend to show similar behavior, the covariance is positive; if they tend to show opposite behavior, the covariance is negative. Understanding and calculating covariance is essential for determining the relationship between variables and for various applications in statistics and data analysis.
Key Concepts:
1. Definition:
- Covariance between two random variables X and Y quantifies the degree to which they vary together. It is defined mathematically as: Cov(X,Y)=E[(X−E[X])(Y−E[Y])]
- Here, E[X] and E[Y] are the expected values (means) of X and Y, respectively.
2. Simplified Formula:
- The covariance can also be calculated using the formula: Cov(X,Y)=E[XY]−E[X]E[Y]
- This formula is often more convenient for practical calculations.
3. Interpretation:
- A positive covariance indicates that as X increases, Y tends to increase, and vice versa.
- A negative covariance indicates that as X increases, Y tends to decrease, and vice versa.
- A covariance close to zero suggests that X and Y are largely independent of each other.
Steps to Calculate Covariance:
1. Determine the Expected Values:
- Calculate the means E[X] and E[Y]. This involves summing all possible values of each variable weighted by their probabilities and dividing by the number of values.
2. Calculate the Expected Value of the Product:
- Compute E[XY] which involves summing the products of corresponding values of X and Y weighted by their joint probabilities.
3. Apply the Covariance Formula:
- Use the simplified formula Cov (X,Y)=E[XY]−E[X]E[Y] to find the covariance.
Understanding and Calculating Correlation
Understanding correlation is crucial for data analysis, as it helps in identifying patterns and making predictions based on data trends.
What is Correlation?
Correlation quantifies the degree to which two variables are related. It is often represented by the correlation coefficient, which ranges from -1 to 1.
- Positive Correlation: When two variables move in the same direction (e.g., as one variable increases, the other also increases), the correlation coefficient is positive. A value of +1 indicates a perfect positive correlation.
- Negative Correlation: When two variables move in opposite directions (e.g., as one variable increases, the other decreases), the correlation coefficient is negative. A value of -1 indicates a perfect negative correlation.
- No Correlation: When there is no discernible pattern in the relationship between the variables, the correlation coefficient is around 0.
Types of Correlation Coefficients
Correlation coefficients are tools used to measure and interpret the strength and direction of the relationship between two variables. Different types of correlation coefficients are suited for various types of data and relationships. Here’s a look at the most commonly used correlation coefficients:
1. Pearson Correlation Coefficient
The Pearson correlation coefficient is the most widely used measure of linear correlation between two continuous variables. It assesses how well the relationship between the variables can be described with a straight line. A Pearson coefficient close to +1 or -1 indicates a strong linear relationship, whereas a value near 0 suggests a weak or no linear relationship. This coefficient assumes that the data is normally distributed and that the relationship between the variables is linear.
2. Spearman's Rank Correlation Coefficient
Spearman's rank correlation is used to evaluate the strength and direction of the association between two ranked variables. It is particularly useful when the data does not follow a normal distribution or when dealing with ordinal data (i.e., data that can be ordered but not measured). This coefficient assesses how well the relationship between the variables can be described by a monotonic function, meaning that as one variable increases, the other variable either consistently increases or decreases, but not necessarily at a constant rate.
3. Kendall’s Tau
Kendall’s Tau measures the strength of the relationship between two variables by considering the concordance and discordance of pairs of observations. It is often used for ordinal data and provides a measure of association based on the number of pairs where both variables change in the same direction (concordant pairs) versus the number of pairs where they change in opposite directions (discordant pairs). Kendall's Tau is particularly useful in cases where there are many tied ranks or where the data is not normally distributed.
Each type of correlation coefficient offers unique insights into the relationship between variables and is selected based on the nature of the data and the specific analysis needs. Understanding the differences between these coefficients helps in choosing the most appropriate measure for your data analysis.
Steps to Calculate Correlation
1. Determine the Means:
- Begin by finding the average value for each of the two variables you are analyzing. This helps in understanding the typical value around which each variable fluctuates.
2. Calculate the Covariance:
- Assess how the two variables vary together. This involves measuring whether increases in one variable are associated with increases or decreases in the other.
3. Measure the Variability of Each Variable:
- Look at how much each variable varies on its own. This involves understanding how spread out the values of each variable are from their averages.
4. Compute the Correlation Coefficient:
- Combine the information from the covariance and the variability of each variable to find the correlation coefficient. This tells you how strongly and in what direction the two variables are related.
Practical Tips for Solving Distribution Theory Assignments
To tackle distribution theory assignments effectively, start by thoroughly understanding the problem and identifying key concepts involved. Apply the correct formulas with precision, and always double-check your work for accuracy. Use visual aids like graphs to interpret data better, and seek assistance if needed to clarify any uncertainties. Practice regularly to enhance your statistical skills.
- Read the Problem Carefully: Thoroughly reading the problem is crucial for successfully solving statistics assignments. Begin by identifying the key variables and the type of data presented. Understand what is being asked—whether you need to calculate probabilities, analyze distributions, or interpret results. Take note of any specific instructions or constraints provided in the problem statement. Break down complex problems into smaller, manageable parts to ensure you address each component accurately. Highlight important information and question any ambiguities before proceeding. By carefully reading and comprehending the problem, you can avoid common pitfalls and ensure that your approach is both accurate and relevant. This foundational step sets the stage for a methodical and effective solution.
- Identify the Relevant Concepts: Identifying the relevant concepts is crucial for solving statistics assignments efficiently. Start by carefully reading the problem to determine which statistical methods are applicable. For instance, if the assignment involves analyzing data variability, focus on concepts like variance and standard deviation. If the task requires examining relationships between variables, correlation and covariance are key. Understanding the specific context of the problem will help you pinpoint the correct concepts. For example, a problem involving probabilities might require knowledge of binomial distributions, while one involving multiple variables might need concepts related to regression analysis. Familiarize yourself with the theoretical foundations of these concepts to ensure you apply them correctly. Additionally, consider the type of data you are working with—discrete or continuous—as this will influence the choice of statistical tools. By accurately identifying and applying the relevant concepts, you can approach your assignment with greater confidence and precision.
- Apply the Formulas Correctly: Applying formulas correctly is essential for accurate results in statistics assignments. Begin by carefully identifying which formulas are relevant to the problem at hand, whether they involve variance, covariance, correlation, or other statistical measures. Ensure you understand each component of the formula and its purpose. Double-check that you are using the right values for each variable, and pay close attention to units and data types to avoid errors. When plugging values into the formula, follow each step methodically to maintain accuracy. Use a calculator or statistical software to minimize arithmetic mistakes, and verify your results by comparing them with expected outcomes or using different methods for cross-checking. Accurate application of formulas not only ensures correct results but also strengthens your understanding of statistical concepts. Consistent practice and attention to detail will improve your proficiency and confidence in solving complex statistics problems.
- Double-Check Your Work: Double-checking your work is a crucial step in solving statistics assignments to ensure accuracy and reliability. After completing your calculations, take a moment to review each step methodically. Verify that you have used the correct formulas and input the right values. Mistakes often occur in data entry or during intermediate calculations, so recalculating key results can help identify errors. Revisit your assumptions and ensure they align with the problem’s requirements. Additionally, compare your results with expected outcomes or example solutions to validate your answers. If possible, have a peer review your work or discuss it with a tutor to gain a fresh perspective. By thoroughly reviewing your work, you not only improve the accuracy of your results but also deepen your understanding of the statistical concepts involved. This careful approach ultimately enhances the quality of your assignments and builds your confidence in statistical analysis.
- Seek Help When Needed: Statistics assignments on distribution theory can be challenging, and seeking help is a smart approach when you're stuck. Don’t hesitate to reach out to tutors, classmates, or online forums for clarification. Additionally, consider using distribution theory assignment help services available online. These services offer expert assistance tailored to your specific needs, whether you're struggling with complex calculations or understanding theoretical concepts. Professional help can provide valuable insights, guide you through difficult problems, and ensure your work is accurate and well-presented. Utilizing these resources not only aids in completing your distribution theory assignments effectively but also enhances your understanding of the subject. By seeking help, you can overcome obstacles, improve your performance, and gain confidence in your statistical skills. Remember, asking for assistance is a proactive step toward mastering binomial distributions and achieving academic success.
Conclusion
In conclusion, understanding variance, covariance, and correlation within the framework of binomial distributions is essential for solving a variety of statistics assignments. By defining your variables, identifying the appropriate probability distributions, and applying the correct formulas, you can navigate through complex problems with ease. This structured approach not only simplifies the process but also enhances your analytical skills, enabling you to solve your statistics assignment more effectively. Remember, double-checking your work and seeking help when necessary are crucial steps to ensure accuracy and clarity. With these strategies, you can confidently handle any statistical problem that comes your way.